Pcaot: A Manhattan Point Cloud Registration Method Towards Large Rotation And Small Overlap
2018 IEEE/RSJ INTERNATIONAL CONFERENCE ON INTELLIGENT ROBOTS AND SYSTEMS (IROS)(2018)
摘要
Point cloud registration is a popular research topic and has been widely used in many tasks, such as robot mapping and localization. It is a challenging problem when the overlap is small, or the rotation is large. The problem has not been well solved by existing methods such as the iterative closest point (ICP) and its variants. In this paper, a novel method named principal coordinate alignment with overlap tuning (PCAOT) is proposed based on the Manhattan world assumption. It solves two key problems together, the transformation estimation and the overlap estimation. The overlap is represented by a 3D cuboid and the transformation is computed only within the overlap region. Instead of finding point correspondence as in traditional methods, we estimate the rotation by principal coordinates alignment, which is faster and less sensitive than ICP and its variants to small overlaps and large rotations. Evaluations demonstrate that our method achieves much better results than the ICP and its variants when the overlap ratio is smaller than 50%, or the rotation angle is larger than 60 degrees. Especially, it is effective when the overlap ratio is less than 30%, or the rotation angle is larger than 90 degrees.
更多查看译文
关键词
Manhattan world assumption,transformation estimation,overlap estimation,ICP,rotation angle,PCAOT,Manhattan point cloud registration method,robot mapping,iterative closest point,robot localization,principal coordinate alignment with overlap tuning,3D cuboid
AI 理解论文
溯源树
样例
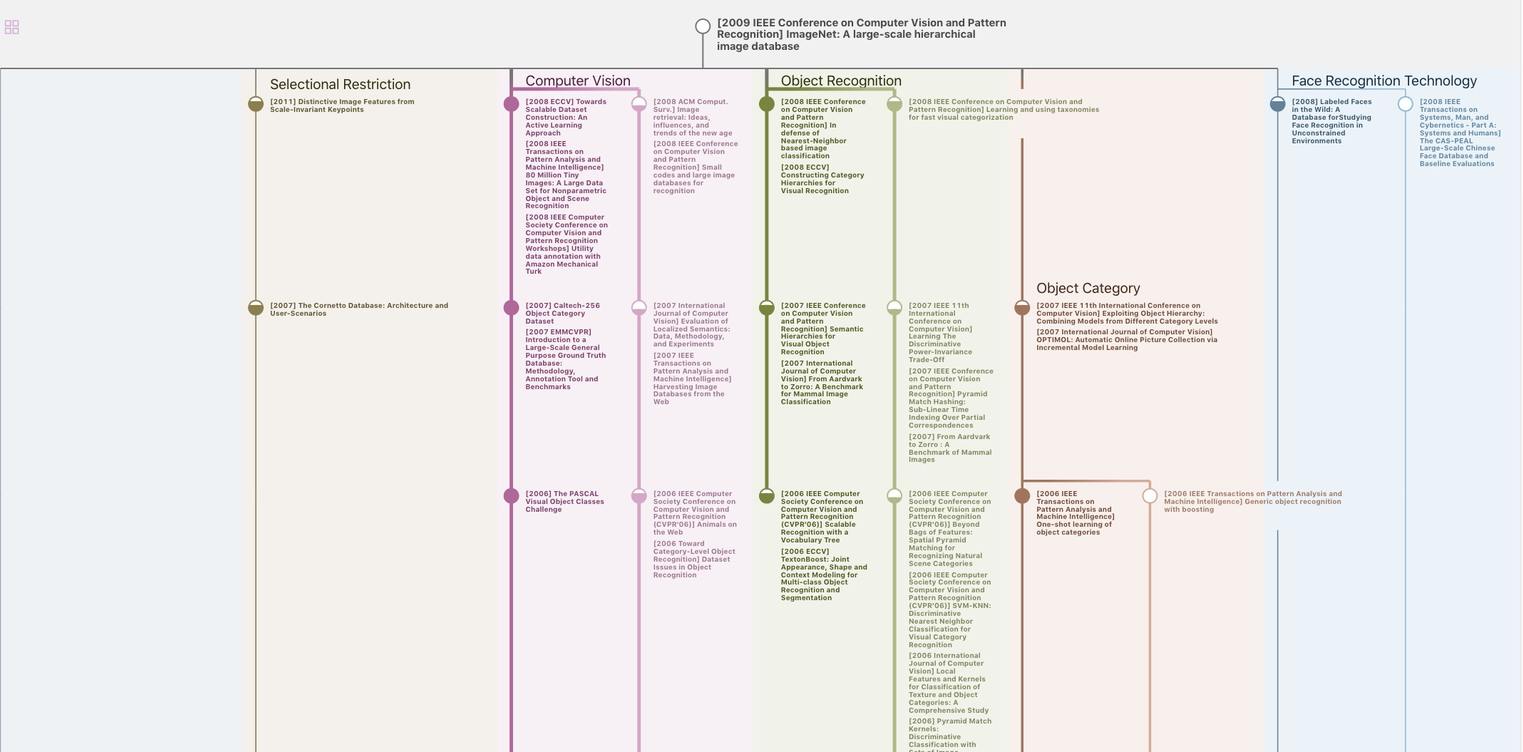
生成溯源树,研究论文发展脉络
Chat Paper
正在生成论文摘要