Making Data Stream Classification Tree-Based Ensembles Lighter
2018 7th Brazilian Conference on Intelligent Systems (BRACIS)(2018)
摘要
Recently, several classification algorithms capable of dealing with potentially infinite data streams have been proposed. One of the main challenges of this task is to continuously update predictive models to address concept drifts without compromise their predictive performance. Moreover, the classification algorithm used must be able to efficiently deal with processing time and memory limitations. In the data stream mining literature, ensemble-based classification algorithms are a good alternative to satisfy the previous requirements. These algorithms combine multiple weak learner algorithms, e.g., the Very Fast Decision Tree (VFDT), to create a model with higher predictive performance. However, the memory costs of each weak learner are stacked in an ensemble, compromising the limited space requirements. To manage the trade-off between accuracy, memory space, and processing time, this paper proposes to use the Strict VFDT (SVFDT) algorithm as an alternative weak learner for ensemble solutions which is capable of reducing memory consumption without harming the predictive performance. This paper experimentally compares two traditional and three state-of-the-art ensembles using as weak learners the VFDT and SVFDT across thirteen benchmark datasets. According to the experimental results, the proposed algorithm can obtain a similar predictive performance with a significant economy of memory space.
更多查看译文
关键词
Machine Learning, Data Streams, Ensembles, Light Weight Algorithm
AI 理解论文
溯源树
样例
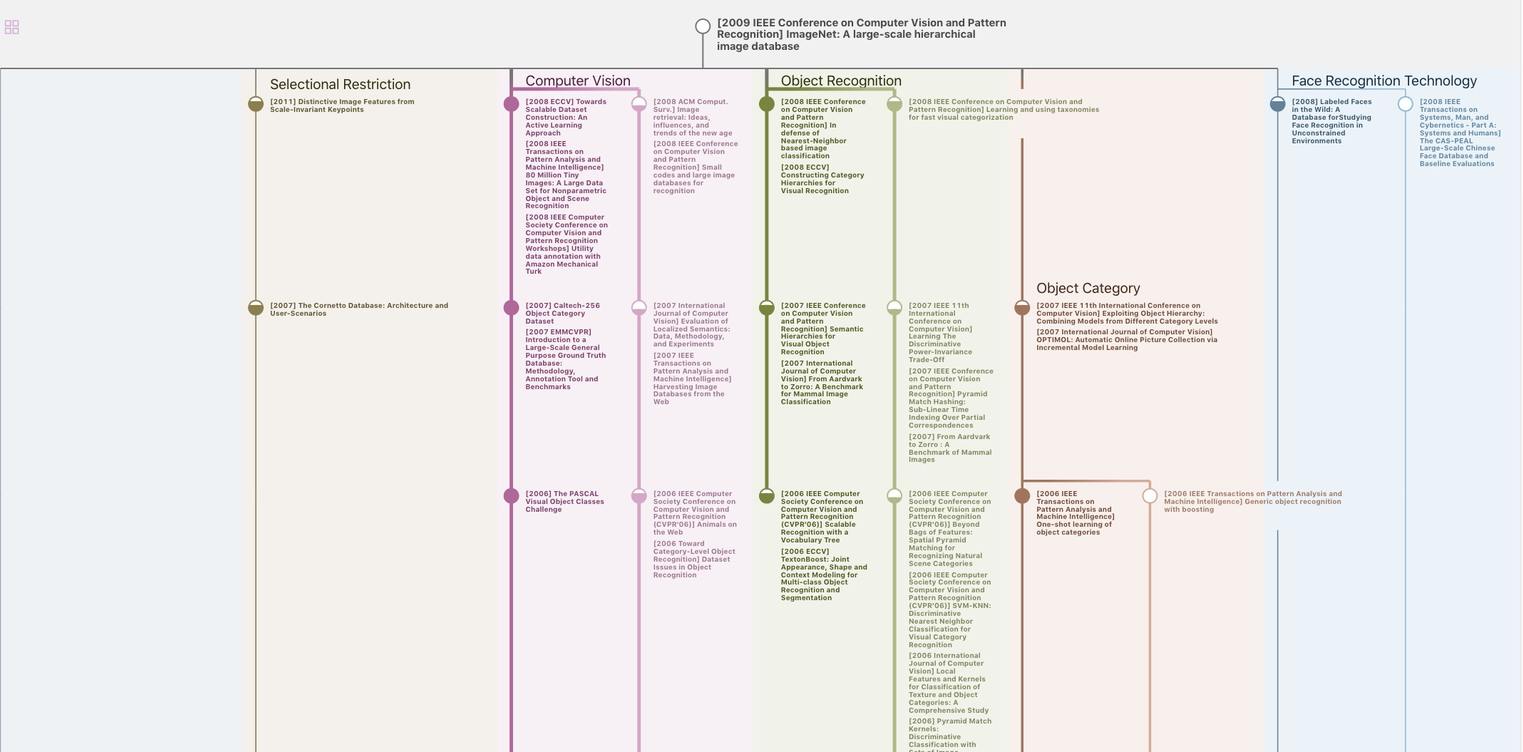
生成溯源树,研究论文发展脉络
Chat Paper
正在生成论文摘要