A Machine Learning-Based Algorithm for Joint Scheduling and Power Control in Wireless Networks
IEEE Internet of Things Journal(2018)
摘要
Wireless network resource allocation is an important issue for designing Internet of Things systems. In this paper, we consider the problem of wireless network capacity optimization that involves issues such as flow allocation, link scheduling, and power control. We show that it can be decomposed into a linear program and a nonlinear weighted sum-rate maximization problem for power allocation. Unlike most traditional methods that iteratively search the optimal solutions of the nonlinear subproblem, we propose to directly compute approximated solutions based on machine learning techniques. Specifically, the learning systems consist of both support vector machines (SVMs) and deep belief networks (DBNs) that are trained based on offline computed optimal solutions. In the running phase, the SVMs perform classification for each link to decide whether to use maximal transmit power or be turned off. At the same time, the DBNs compute an approximation of the optimal power allocation. The two results are combined to obtain an approximated solution of the nonlinear program. Simulation results demonstrate the effectiveness of the proposed machine learning-based algorithm.
更多查看译文
关键词
Machine learning,Resource management,Optimization,Power control,Wireless networks,Machine learning algorithms,Routing
AI 理解论文
溯源树
样例
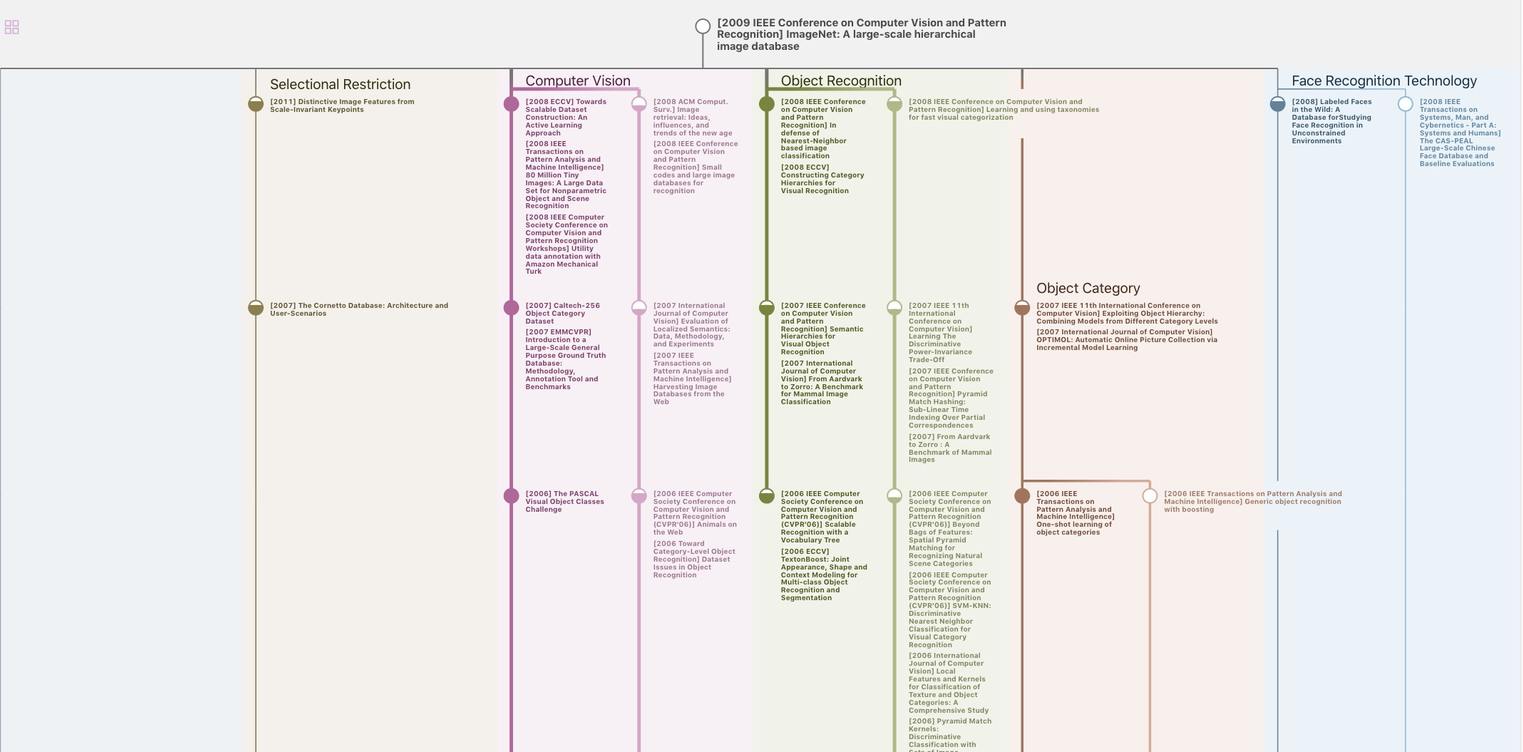
生成溯源树,研究论文发展脉络
Chat Paper
正在生成论文摘要