Bayesian optimistic Kullback–Leibler exploration
Machine Learning(2018)
摘要
We consider a Bayesian approach to model-based reinforcement learning, where the agent uses a distribution of environment models to find the action that optimally trades off exploration and exploitation. Unfortunately, it is intractable to find the Bayes-optimal solution to the problem except for restricted cases. In this paper, we present BOKLE, a simple algorithm that uses Kullback–Leibler divergence to constrain the set of plausible models for guiding the exploration. We provide a formal analysis that this algorithm is near Bayes-optimal with high probability. We also show an asymptotic relation between the solution pursued by BOKLE and a well-known algorithm called Bayesian exploration bonus. Finally, we show experimental results that clearly demonstrate the exploration efficiency of the algorithm.
更多查看译文
关键词
Model-based Bayesian reinforcement learning,Bayes-adaptive Markov decision process,PAC-BAMDP
AI 理解论文
溯源树
样例
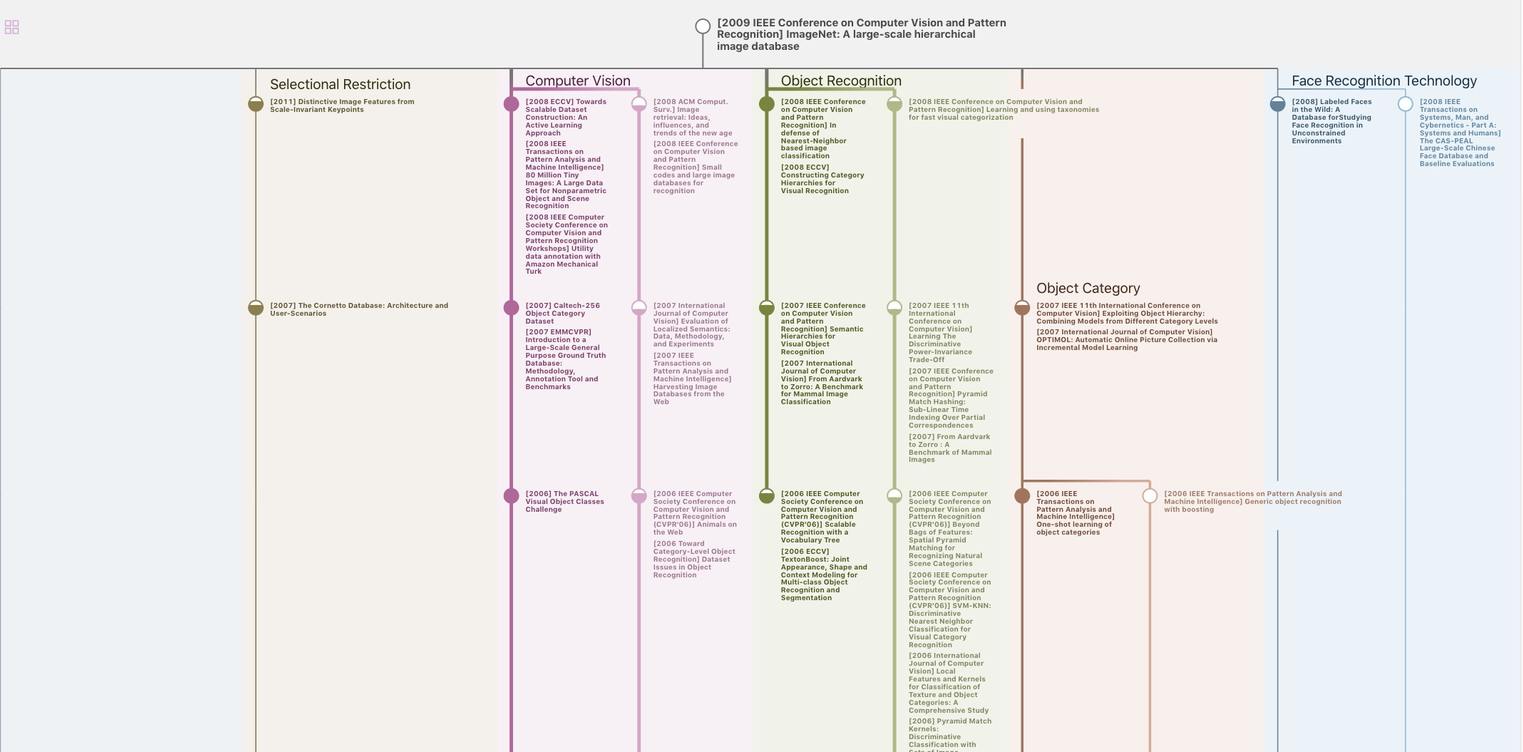
生成溯源树,研究论文发展脉络
Chat Paper
正在生成论文摘要