NavWalker: Information Augmented Network Embedding
WI(2018)
摘要
We present NavWalker, a flexible random walk-based approach for learning the representations of vertices in an information network. The proposed method enables us to incorporate different walk strategies into the sampling process of random walks, in order to further boost the network embedding techniques. Specifically, we formulate the proposed method by integrating the adjacency matrix of a network with a pre-defined information augmentation matrix. In contrast to SkipGram-based network embedding methods such as DeepWalk and Node2vec, which use only local network information to learn the representations, our method is flexible to further incorporate global or other auxiliary network information to guide the sampling process. Experiments on six real-world datasets demonstrate the advantages of the flexibility and its superior performance as compared to other state-of-the-art network embedding algorithms for the tasks of classification and recommendation.
更多查看译文
关键词
Task analysis,Sampling methods,Dimensionality reduction,Stochastic processes,Data mining,Information technology,Technological innovation
AI 理解论文
溯源树
样例
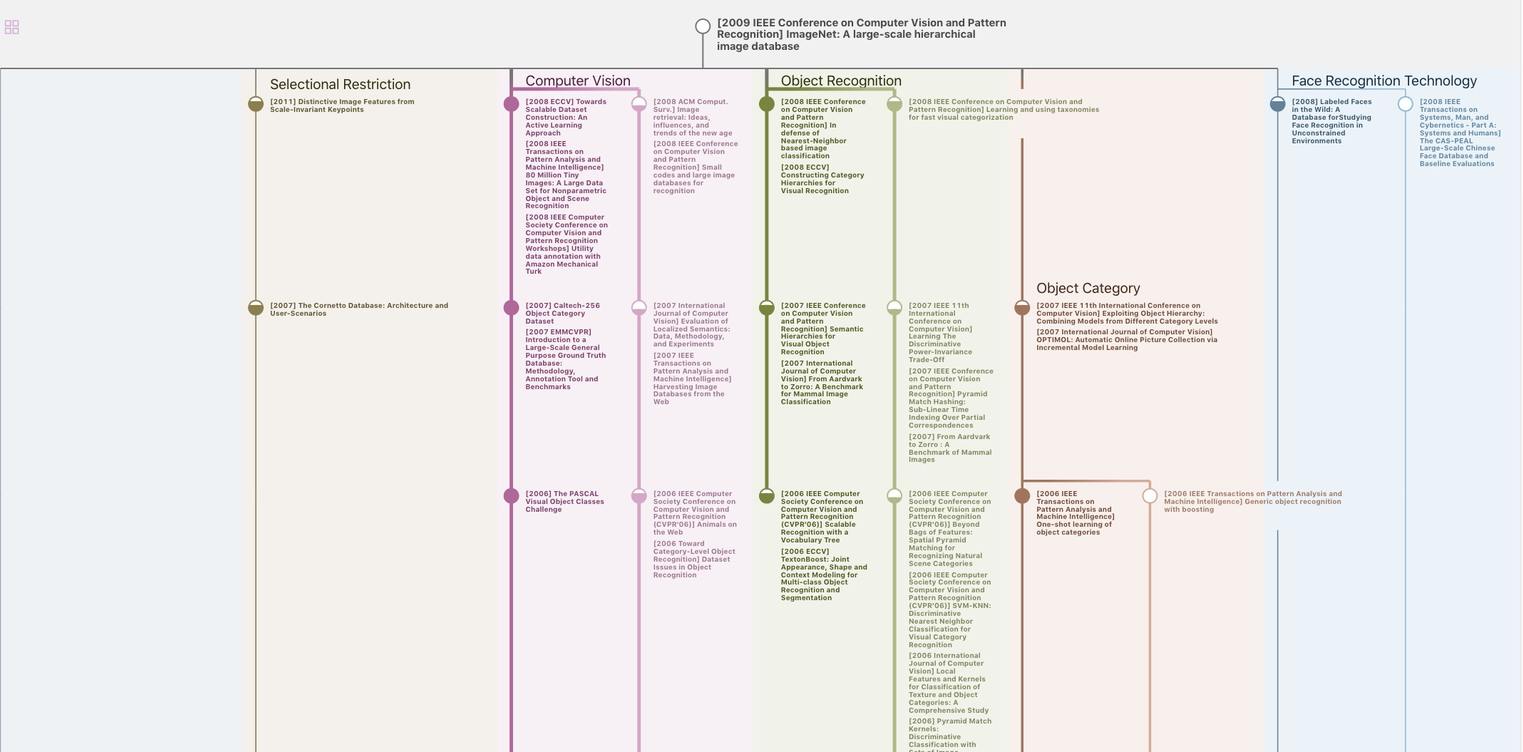
生成溯源树,研究论文发展脉络
Chat Paper
正在生成论文摘要