A Variant of AIC Based on the Bayesian Marginal Likelihood
Sankhya B(2018)
摘要
We propose information criteria that measure the prediction risk of a predictive density based on the Bayesian marginal likelihood from a frequentist point of view. We derive criteria for selecting variables in linear regression models, assuming a prior distribution of the regression coefficients. Then, we discuss the relationship between the proposed criteria and related criteria. There are three advantages of our method. First, this is a compromise between the frequentist and Bayesian standpoints because it evaluates the frequentist’s risk of the Bayesian model. Thus, it is less influenced by a prior misspecification. Second, the criteria exhibits consistency when selecting the true model. Third, when a uniform prior is assumed for the regression coefficients, the resulting criterion is equivalent to the residual information criterion (RIC) of Shi and Tsai ( J. R. Stat. Soc. Ser. B 64 , 237–252 2002 ).
更多查看译文
关键词
AIC,BIC,Consistency,Kullback–Leibler divergence,Linear regression model,Residual information criterion,Variable selection
AI 理解论文
溯源树
样例
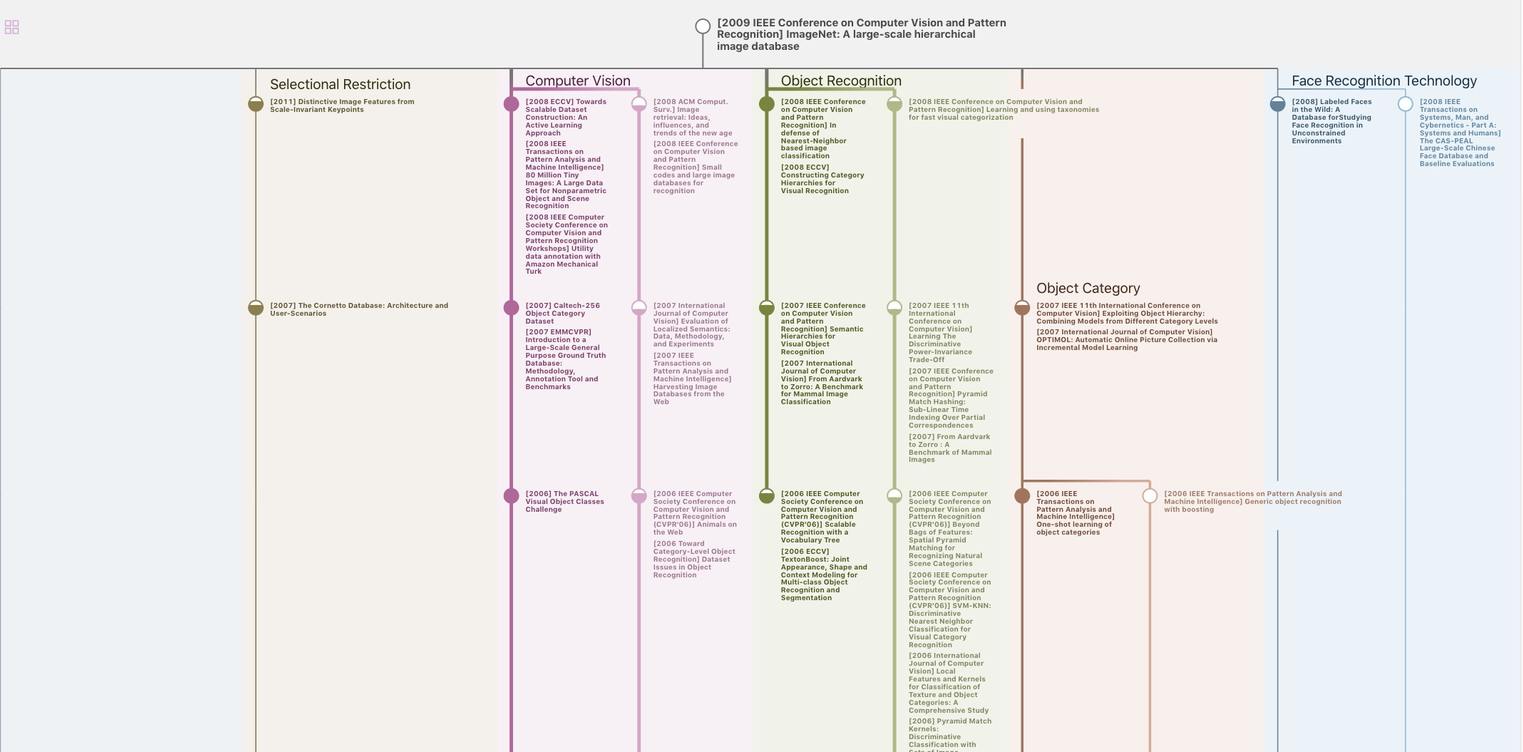
生成溯源树,研究论文发展脉络
Chat Paper
正在生成论文摘要