A context-aware convention formation framework for large-scale networks
Autonomous Agents and Multi-Agent Systems(2018)
摘要
In this article, we present a decentralized convention formation framework for creating social conventions within large multiagent convention spaces. We study the role of the topological characteristics of the network in forming conventions with an emphasis on scale-free topologies. We hypothesize that contextual knowledge encapsulated in the topology can help improve both the quality of the emergent convention and the speed of forming such a convention. We also investigate the influence of network diversity. While recent research on diversity indicates that it improves organizational productivity, we observe that not all diversity is equally useful and identify the necessary conditions to maximize the benefit of diversity. We validate our convention formation framework using a language coordination problem in which agents in a multiagent system construct a common lexicon in a decentralized fashion. Agent interactions are modeled using a language game where every agent repeatedly plays with its neighbors. Each agent stochastically updates its lexicon based on the utility values of the lexicons received from its immediate neighbors. We introduce a novel context-aware utility computation mechanism and equip the agents with the ability to reorganize their neighborhood based on this utility estimate to expedite the convention formation process. A key idea behind our approach is the ability of socially influential high-utility-lexicon agents to bias their neighbors towards accepting their lexicons. Extensive experimentation results indicate that our proposed solution is both effective (able to converge into a large majority convention state with more than 90% agents sharing a high-quality lexicon) and efficient (faster) as compared to state-of-the-art approaches for social conventions in large convention spaces.
更多查看译文
关键词
Multiagent systems,Large dynamic networks,Contextual information,Convention,Diversity,Framework
AI 理解论文
溯源树
样例
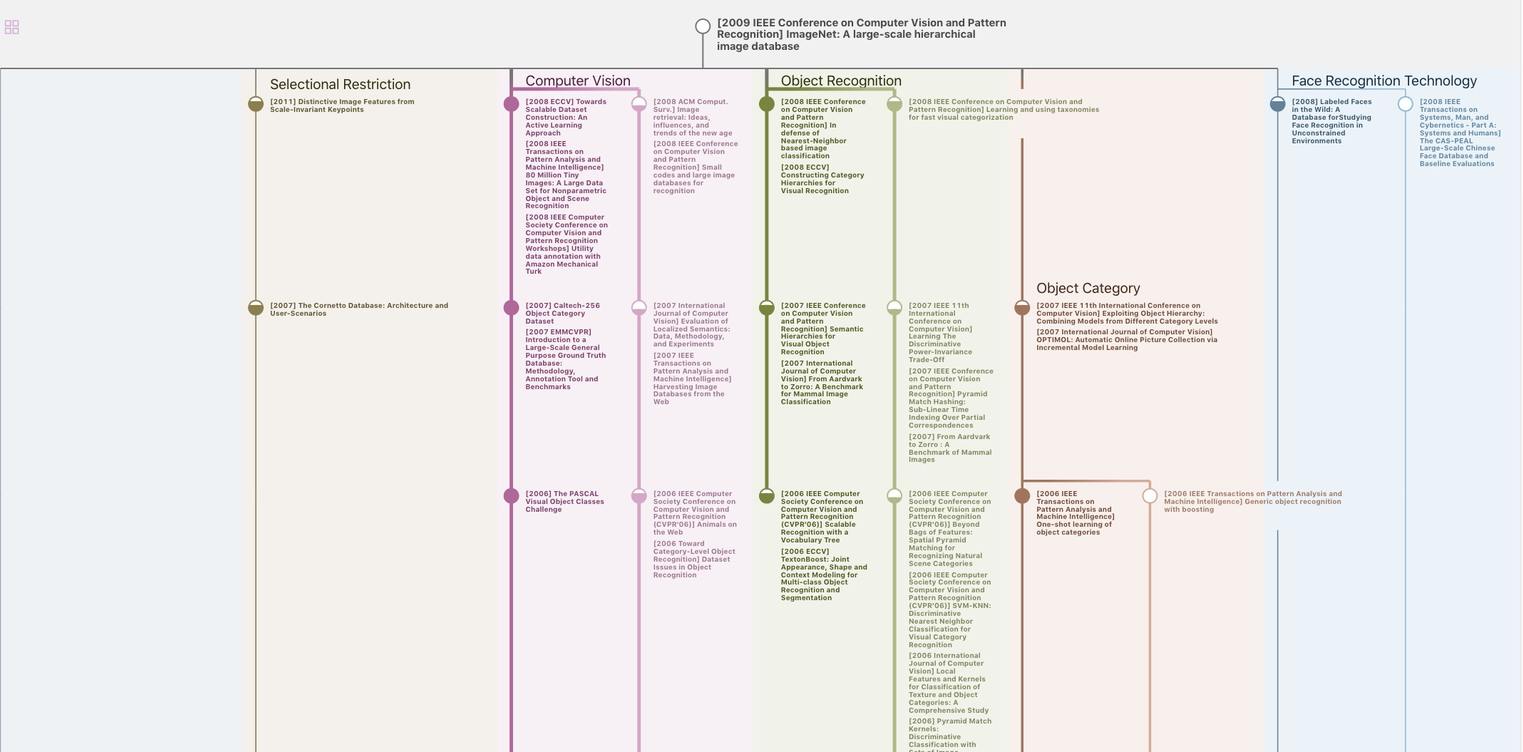
生成溯源树,研究论文发展脉络
Chat Paper
正在生成论文摘要