Generative Adversarial Network for Synthetic Time Series Data Generation in Smart Grids
2018 IEEE International Conference on Communications, Control, and Computing Technologies for Smart Grids (SmartGridComm)(2018)
摘要
The availability of fine grained time series data is a pre-requisite for research in smart-grids. While data for transmission systems is relatively easily obtainable, issues related to data collection, security and privacy hinder the widespread public availability/accessibility of such datasets at the distribution system level. This has prevented the larger research community from effectively applying sophisticated machine learning algorithms to significantly improve the distribution-level accuracy of predictions and increase the efficiency of grid operations. Synthetic dataset generation has proven to be a promising solution for addressing data availability issues in various domains such as computer vision, natural language processing and medicine. However, its exploration in the smart grid context remains unsatisfactory. Previous works have tried to generate synthetic datasets by modeling the underlying system dynamics: an approach which is difficult, time consuming, error prone and often times infeasible in many problems. In this work, we propose a novel data-driven approach to synthetic dataset generation by utilizing deep generative adversarial networks (GAN) to learn the conditional probability distribution of essential features in the real dataset and generate samples based on the learned distribution. To evaluate our synthetically generated dataset, we measure the maximum mean discrepancy (MMD) between real and synthetic datasets as probability distributions, and show that their sampling distance converges. To further validate our synthetic dataset, we perform common smart grid tasks such as k-means clustering and short-term prediction on both datasets. Experimental results show the efficacy of our synthetic dataset approach: the real and synthetic datasets are indistinguishable by solely examining the output of these tasks.
更多查看译文
关键词
generative adversarial network,synthetic time series data generation,transmission systems,distribution system level,grid operations,synthetic dataset generation,data availability issues,natural language processing,smart grid context,deep generative adversarial networks,conditional probability distribution,probability distributions,common smart grid tasks,synthetic dataset approach,machine learning algorithms,data-driven approach,maximum mean discrepancy
AI 理解论文
溯源树
样例
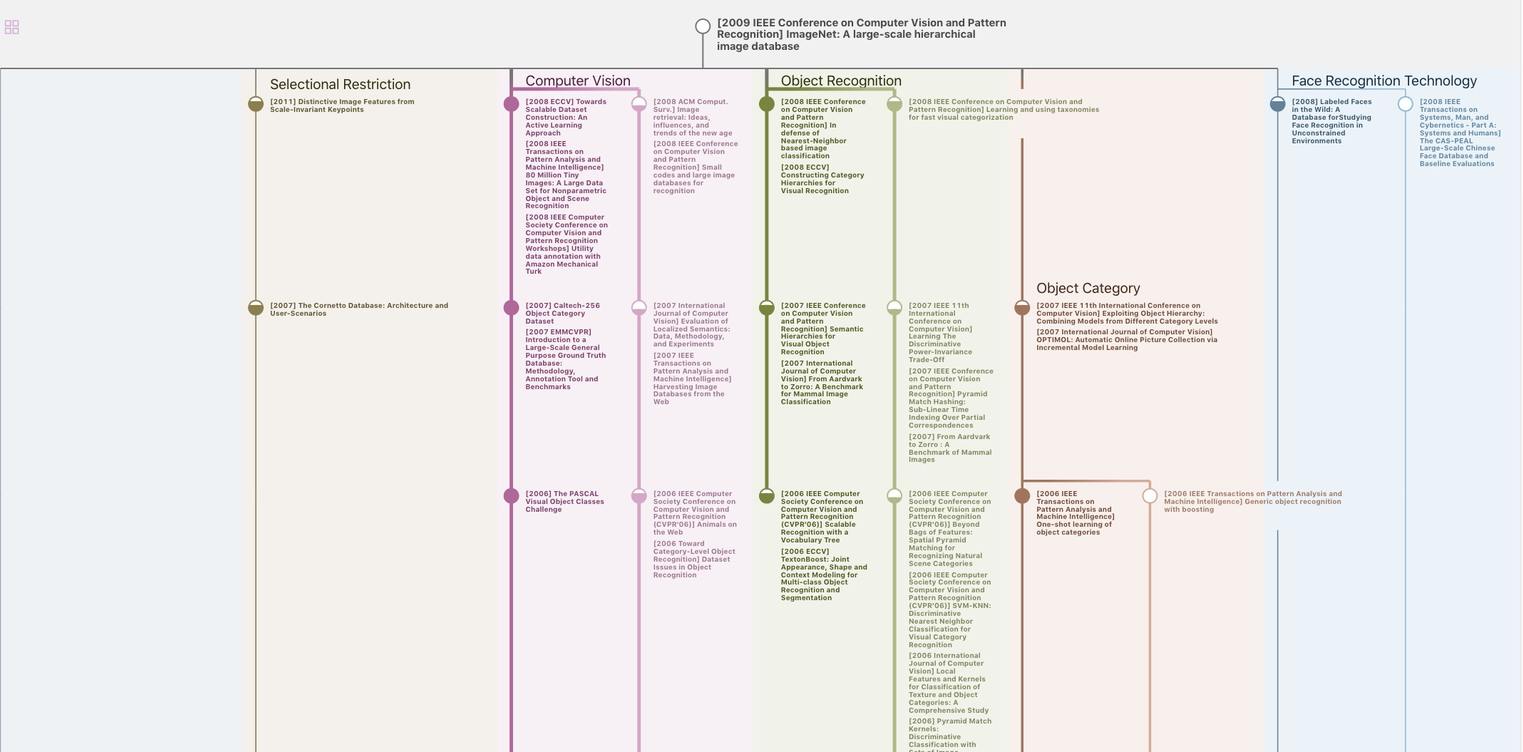
生成溯源树,研究论文发展脉络
Chat Paper
正在生成论文摘要