Lifted discriminative learning of probabilistic logic programs
Machine Learning(2018)
摘要
Probabilistic logic programming (PLP) provides a powerful tool for reasoning with uncertain relational models. However, learning probabilistic logic programs is expensive due to the high cost of inference. Among the proposals to overcome this problem, one of the most promising is lifted inference. In this paper we consider PLP models that are amenable to lifted inference and present an algorithm for performing parameter and structure learning of these models from positive and negative examples. We discuss parameter learning with EM and LBFGS and structure learning with LIFTCOVER, an algorithm similar to SLIPCOVER. The results of the comparison of LIFTCOVER with SLIPCOVER on 12 datasets show that it can achieve solutions of similar or better quality in a fraction of the time.
更多查看译文
关键词
Statistical relational learning,Probabilistic inductive logic programming,Probabilistic logic programming,Lifted inference,Expectation maximization
AI 理解论文
溯源树
样例
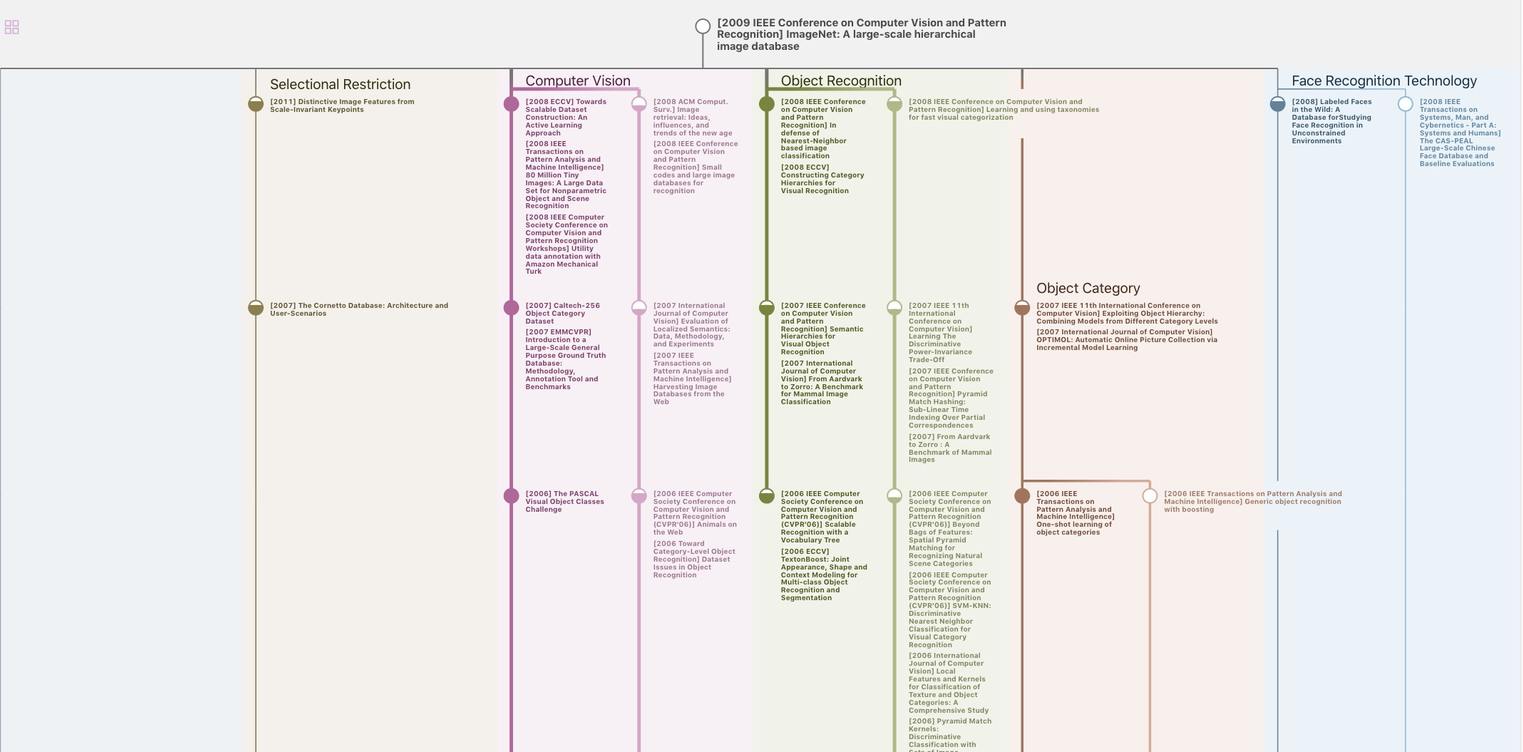
生成溯源树,研究论文发展脉络
Chat Paper
正在生成论文摘要