Adaptive Sparse Recovery with Limited Adaptivity.
SODA '19: Symposium on Discrete Algorithms San Diego California January, 2019(2019)
摘要
The goal of adaptive sparse recovery is to estimate an approximately sparse vector x from a series of linear measurements A1x, A2x, . . . , ARx, where each matrix Ai may depend on the previous observations. With an unlimited number of rounds R, it is known that O(k log log n) measurements suffice for O(1)-approximate k-sparse recovery in Rn, and that Ω(k + log log n) measurements are necessary. We initiate the study of what happens with a constant number of rounds of adaptivity. Previous techniques could not give nontrivial bounds using less than 5 rounds of adaptivity, and were inefficient for any constant R.
We give nearly matching upper and lower bounds for any constant number of rounds R. Our lower bound shows that [MATH HERE] measurements are necessary for any [MATH HERE]; significantly, this is the first lower bound that combines k and n in an adaptive setting.
Our upper bound shows that [MATH HERE] measurements suffice. The O(log* k) gap between the two bounds comes from a similar gap for nonadaptive sparse recovery in the high-SNR regime, and would be reduced to constant factors with improvements to nonadaptive high-SNR sparse recovery.
更多查看译文
AI 理解论文
溯源树
样例
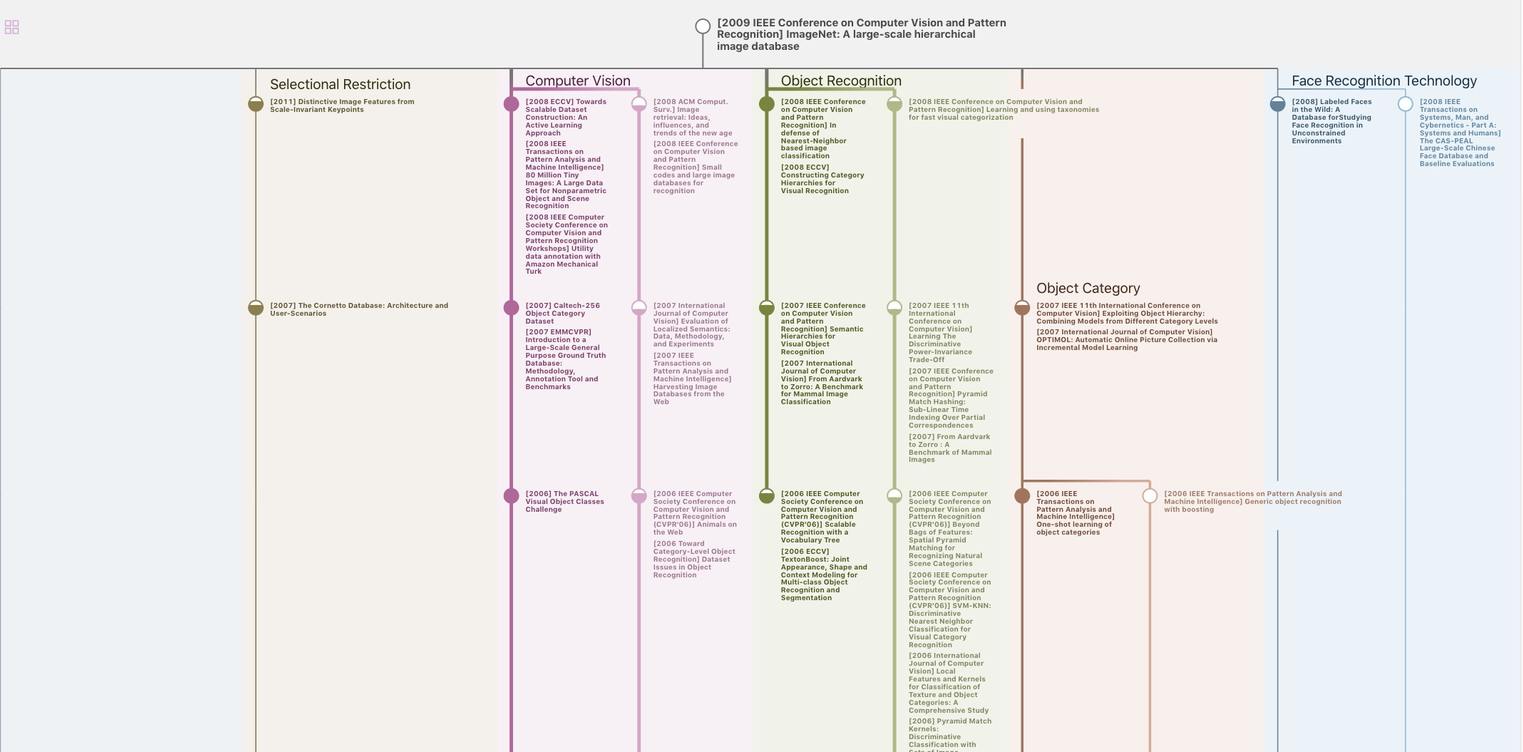
生成溯源树,研究论文发展脉络
Chat Paper
正在生成论文摘要