Multiple Co-clusterings.
ICDM(2018)
摘要
The goal of multiple clusterings is to discover multiple independent ways of organizing a dataset into clusters. Current approaches to this problem just focus on one-way clustering. In many real-world applications, though, it's meaningful and desirable to explore alternative two-way clustering (or co-clusterings), where both samples and features are clustered. To tackle this challenge and unexplored problem, in this paper we introduce an approach, called Multiple Co-Clusterings (MultiCC), to discover non-redundant alternative co-clusterings. MultiCC makes use of matrix tri-factorization to optimize the sample-wise and feature-wise co-clustering indicator matrices, and introduces two non-redundancy terms to enforce diversity among co-clusterings. We then combine the objective of matrix tri-factorization and two non-redundancy terms into a unified objective function and introduce an iterative solution to optimize the function. Experimental results show that MultiCC outperforms existing multiple clustering methods, and it can find interesting co-clusters which cannot be discovered by current solutions.
更多查看译文
关键词
multiple clusterings, co-clustering, matrix tri-factorization, redundancy
AI 理解论文
溯源树
样例
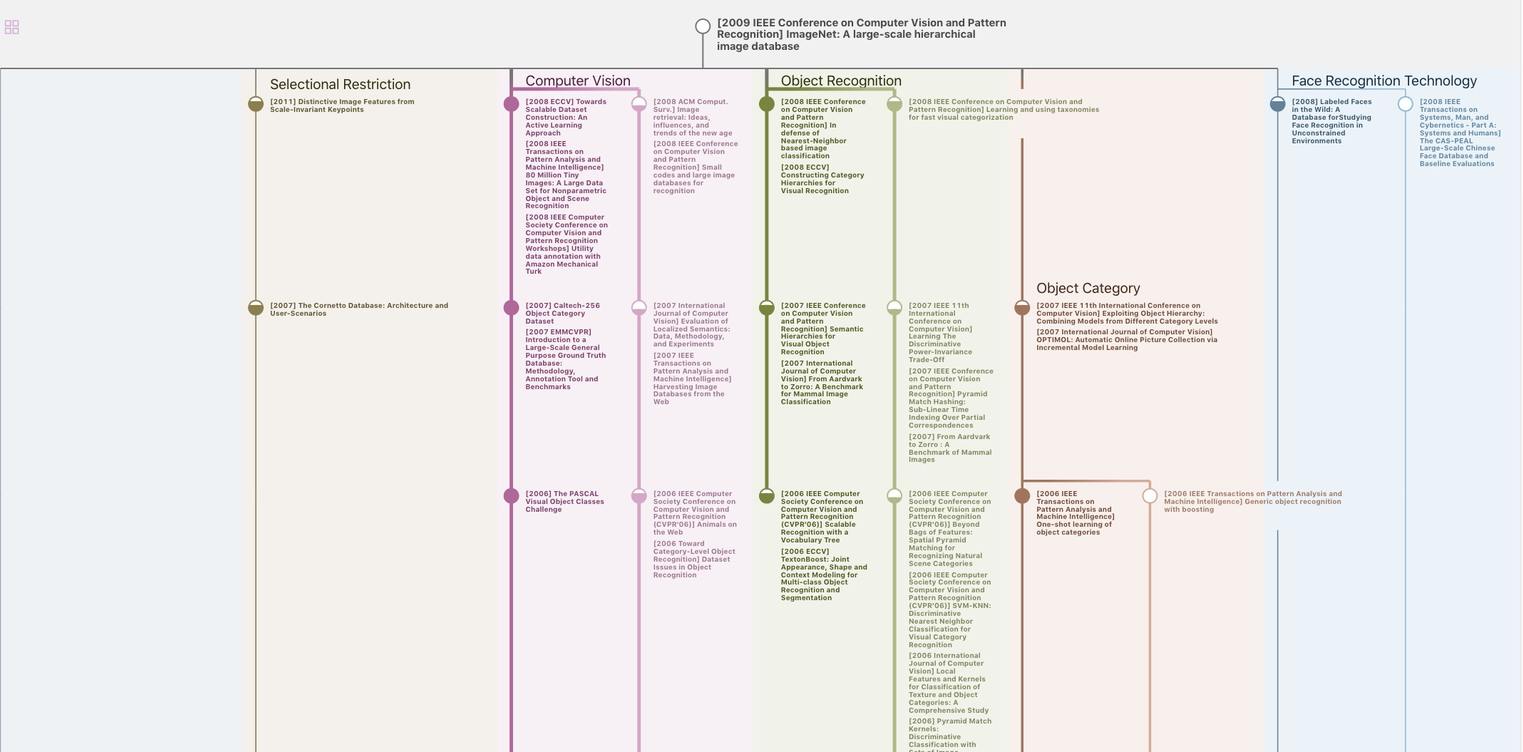
生成溯源树,研究论文发展脉络
Chat Paper
正在生成论文摘要