Evaluating the Impact of Intensity Normalization on MR Image Synthesis.
Proceedings of SPIE(2019)
摘要
Image synthesis learns a transformation from the intensity features of an input image to yield a different tissue contrast of the output image. This process has been shown to have application in many medical image analysis tasks including imputation, registration, and segmentation. To carry out synthesis, the intensities of the input images are typically scaled-i.e., normalized-both in training to learn the transformation and in testing when applying the transformation, but it is not presently known what type of input scaling is optimal. In this paper, we consider seven different intensity normalization algorithms and three different synthesis methods to evaluate the impact of normalization. Our experiments demonstrate that intensity normalization as a preprocessing step improves the synthesis results across all investigated synthesis algorithms. Furthermore, we show evidence that suggests intensity normalization is vital for successful deep learning-based MR image synthesis.
更多查看译文
关键词
intensity normalization,image synthesis,brain MRI
AI 理解论文
溯源树
样例
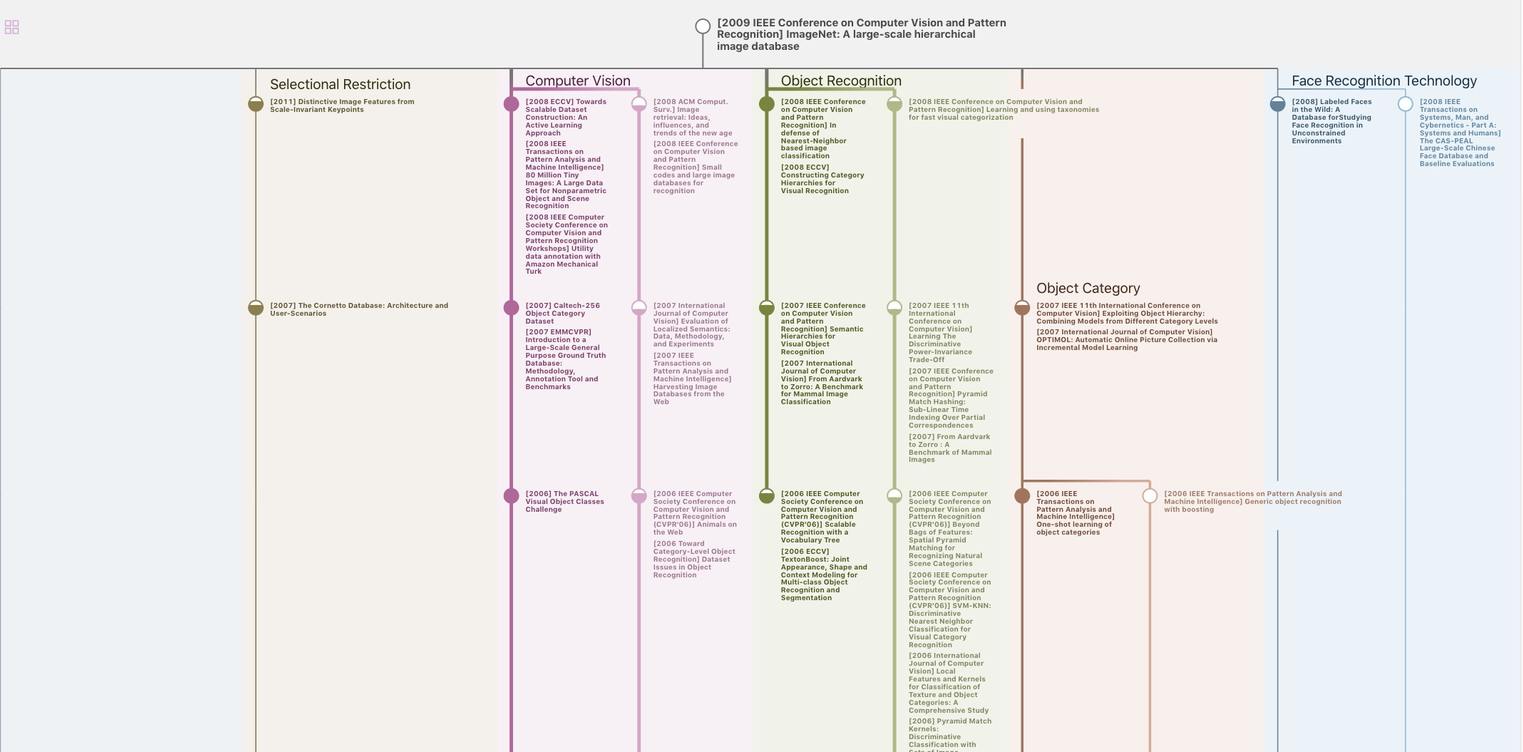
生成溯源树,研究论文发展脉络
Chat Paper
正在生成论文摘要