Clinically Comprehensible Clustering Of Change In Time Series: The C4ts Algorithm
PROCEEDINGS OF THE AUSTRALASIAN COMPUTER SCIENCE WEEK MULTICONFERENCE (ACSW 2019)(2019)
摘要
Multivariate time series provide many challenges for analysis, especially if there are additional requirements of being able to translate analytical results easily to the clinical context, and scale analyses to large numbers of records. The Clinically Comprehensible Clustering of Change in Time Series (C4TS) algorithm is a novel approach to reduce anaesthetic records from a multivariate physiological time series to a set of features, directly relatable to the clinical context, scalable to large data sets, and compatible with a wide range of statistical analyses. These features are windows of the records, located at points of change detected via a drift detector and clustered into cohesive groups. A proof of concept version of C4TS was implemented and evaluated on a retrospective set of 9,246 cardiac, thoracic and otorhinological electronic anaesthetic records from one hospital in New Zealand. C4TS captured distinct features that were interpretable by clinicians, and were amenable to statistical analysis relating them to 30-day mortality yielding plausible results that would be worth exploring with a more robust implementation of the algorithm.
更多查看译文
AI 理解论文
溯源树
样例
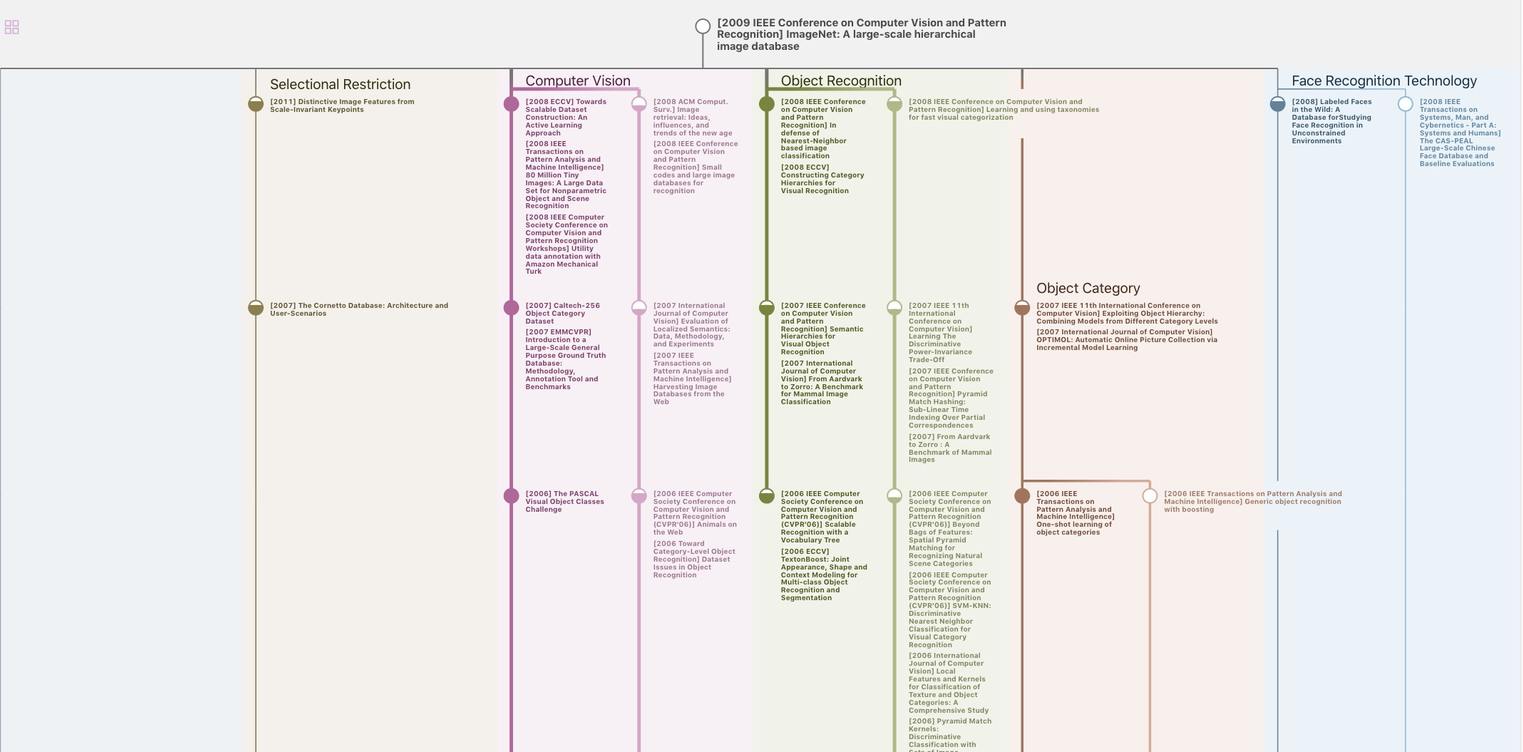
生成溯源树,研究论文发展脉络
Chat Paper
正在生成论文摘要