Distill-and-Compare: Auditing Black-Box Models Using Transparent Model Distillation
PROCEEDINGS OF THE 2018 AAAI/ACM CONFERENCE ON AI, ETHICS, AND SOCIETY (AIES'18)(2018)
摘要
Black-box risk scoring models permeate our lives, yet are typically proprietary or opaque. We propose Distill-and-Compare, a model distillation and comparison approach to audit such models. To gain insight into black-box models, we treat them as teachers, training transparent student models to mimic the risk scores assigned by black-box models. We compare the student model trained with distillation to a second un-distilled transparent model trained on ground-truth outcomes, and use differences between the two models to gain insight into the black-box model. Our approach can be applied in a realistic setting, without probing the black-box model API. We demonstrate the approach on four public data sets: COMPAS, Stop-and-Frisk, Chicago Police, and Lending Club. We also propose a statistical test to determine if a data set is missing key features used to train the black-box model. Our test finds that the ProPublica data is likely missing key feature(s) used in COMPAS.
更多查看译文
关键词
Interpretability,Black-box models,Distillation,Fairness
AI 理解论文
溯源树
样例
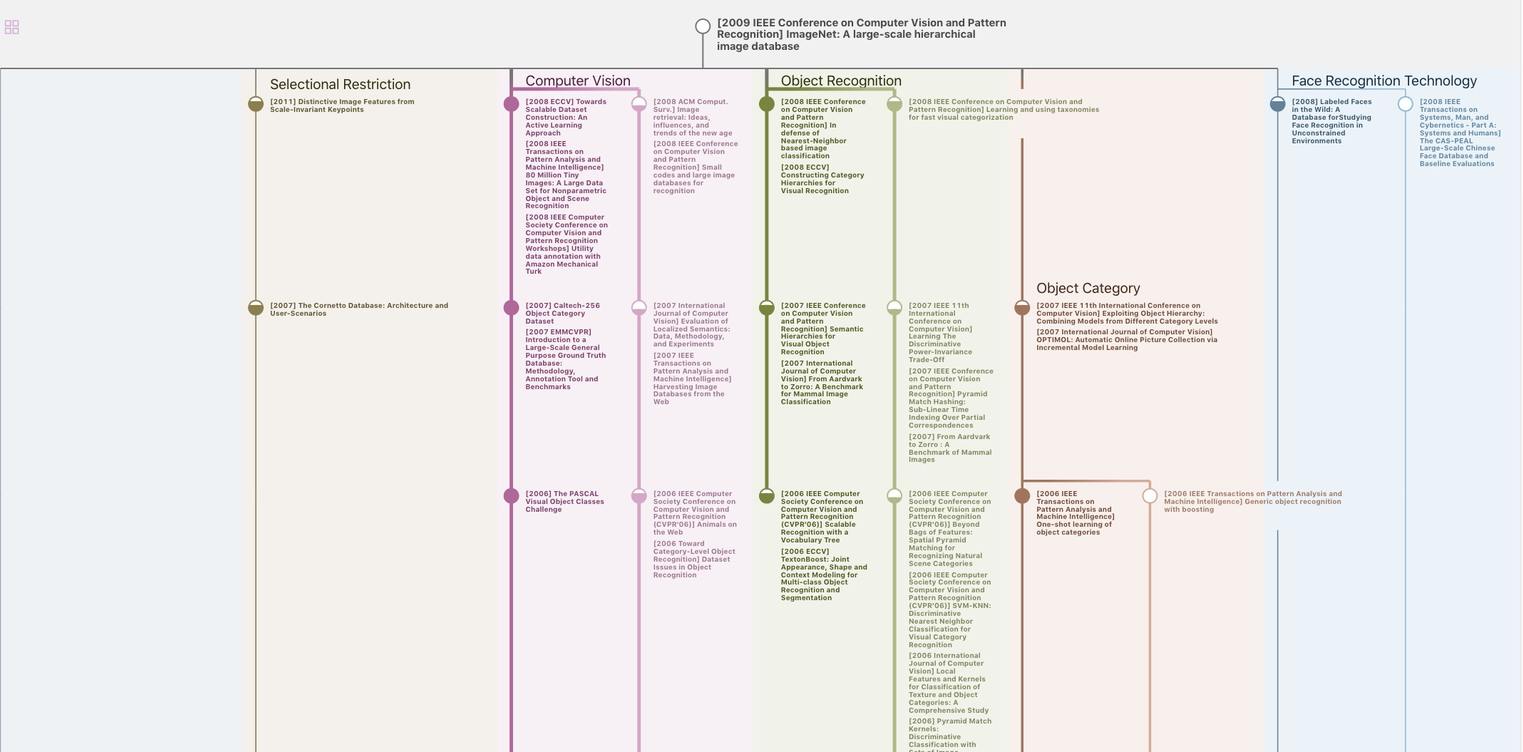
生成溯源树,研究论文发展脉络
Chat Paper
正在生成论文摘要