Quantifying Vegetation Biophysical Variables from Imaging Spectroscopy Data: A Review on Retrieval Methods
Surveys in Geophysics(2018)
摘要
An unprecedented spectroscopic data stream will soon become available with forthcoming Earth-observing satellite missions equipped with imaging spectroradiometers. This data stream will open up a vast array of opportunities to quantify a diversity of biochemical and structural vegetation properties. The processing requirements for such large data streams require reliable retrieval techniques enabling the spatiotemporally explicit quantification of biophysical variables. With the aim of preparing for this new era of Earth observation, this review summarizes the state-of-the-art retrieval methods that have been applied in experimental imaging spectroscopy studies inferring all kinds of vegetation biophysical variables. Identified retrieval methods are categorized into: (1) parametric regression, including vegetation indices, shape indices and spectral transformations; (2) nonparametric regression, including linear and nonlinear machine learning regression algorithms; (3) physically based, including inversion of radiative transfer models (RTMs) using numerical optimization and look-up table approaches; and (4) hybrid regression methods, which combine RTM simulations with machine learning regression methods. For each of these categories, an overview of widely applied methods with application to mapping vegetation properties is given. In view of processing imaging spectroscopy data, a critical aspect involves the challenge of dealing with spectral multicollinearity. The ability to provide robust estimates, retrieval uncertainties and acceptable retrieval processing speed are other important aspects in view of operational processing. Recommendations towards new-generation spectroscopy-based processing chains for operational production of biophysical variables are given.
更多查看译文
关键词
Imaging spectroscopy,Retrieval,Vegetation properties,Parametric and nonparametric regression,Machine learning,Radiative transfer models,Inversion,Uncertainties
AI 理解论文
溯源树
样例
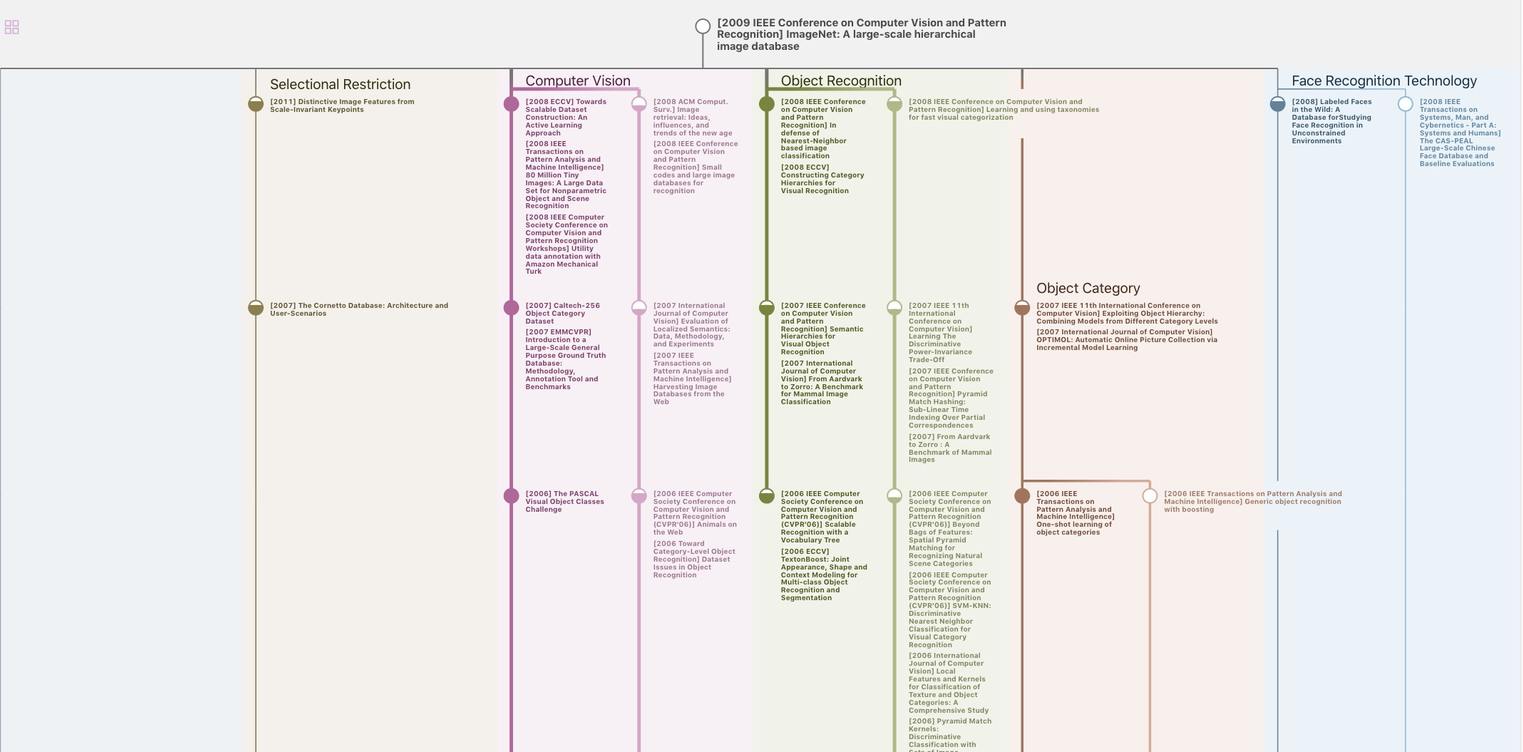
生成溯源树,研究论文发展脉络
Chat Paper
正在生成论文摘要