FCNN-based axon segmentation for convection-enhanced delivery optimization
International Journal of Computer Assisted Radiology and Surgery(2019)
摘要
Purpose Glioblastoma multiforme treatment is a challenging task in clinical oncology. Convection- enhanced delivery (CED) is showing encouraging but still suboptimal results due to drug leakages. Numerical models can predict drug distribution within the brain, but require retrieving brain physical properties, such as the axon diameter distribution (ADD), through axon architecture analysis. The goal of this work was to provide an automatic, accurate and fast method for axon segmentation in electronic microscopy images based on fully convolutional neural network (FCNN) as to allow automatic ADD computation. Methods The segmentation was performed using a residual FCNN inspired by U-Net and Resnet. The FCNN training was performed exploiting mini-batch gradient descent and the Adam optimizer. The Dice coefficient was chosen as loss function. Results The proposed segmentation method achieved results comparable with already existing methods for axon segmentation in terms of Information Theoretic Scoring ( 0.98% ) with a faster training (5 h on the deployed GPU) and without requiring heavy post-processing (testing time was 0.2 s with a non-optimized code). The ADDs computed from the segmented and ground-truth images were statistically equivalent. Conclusions The algorithm proposed in this work allowed fast and accurate axon segmentation and ADD computation, showing promising performance for brain microstructure analysis for CED delivery optimization.
更多查看译文
关键词
Axon segmentation, Electron microscopy, Deep learning, Convection-enhanced delivery, Glioblastoma
AI 理解论文
溯源树
样例
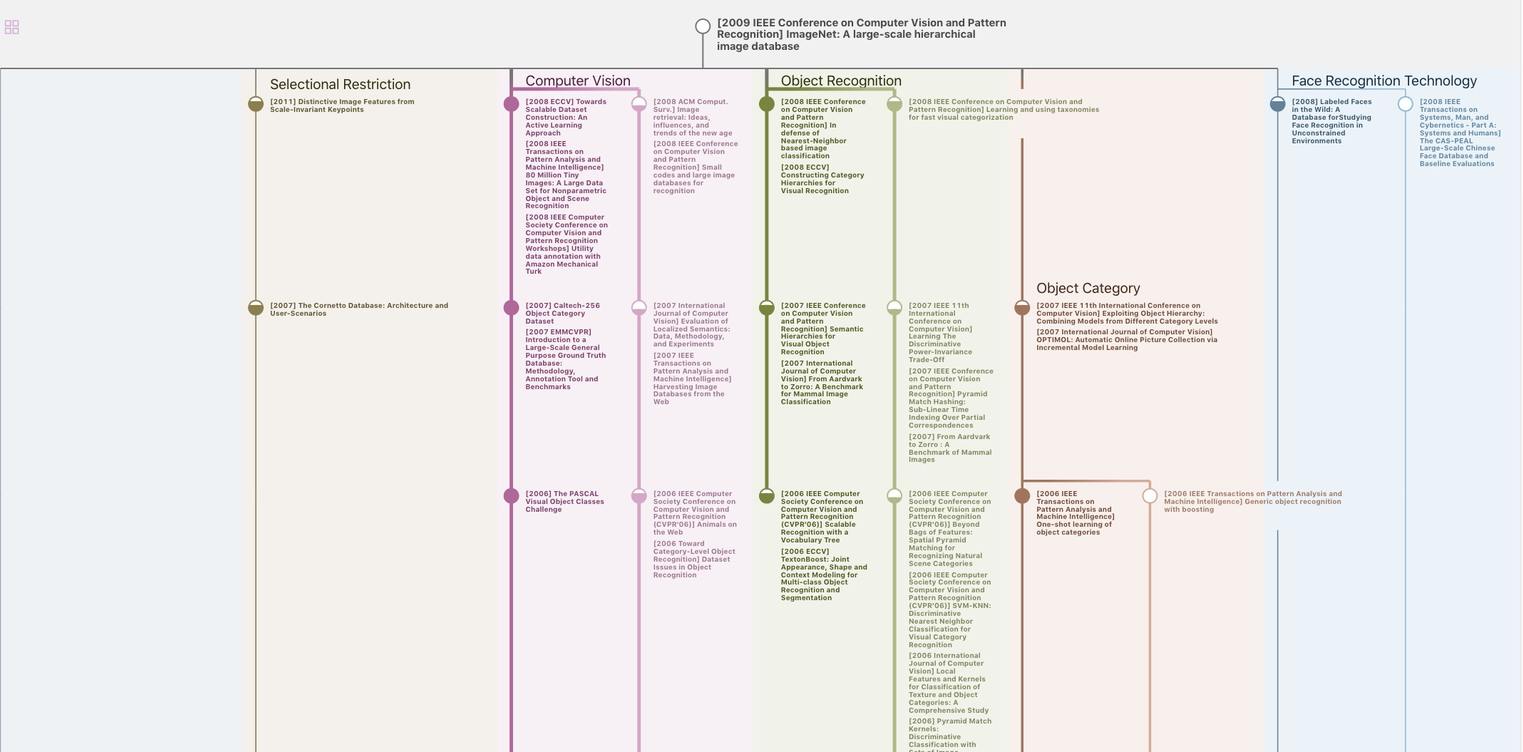
生成溯源树,研究论文发展脉络
Chat Paper
正在生成论文摘要