Depth Estimation of Optically Transparent Microrobots Using Convolutional and Recurrent Neural Networks
2018 IEEE/RSJ INTERNATIONAL CONFERENCE ON INTELLIGENT ROBOTS AND SYSTEMS (IROS)(2018)
摘要
Estimating the three-dimensional (3D) position of microrobots is necessary in order to develop closed-loop control techniques and to improve the user's 3D perception in the micro-scale. This paper describes a depth estimation method based on supervised learning for optically transparent microrobots of known geometry. The proposed methodology uses Convolutional Neural Networks (CNNs) combined with a Recurrent Network, in particular a Long Short-Term Memory (LSTM) cell for depth regression. The proposed depth regression model is independent of the 3D orientation of the microrobot and is robust to varying illumination levels while it uses learned data-specific features. The model is trained and validated using microscope images and ground truth data generated from 3D-printed microrobots imaged in an Optical Tweezers (OT) setup. The validation results demonstrate that the proposed trained model can reconstruct the depth of the microrobot independently of its 3D orientation with submicron accuracy for the test set.
更多查看译文
关键词
optically transparent microrobots,closed-loop control techniques,depth estimation method,supervised learning,depth regression model,3D-printed microrobots,recurrent neural networks,convolutional neural networks,optical tweezers setup,three-dimensional position estimation,long short-term memory cell
AI 理解论文
溯源树
样例
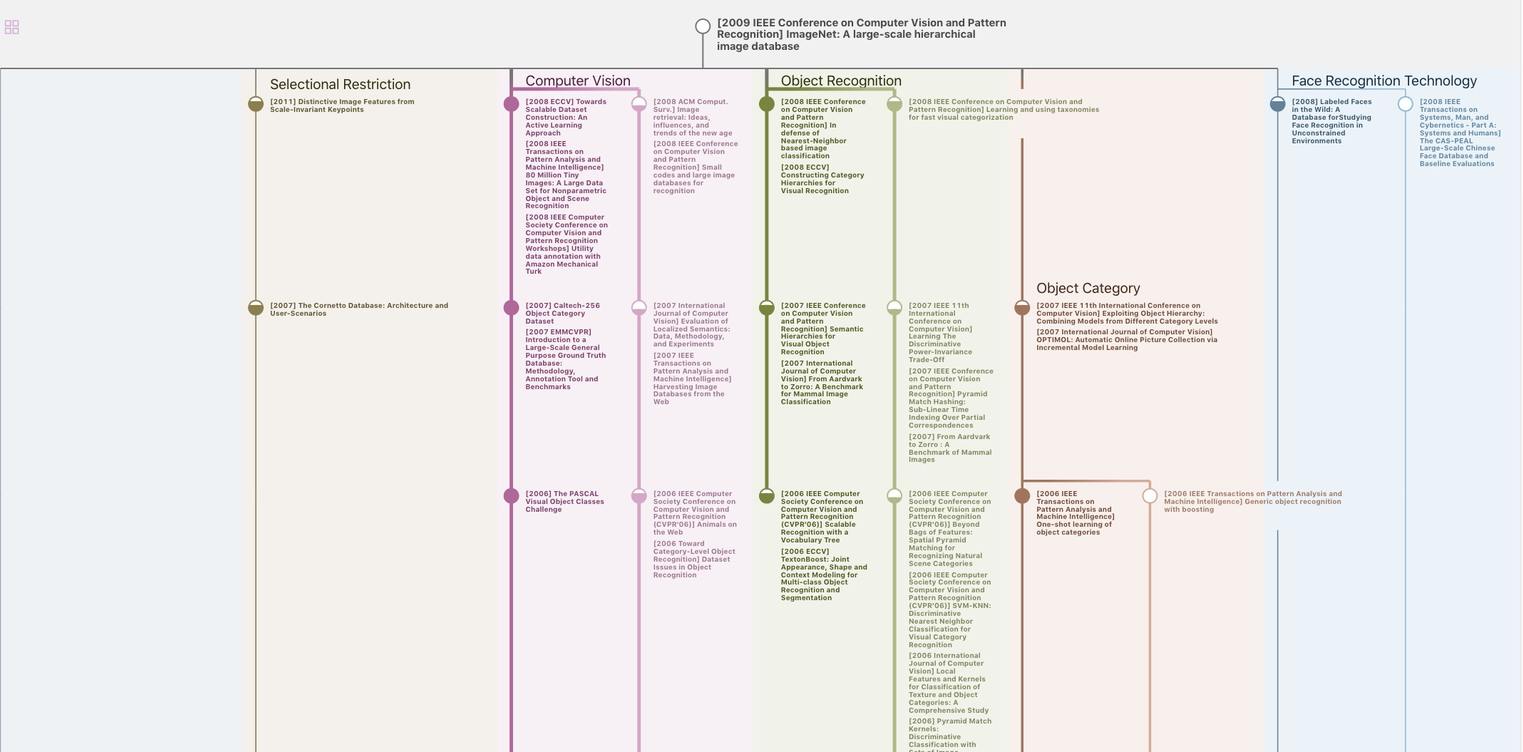
生成溯源树,研究论文发展脉络
Chat Paper
正在生成论文摘要