Deep Reinforcement Learning For Robotic Assembly Of Mixed Deformable And Rigid Objects
2018 IEEE/RSJ INTERNATIONAL CONFERENCE ON INTELLIGENT ROBOTS AND SYSTEMS (IROS)(2018)
摘要
Reinforcement learning for assembly tasks can yield powerful robot control algorithms for applications that are challenging or even impossible for "conventional" feedback control methods. Insertion of a rigid peg into a deformable hole of smaller diameter is such a task. In this contribution we solve this task with Deep Reinforcement Learning. Force-torque measurements from a robot arm wrist sensor are thereby incorporated two-fold; they are integrated into the policy learning process and they are exploited in an admittance controller that is coupled to the neural network. This enables robot learning of contact-rich assembly tasks without explicit joint torque control or passive mechanical compliance. We demonstrate our approach in experiments with an industrial robot.
更多查看译文
关键词
neural network,force torque measurements,passive mechanical compliance,deep reinforcement learning,torque control,robot control algorithms,assembly tasks,feedback control methods,robotic assembly,industrial robot,robot learning,admittance controller,policy learning process,robot arm wrist sensor,deformable hole,rigid peg
AI 理解论文
溯源树
样例
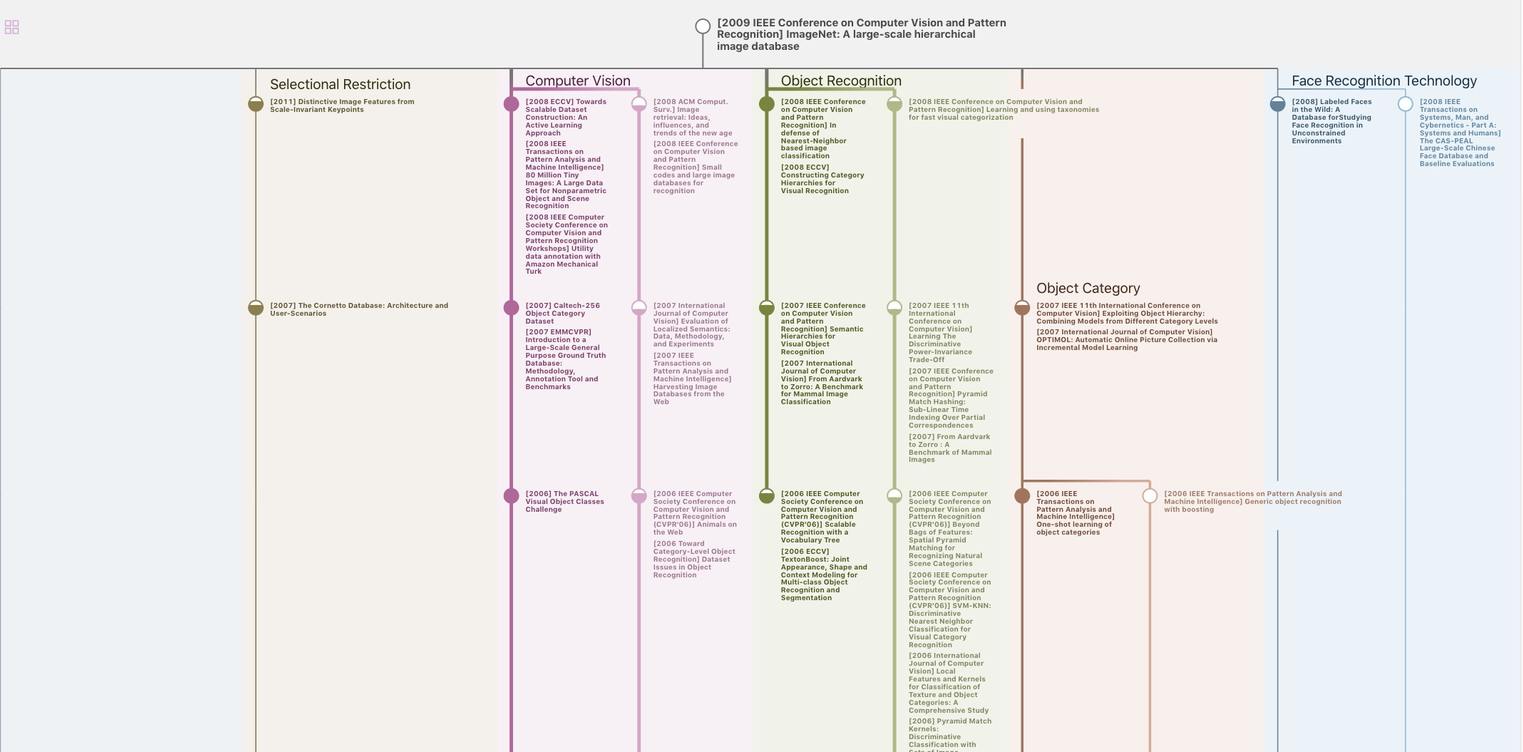
生成溯源树,研究论文发展脉络
Chat Paper
正在生成论文摘要