Sparse Non-Linear Cca Through Hilbert-Schmidt Independence Criterion
2018 IEEE INTERNATIONAL CONFERENCE ON DATA MINING (ICDM)(2018)
摘要
We present SCCA-HSIC, a method for finding sparse non-linear multivariate relations in high-dimensional settings by maximizing the Hilbert-Schmidt Independence Criterion (HSIC). We propose efficient optimization algorithms using a projected stochastic gradient and Nystrom approximation of HSIC. We demonstrate the favourable performance of SCCA-HSIC over competing methods in detecting multivariate non-linear relations both in simulation studies, with varying numbers of related variables, noise variables, and samples, as well as in real datasets.
更多查看译文
关键词
dimensionality reduction, canonical correlation, sparsity, kernel methods, Hilbert-Schmidt Independence Criterion
AI 理解论文
溯源树
样例
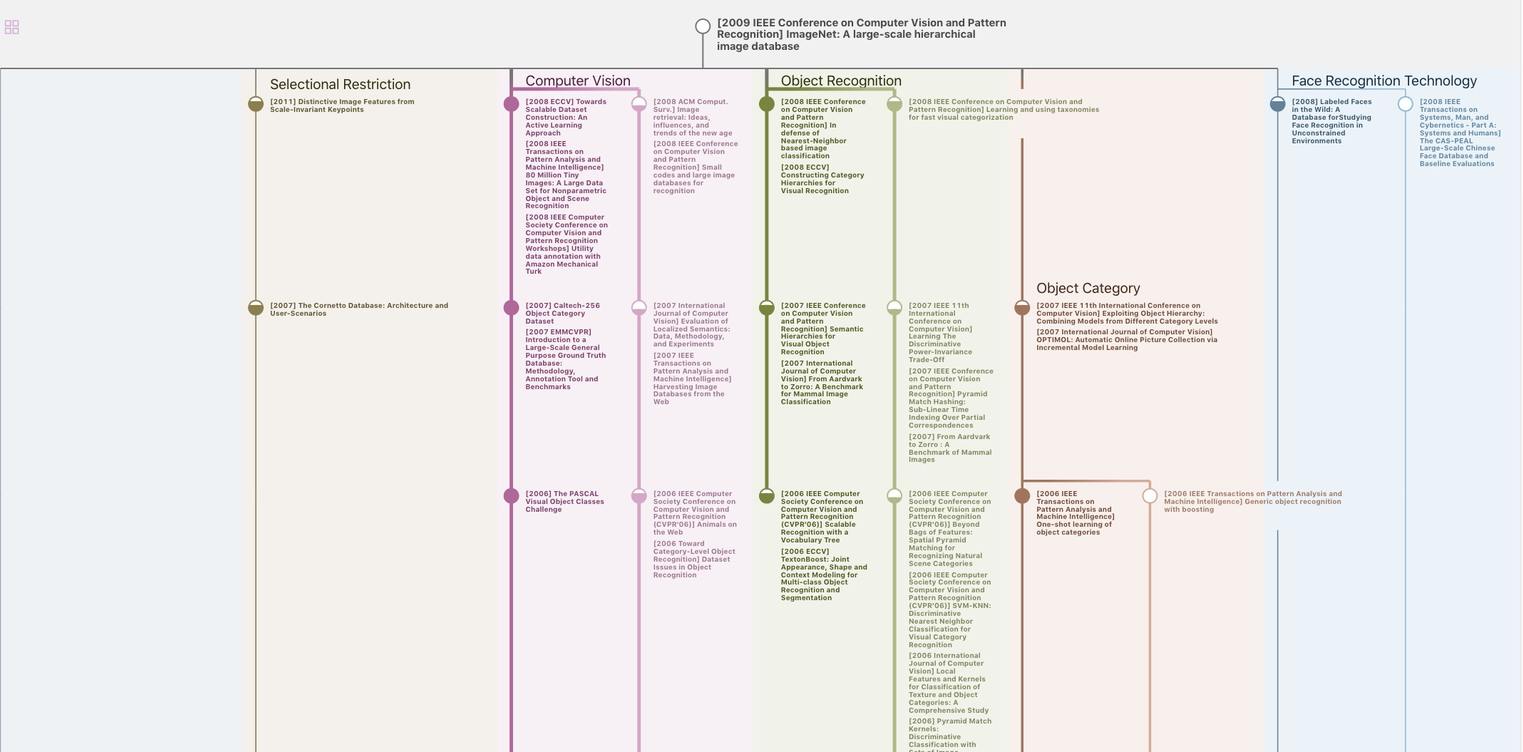
生成溯源树,研究论文发展脉络
Chat Paper
正在生成论文摘要