Constrained Path Planning Using Quadratic Programming
2018 IEEE/RSJ INTERNATIONAL CONFERENCE ON INTELLIGENT ROBOTS AND SYSTEMS (IROS)(2018)
摘要
Sampling-based planning algorithms have been extensively exploited to solve a wide variety of problems. In recent years, many efforts have been dedicated to extend these tools to solve problems involving constraints, such as geometric loop-closure, which lead the valid Configuration Space (CS) to collapse to a lower-dimensional manifold.One proposed solution considers an approximation of the constrained Configuration Space that is obtained by relaxing constraints up to a desired tolerance. The resulting set has then non-zero measure, allowing to exploit classical planning algorithms to search for a path connecting two given states. When constraints involve kinematic loops in the system, relaxation generally bears to undesired contact forces, which need to be compensated during execution by a proper control action.We propose a new tool that exploits relaxation to plan in presence of constraints. Local motions inside the approximated manifold are found as the result of an iterative scheme that uses Quadratic Optimization to proceed towards a new sample without falling outside the relaxed region. By properly guiding the exploration, paths are found with smaller relaxation factors and the need of a dedicated controller to compensate errors is reduced. We complete the analysis by showing the feasibility of the approach with experiments on a real platform.
更多查看译文
关键词
kinematic loops,local motions,iterative scheme,geometric loop-closure,CS,lower-dimensional manifold,contact forces,quadratic optimization,quadratic programming,constrained configuration space,constrained path planning,sampling-based planning algorithms
AI 理解论文
溯源树
样例
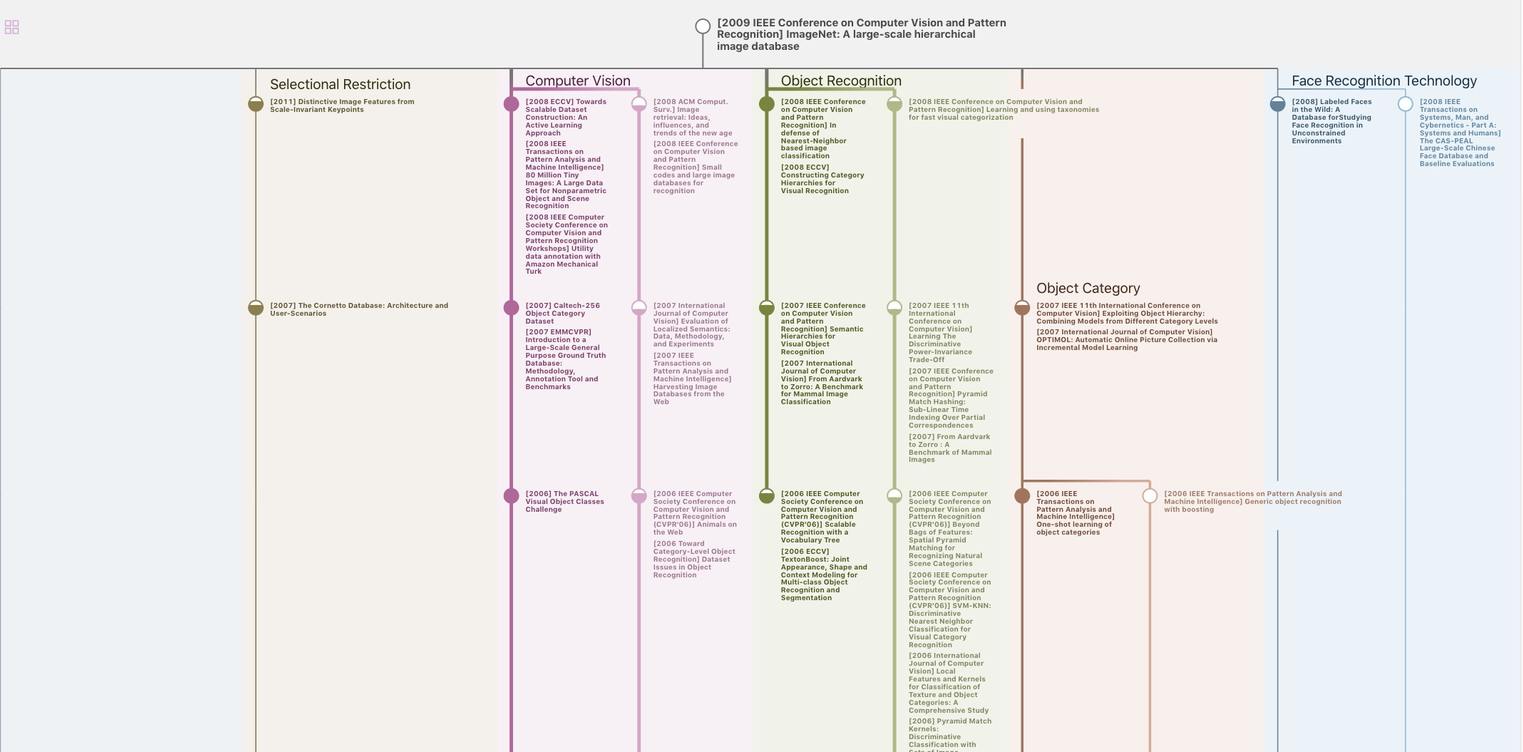
生成溯源树,研究论文发展脉络
Chat Paper
正在生成论文摘要