Robust $$l_{2,1}$$l2,1 Norm-Based Sparse Dictionary Coding Regularization of Homogenous and Heterogenous Graph Embeddings for Image Classifications
Neural Processing Letters(2018)
摘要
In the field of manifold learning, Marginal Fisher Analysis (MFA), Discriminant Neighborhood Embedding (DNE) and Double Adjacency Graph-based DNE (DAG-DNE) construct the graph embedding for homogeneous and heterogeneous k-nearest neighbors (i.e. double adjacency) before feature extraction. All of them have two shortcomings: (1) vulnerable to noise; (2) the number of feature dimensions is fixed and likely very large. Taking advantage of the sparsity effect and de-noising property of sparse dictionary, we add the \(l_{2,1}\) norm-based sparse dictionary coding regularization term to the graph embedding of double adjacency, to form an objective function, which seeks a small amount of significant dictionary atoms for feature extraction. Since our initial objective function cannot generate the closed-form solution, we construct an auxiliary function instead. Theoretically, the auxiliary function has closed-form solution w.r.t. dictionary atoms and sparse coding coefficients in each iterative step and its monotonously decreased value can pull down the initial objective function value. Extensive experiments on the synthetic dataset, the Yale face dataset, the UMIST face dataset and the terrain cover dataset demonstrate that our proposed algorithm has the ability of pushing the separability among heterogenous classes onto much fewer dimensions, and robust to noise.
更多查看译文
关键词
Graph embedding,Sparse dictionary coding,$$l_{2,1}$$l2,1 norm,Auxiliary function,Feature extraction,Sparsity effect,De-noising property
AI 理解论文
溯源树
样例
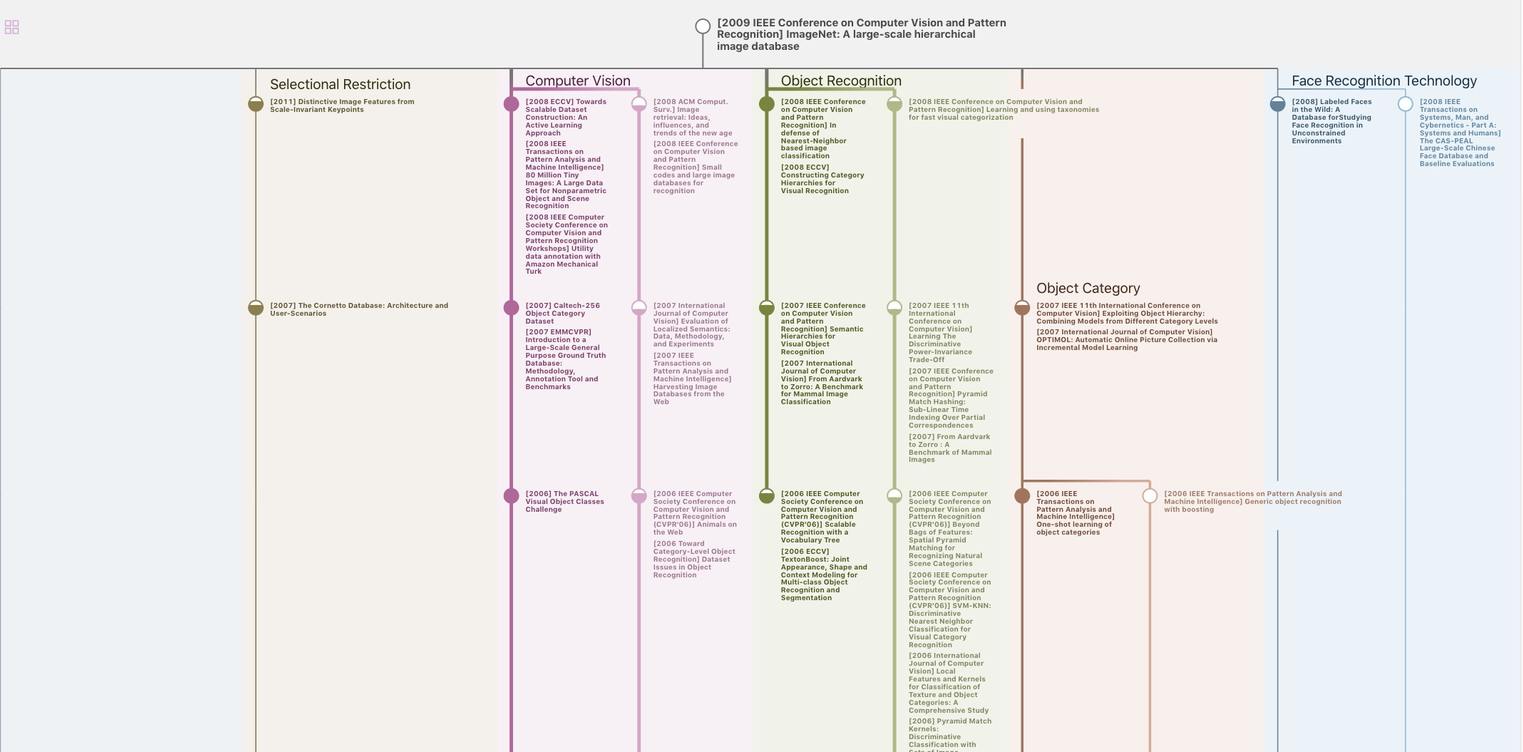
生成溯源树,研究论文发展脉络
Chat Paper
正在生成论文摘要