Density-Based Core Support Extraction for Non-stationary Environments with Extreme Verification Latency
2018 7th Brazilian Conference on Intelligent Systems (BRACIS)(2018)
摘要
Machine learning solutions usually consider that the train and test data has the same probabilistic distribution, that is, the data is stationary. However, in streaming scenarios, data distribution generally change through the time, that is, the data is non-stationary. The main challenge in such online environment is the model adaptation for the constant drifts in data distribution. Besides, other important restriction may happen in online scenarios: the extreme latency to verify the labels. Worth to mention that the incremental drift assumption is that class distributions overlap at subsequent time steps. Hence, the core region of data distribution have significant overlap with incoming data. Therefore, selecting samples from these core regions helps to retain the most important instances that represent the new distribution. This selection is denominated core support extraction (CSE). Thus, we present a study about density-based algorithms applied in non-stationary environments. We compared KDE, GMM and two variations of DBSCAN against single semi-supervised approaches. We validated these approaches in seventeen synthetic datasets and a real one, showing the strengths and weaknesses of these CSE methods through many metrics. We show that a semi-supervised classifier is improved up to 68% on a real dataset when it is applied along with a density-based CSE algorithm. The results between KDE and GMM, as CSE methods, were close but the approach using KDE is more practical due to having less parameters.
更多查看译文
关键词
Non-stationary environments,concept-drift,adaptive learning,extreme verification latency,density-based core support extraction
AI 理解论文
溯源树
样例
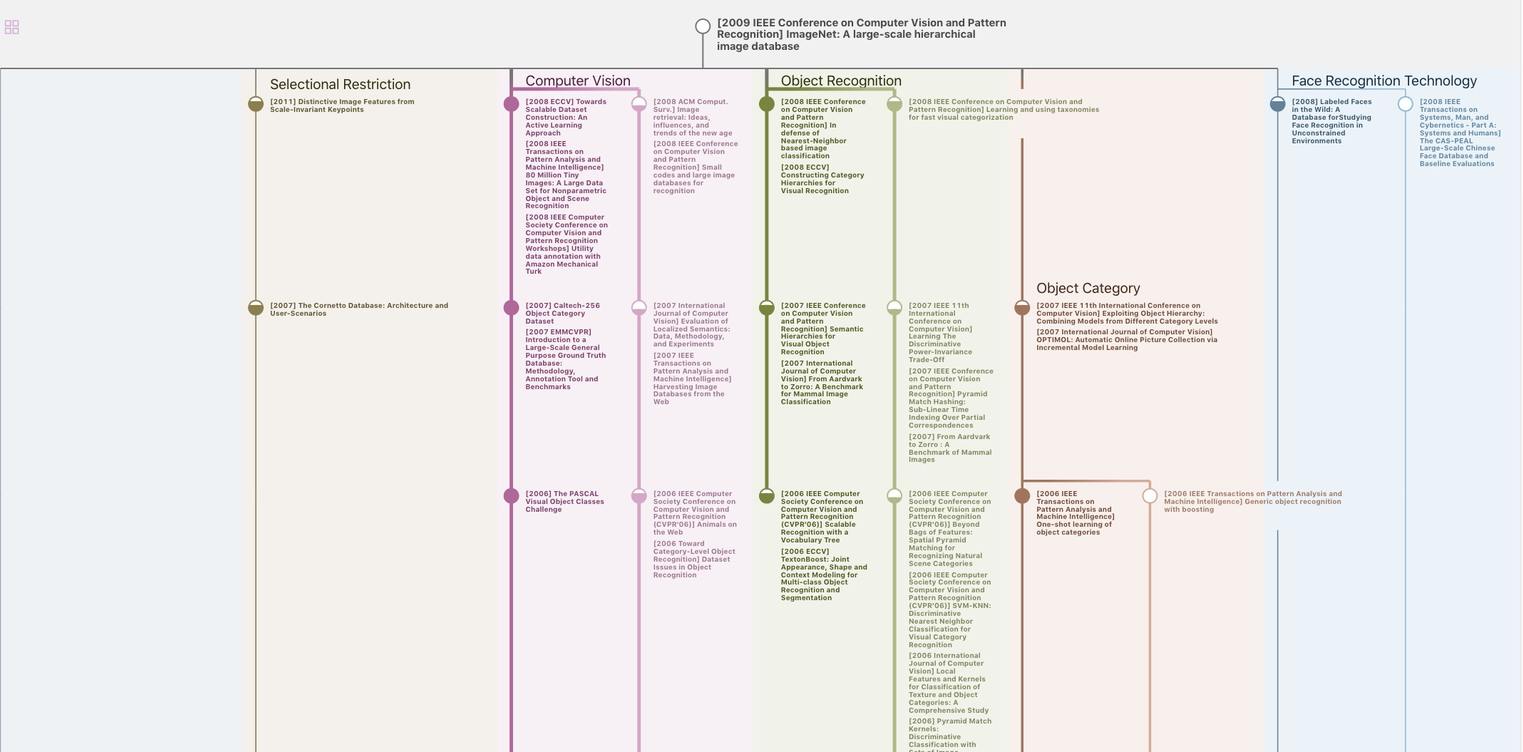
生成溯源树,研究论文发展脉络
Chat Paper
正在生成论文摘要