Affective recommender systems in online news industry: how emotions influence reading choices
User Modeling and User-Adapted Interaction(2018)
摘要
Recommender systems have become ubiquitous over the last decade, providing users with personalized search results, video streams, news excerpts, and purchasing hints. Human emotions are widely regarded as important predictors of behavior and preference. They are a crucial factor in decision making, but until recently, relatively little has been known about the effectiveness of using human emotions in personalizing real-world recommender systems. In this paper we introduce the Emotion Aware Recommender System (EARS), a large scale system for recommending news items using user’s self-assessed emotional reactions. Our original contribution includes the formulation of a multi-dimensional model of emotions for news item recommendations, introduction of affective item features that can be used to describe recommended items, construction of affective similarity measures, and validation of the EARS on a large corpus of real-world Web traffic. We collect over 13,000,000 page views from 2,700,000 unique users of two news sites and we gather over 160,000 emotional reactions to 85,000 news articles. We discover that incorporating pleasant emotions into collaborative filtering recommendations consistently outperforms all other algorithms. We also find that targeting recommendations by selected emotional reactions presents a promising direction for further research. As an additional contribution we share our experiences in designing and developing a real-world emotion-based recommendation engine, pointing to various challenges posed by the practical aspects of deploying emotion-based recommenders.
更多查看译文
关键词
Recommender systems,Affective computing,Emotion analysis,Emotion mining,News recommendations
AI 理解论文
溯源树
样例
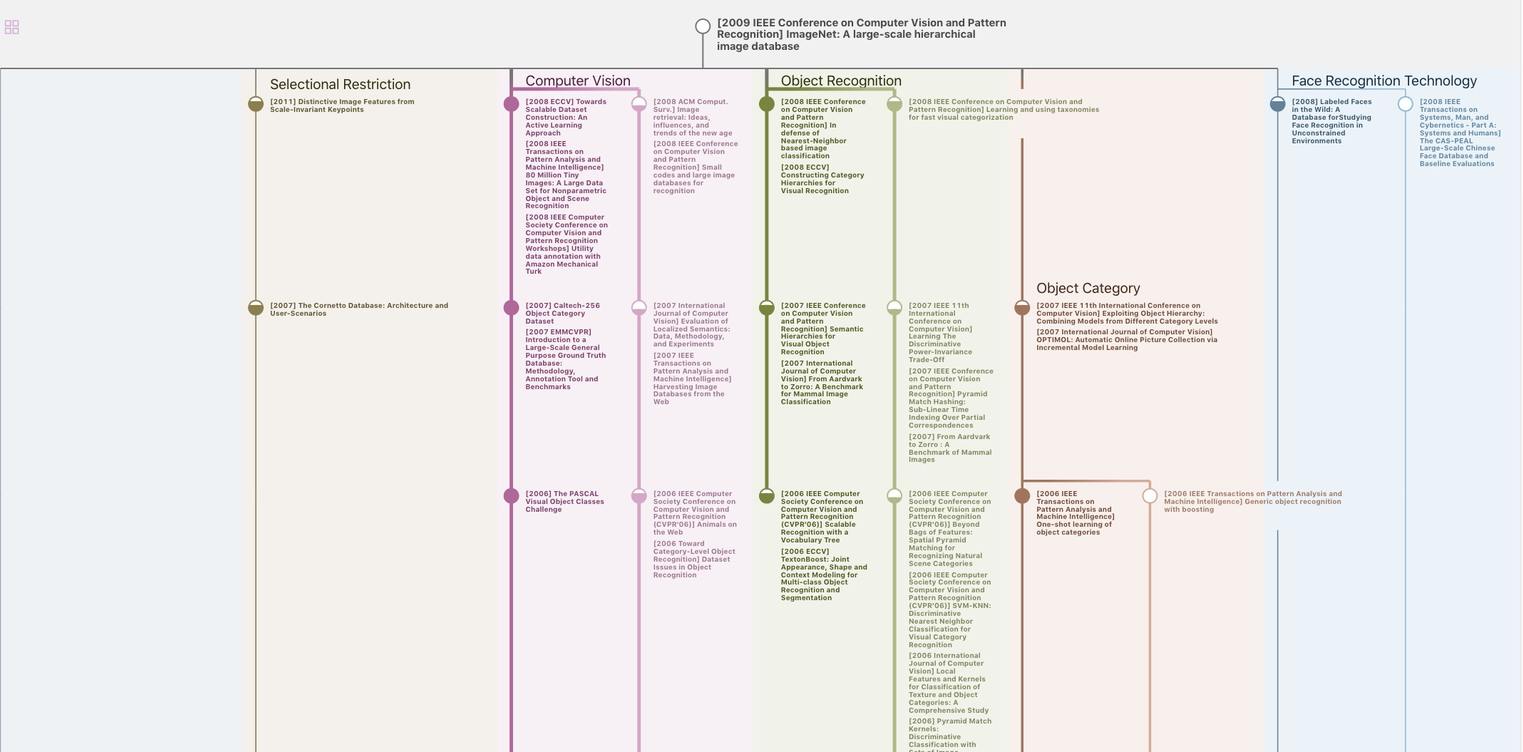
生成溯源树,研究论文发展脉络
Chat Paper
正在生成论文摘要