Privacy-Preserving SRS Data Anonymization by Incorporating Missing Values
2018 Conference on Technologies and Applications of Artificial Intelligence (TAAI)(2018)
摘要
Spontaneous Reporting Systems (SRSs) refer to systems used to collect voluntary reporting of adverse drug events (ADEs), which usually contain sensitive personal privacy information. Although many scholars have proposed various privacy protection models, they overlooked characteristics of SRS data. We previously have proposed a feasible privacy model and anonymization method dedicate to SRS data. However, this method is only applicable to complete data, not considering the fact that SRS data contain a lot of missing data. In this paper, we propose a new privacy model Closed MS(k, θ*)-bounding and a new anonymization method, Closed-MSpartition, to process SRS data with missing values. We used US FDA's FAERS data to evaluate our proposed method from the aspects of information loss, privacy risk, and data utility. The results show that our proposed new method can effectively prevent attackers from learning personal privacy without sacrificing data quality and utility.
更多查看译文
关键词
adverse drug reaction, data anonymization, privacy preserving data publishing, spontaneous reporting system, missing values
AI 理解论文
溯源树
样例
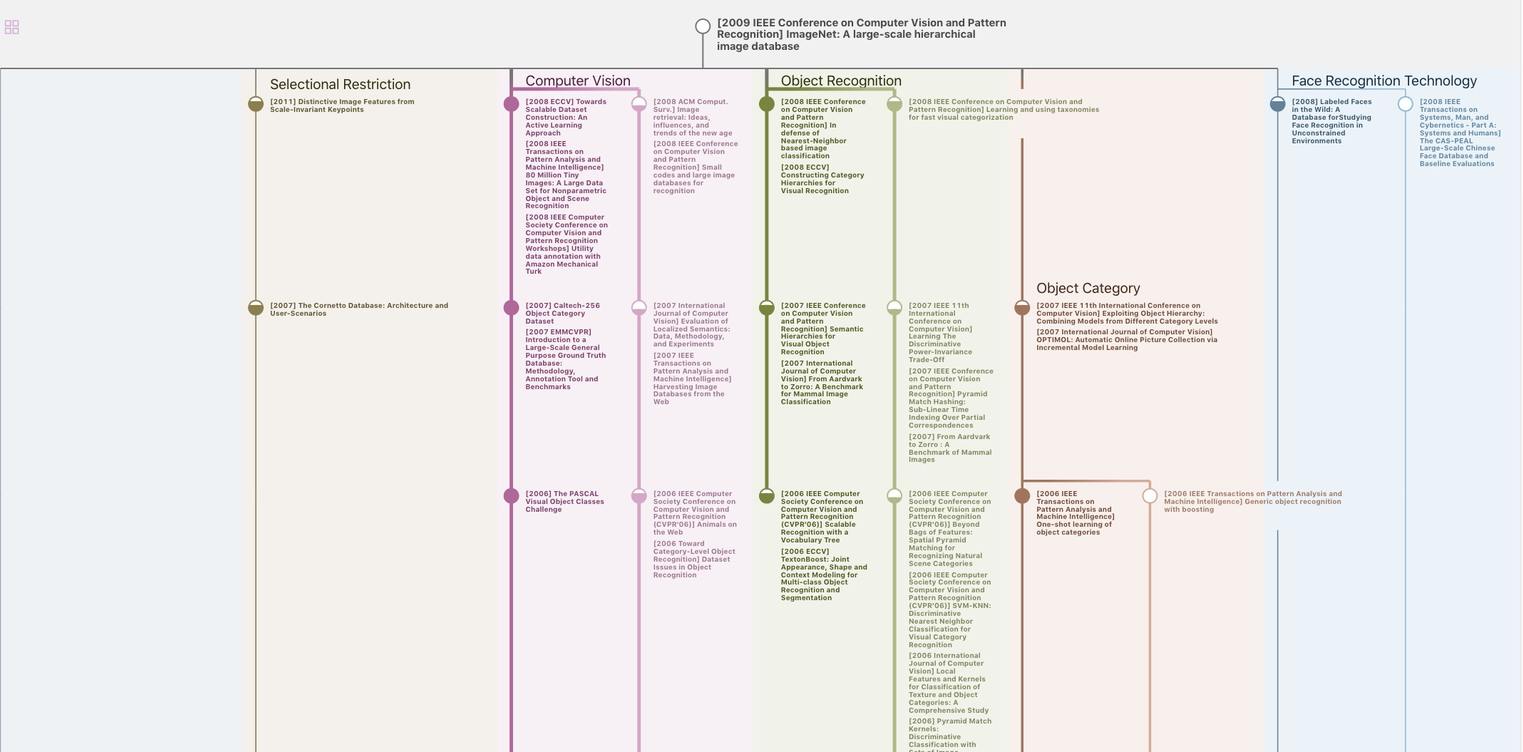
生成溯源树,研究论文发展脉络
Chat Paper
正在生成论文摘要