Stochastic Gradient Descent for Spectral Embedding with Implicit Orthogonality Constraint
ICASSP 2019 - 2019 IEEE International Conference on Acoustics, Speech and Signal Processing (ICASSP)(2019)
摘要
In this paper, we propose a scalable algorithm for spectral embedding. The latter is a standard tool for graph clustering. However, its computational bottleneck is the eigendecomposition of the graph Laplacian matrix, which prevents its application to large-scale graphs. Our contribution consists of reformulating spectral embedding so that it can be solved via stochastic optimization. The idea is to replace the orthogonality constraint with an orthogonalization matrix injected directly into the criterion. As the gradient can be computed through a Cholesky factorization, our reformulation allows us to develop an efficient algorithm based on mini-batch gradient descent. Experimental results, both on synthetic and real data, confirm the efficiency of the proposed method in term of execution speed with respect to similar existing techniques.
更多查看译文
关键词
Spectral Clustering,Stochastic Optimization,Cholesky factorization,Multilayer Graph
AI 理解论文
溯源树
样例
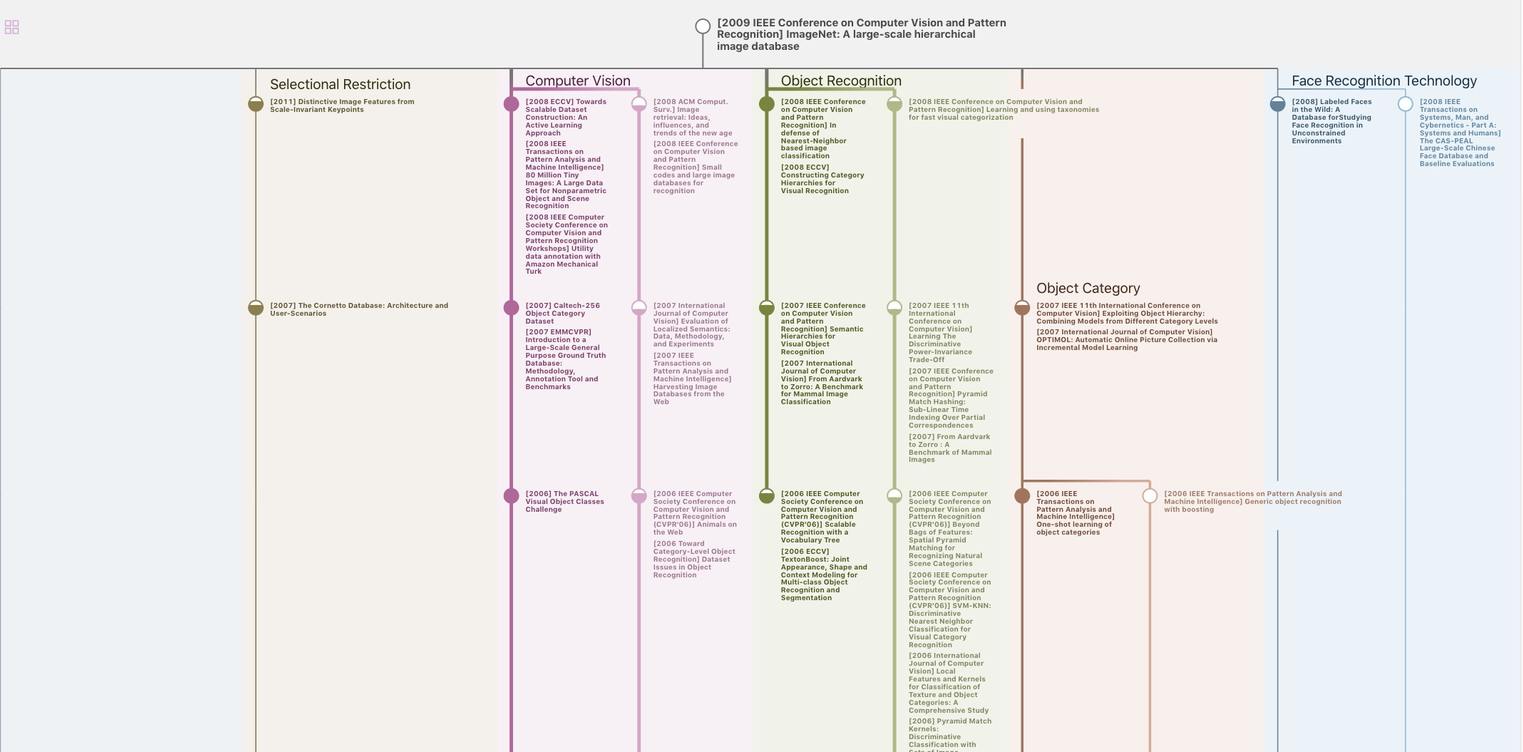
生成溯源树,研究论文发展脉络
Chat Paper
正在生成论文摘要