Improvement of the LPWAN AMI backhaul’s latency thanks to reinforcement learning algorithms
EURASIP Journal on Wireless Communications and Networking(2018)
摘要
Low power wide area networks (LPWANs) have been recently deployed for long-range machine-to-machine (M2M) communications. These networks have been proposed for many applications and in particular for the communications of the advanced metering infrastructure (AMI) backhaul of the smart grid. However, they rely on simple access schemes that may suffer from important latency, which is one of the main performance indicators in smart grid communications. In this article, we apply reinforcement learning (RL) algorithms to reduce the latency of AMI communications in LPWANs. For that purpose, we first study the collision probability in an unslotted ALOHA-based LPWAN AMI backhaul which uses the LoRaWAN acknowledgement procedure. Then, we analyse the effect of collisions on the latency for different frequency access schemes. We finally show that RL algorithms can be used for the purpose of frequency selection in these networks and reduce the latency of the AMI backhaul in LPWANs. Numerical results show that non-coordinated algorithms featuring a very low complexity reduce the collision probability by 14% and the mean latency by 40%.
更多查看译文
关键词
Advanced metering infrastructure (AMI),Low power wide area networks (LPWANs),Internet of Things (IoT),LoRaWAN,ALOHA,Smart grid communications
AI 理解论文
溯源树
样例
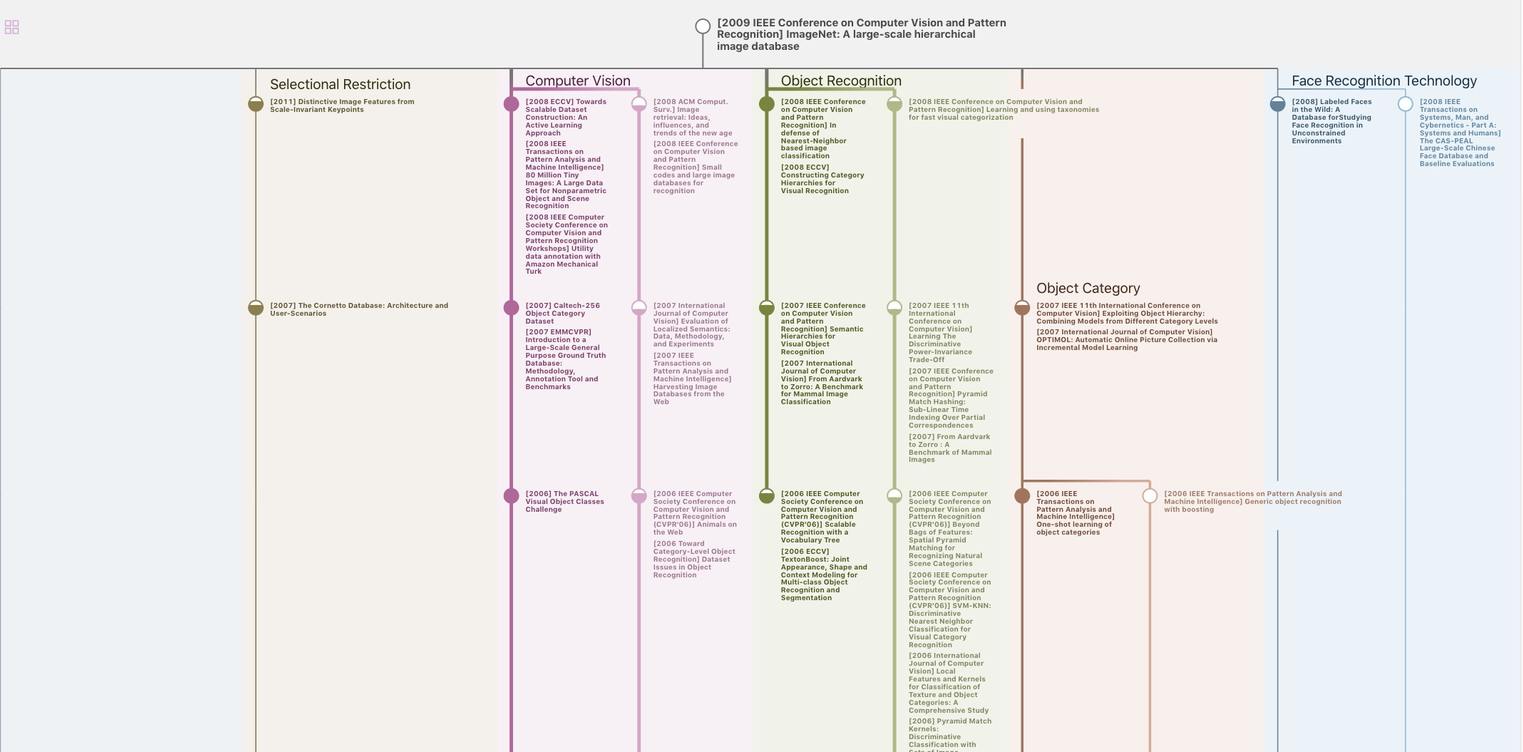
生成溯源树,研究论文发展脉络
Chat Paper
正在生成论文摘要