Designing a system to extract and interpret timed causal sentences in medical reports.
JOURNAL OF EXPERIMENTAL & THEORETICAL ARTIFICIAL INTELLIGENCE(2019)
摘要
Causal sentences are a main part of the medical explanations, providing the causes of diseases or showing the effects of medical treatments. In medicine, causal association is frequently related to time restrictions. So, some drugs must be taken before or after meals, being 'after' and 'before' temporary constraints. Thus, we conjecture that medical papers include a lot of time causal sentences. Causality involves a transfer of qualities from the cause to the effect, denoted by a directed arrow. An arrow connecting the node cause with the node effect is a causal graph. Causal graphs are an imagery way to show the causal dependencies that a sentence shows using plain text. In this article, we provide several programs to extract time causal sentences from medical Internet resources and to convert the obtained sentences in their equivalent causal graphs, providing an enlightening image of the relations that a text describes, showing the cause-effect links and the temporary constraints affecting their interpretation.
更多查看译文
关键词
Causality,time,mining causal sentences,causal graphs,time constrained causal graphs
AI 理解论文
溯源树
样例
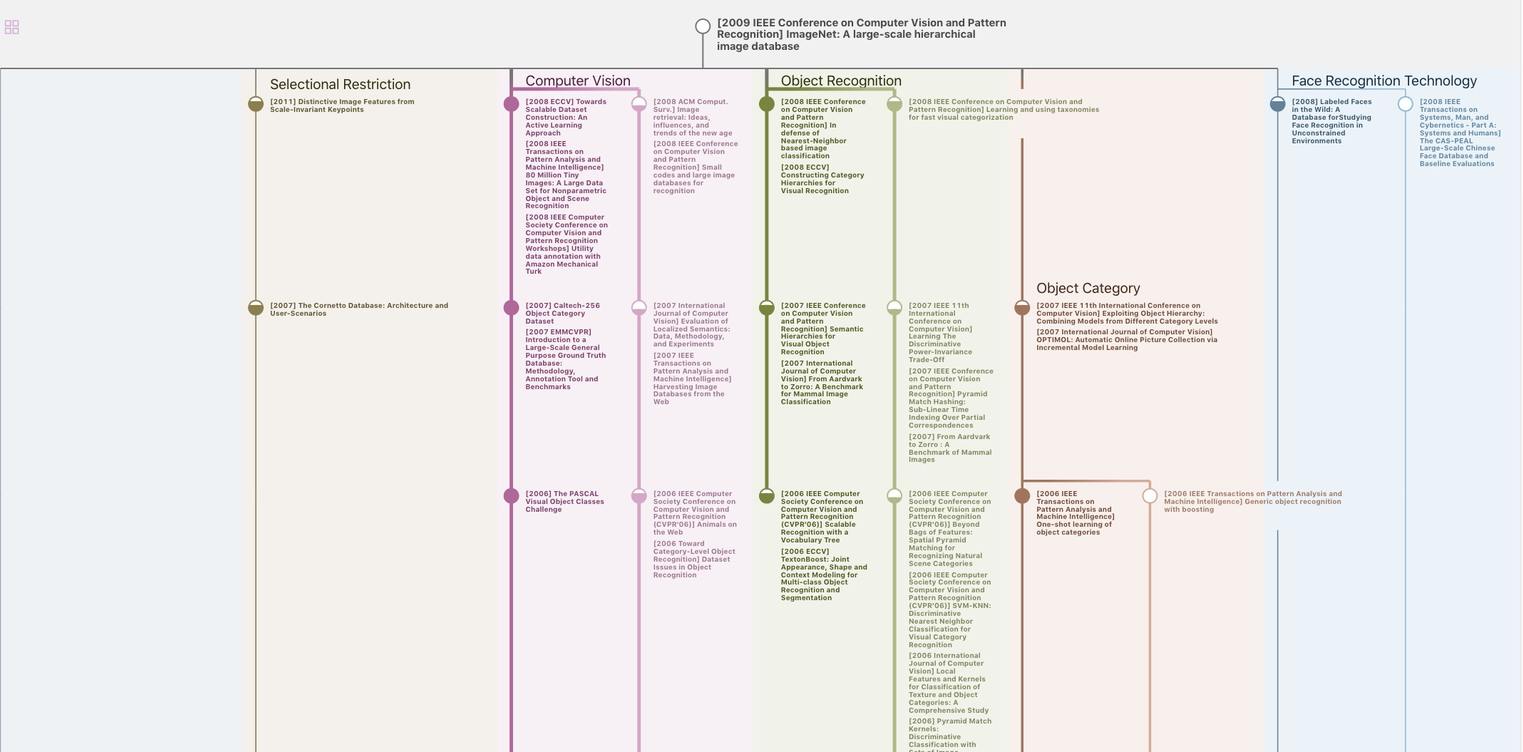
生成溯源树,研究论文发展脉络
Chat Paper
正在生成论文摘要