Information gain Aggregation-based Approach for Time Series Shapelets Discovery
2018 10th International Conference on Knowledge and Systems Engineering (KSE)(2018)
摘要
Time series shapelets is a small subsequence that most efficiently separates time series into different classes using information gain as a measure. It has been recognized that the use of time series shapelets is able to improve both accuracy and explicability of the resulting classifier. By disregarding the distribution information around shapelet candidate in order to calculate information gain, existing shapelets discovery methods may be overfitted to the training data. In this paper, we propose an information gain aggregation mechanism to select less overfitted shapelet candidates. Our idea is to compensate the lost of distribution around the candidates by aggregating information gain of all surrounding subsequences. Experimental results on 24 datasets show that the proposed shapelets discovery method provides higher accuracy compared with the state-of-the-art method. Further, the proposed information gain aggregation mechanism can be applied to all existing shapelets discovery methods for improving their performance.
更多查看译文
关键词
Time Series,Classification,Information Gain,Time Series Shapelets
AI 理解论文
溯源树
样例
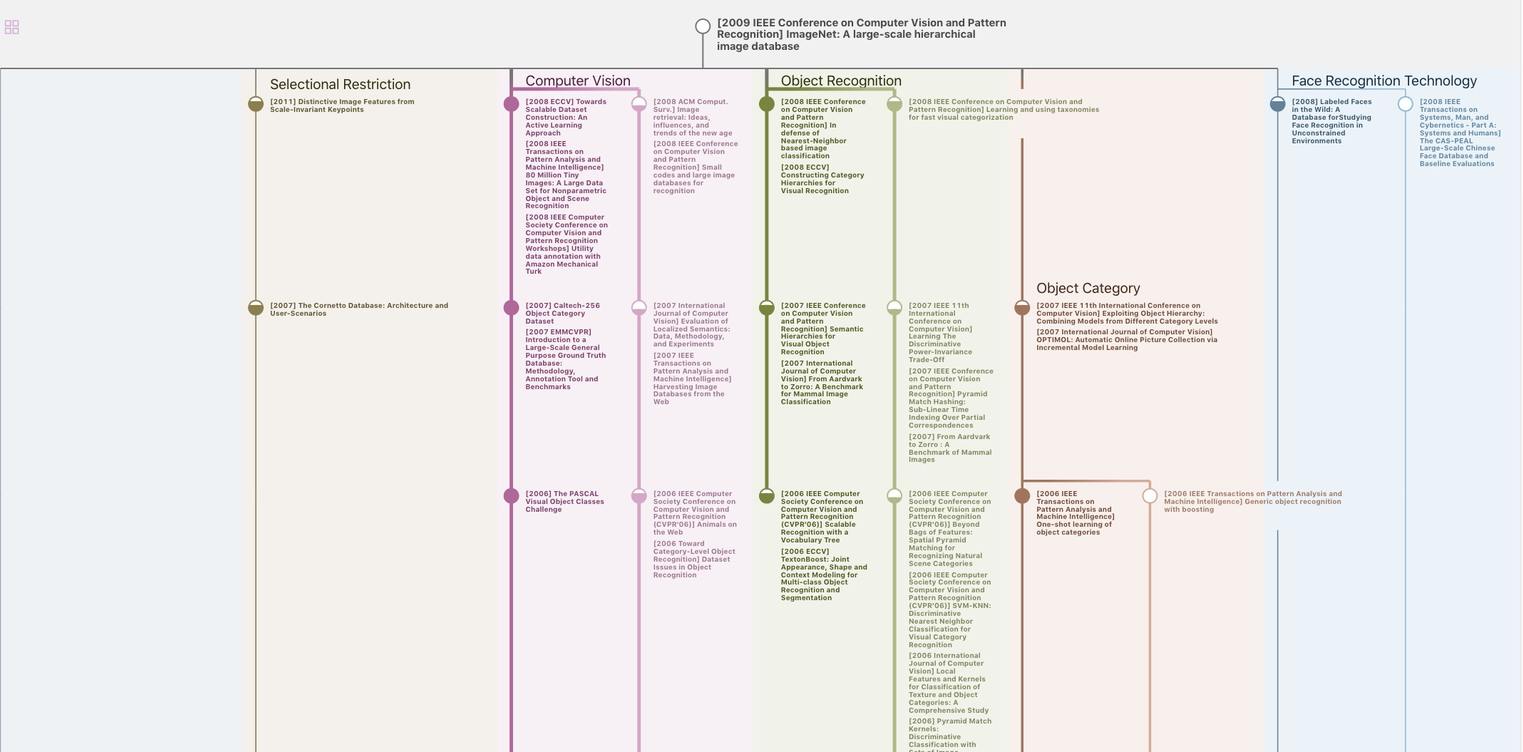
生成溯源树,研究论文发展脉络
Chat Paper
正在生成论文摘要