Machine-learning analysis outperforms conventional statistical models and CT classification systems in predicting 6-month outcomes in pediatric patients sustaining traumatic brain injury.
NEUROSURGICAL FOCUS(2018)
摘要
OBJECTIVE Modern surgical planning and prognostication requires the most accurate outcomes data to practice evidence-based medicine. For clinicians treating children following traumatic brain injury (TBI) these data are severely lacking. The first aim of this study was to assess published CT classification systems in the authors' pediatric cohort. A pediatric-specific machine-learning algorithm called an artificial neural network (ANN) was then created that robustly outperformed traditional CT classification systems in predicting TBI outcomes in children. METHODS The clinical records of children under the age of 18 who suffered a TBI and underwent head CT within 24 hours after TBI (n = 565) were retrospectively reviewed. RESULTS "Favorable" outcome (alive with Glasgow Outcome Scale [GOS] score >= 4 at 6 months postinjury, n = 533) and "unfavorable" outcome (death at 6 months or GOS score <= 3 at 6 months postinjury, n = 32) were used as the primary outcomes. The area under the receiver operating characteristic (ROC) curve (AUC) was used to delineate the strength of each CT grading system in predicting survival (Helsinki, 0.814; Rotterdam, 0.838; and Marshall, 0.781). The AUC for CT score in predicting GOS score <= 3, a measure of overall functionality, was similarly predictive (Helsinki, 0.717; Rotterdam, 0.748; and Marshall, 0.663). An ANN was then constructed that was able to predict 6-month outcomes with profound accuracy (AUC = 0.9462 +/- 0.0422). CONCLUSIONS This study showed that machine-learning can be leveraged to more accurately predict TBI outcomes in children.
更多查看译文
关键词
neurotrauma,traumatic brain injury,artificial neural network
AI 理解论文
溯源树
样例
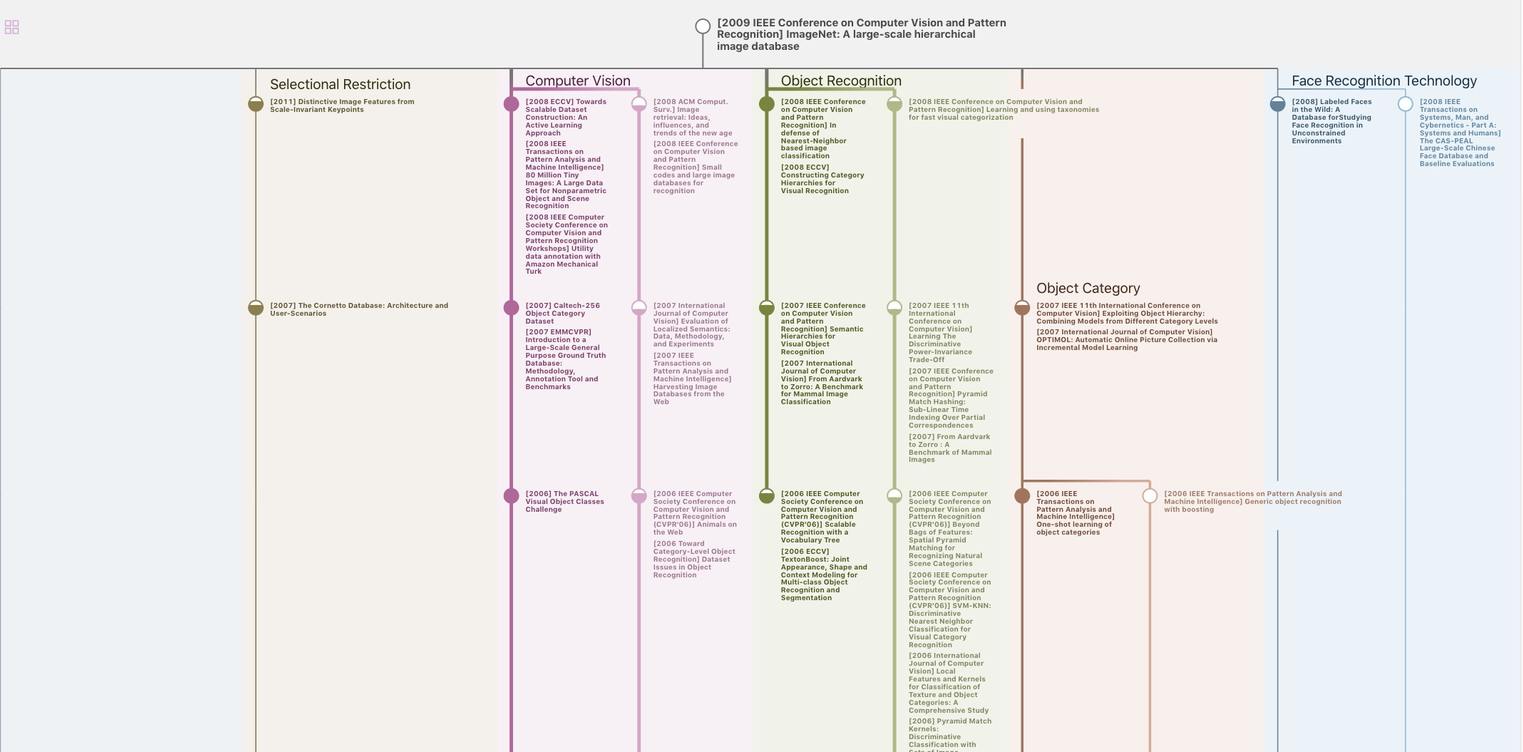
生成溯源树,研究论文发展脉络
Chat Paper
正在生成论文摘要