Smart bacteria-foraging algorithm-based customized kernel support vector regression and enhanced probabilistic neural network for compaction quality assessment and control of earth-rock dam.
EXPERT SYSTEMS(2018)
摘要
Compaction quality assessment and control for an earth-rock dam is the key measure to ensure dam safety. However, to date, the compaction quality assessment model has not been accurate enough, and no effective feedback control measures have been developed. Hybrid data mining algorithms have great potential for solving this problem. In this study, smart bacteria-foraging algorithm-based customized kernel support vector regression (SBFA-CKSVR) is proposed for compaction quality assessment, whereas an enhanced probabilistic neural network (EPNN) is adopted for compaction quality control. SBFA integrates a bacteria-foraging algorithm, chaos mapping, and adaptive and quantum computing to solve the high-dimensional complex problem effectively. CKSVR is proposed to approximate a function in quadratic continuous integral space L2(R) where its hyperparameters are optimized by SBFA. Finally, SBFA-CKSVR is used to establish a high-precision compaction quality assessment model whereas the EPNN is adopted to realize the compaction quality feedback control. A three-dimensional real-time monitoring system for the earth-rock dam is also developed based on SBFA-CKSVR and EPNN. A large-scale hydraulic engineering application proves the effectiveness and superiority of this research compared with the previous work.
更多查看译文
关键词
bacteria-foraging algorithm,compaction quality,earth-rock dam,probabilistic neural network,support vector regression
AI 理解论文
溯源树
样例
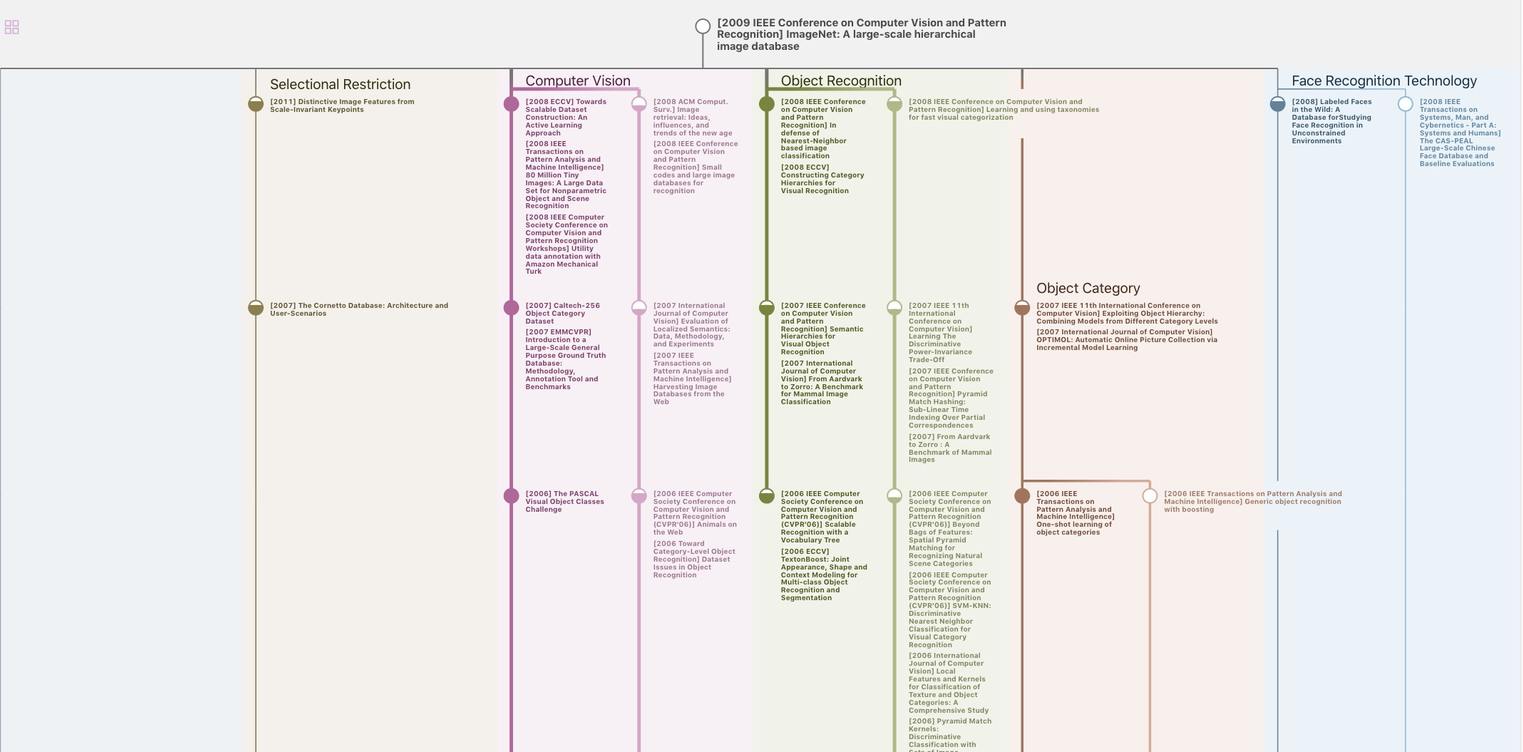
生成溯源树,研究论文发展脉络
Chat Paper
正在生成论文摘要