A Probabilistic Retrieval Model for Word Spotting Based on Direct Attribute Prediction
2018 16th International Conference on Frontiers in Handwriting Recognition (ICFHR)(2018)
摘要
In recent years CNNs took over in various fields of computer vision. Adapted to document image analysis, they achieved state-of-the-art performance in word spotting by predicting word string embeddings. One prominent embedding splits a given string in temporal pyramidal regions of character occurrences, namely the Pyramidal Histogram of Characters (PHOC). This string embedding can be interpreted as a binary attribute representation. In this work we present a new approach for ranking retrieval lists originally proposed for zero-shot learning where attribute representations play an important role. Instead of a distance-based matching of the predicted string embedding, we compute the posterior probability of the attribute representation given a word image which can be interpreted as a posterior of the query. We can show that this probabilistic ranking improves word spotting performance, especially in the query-by-string scenario.
更多查看译文
关键词
deep learning,word spotting
AI 理解论文
溯源树
样例
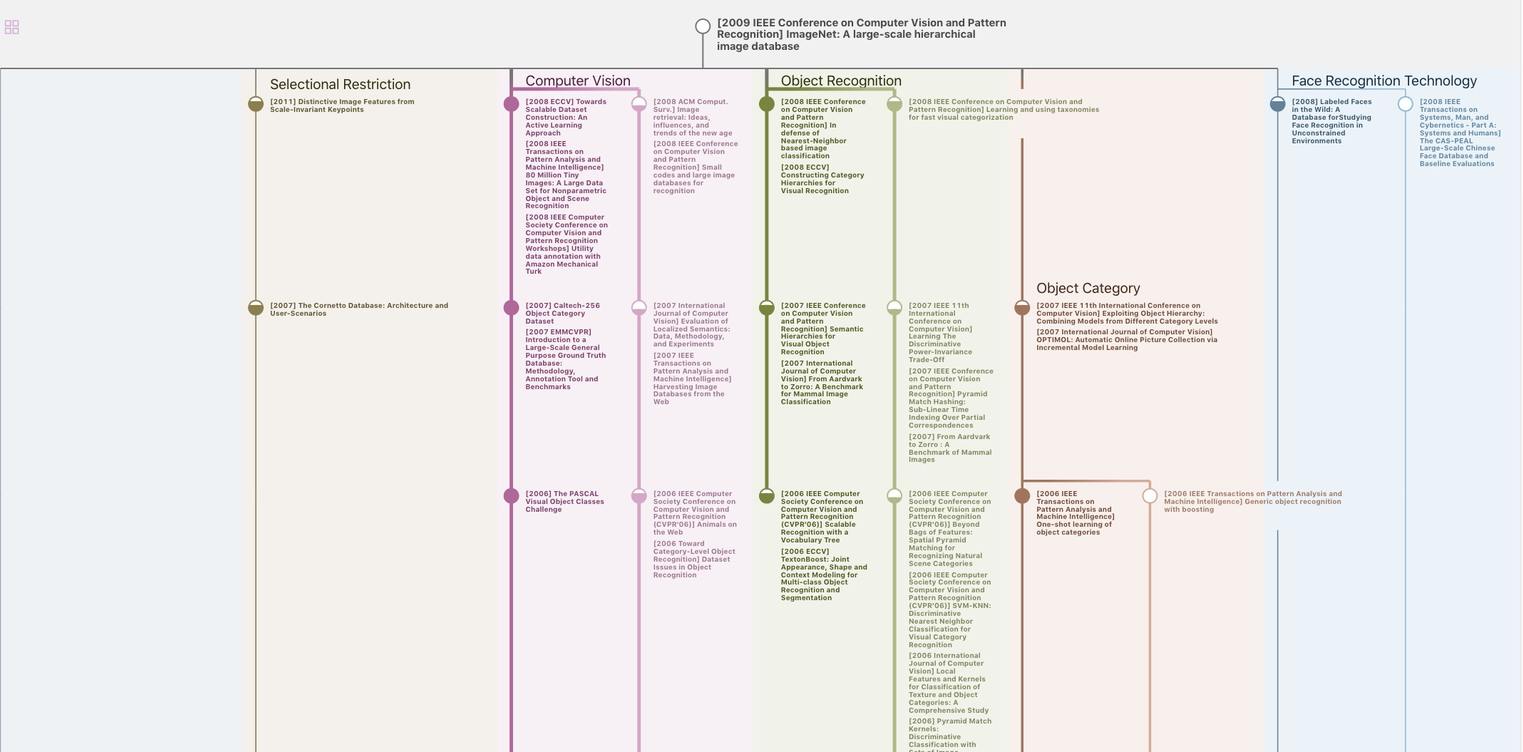
生成溯源树,研究论文发展脉络
Chat Paper
正在生成论文摘要