3D Point Cloud Learning for Large-scale Environment Analysis and Place Recognition.
arXiv: Computer Vision and Pattern Recognition(2018)
摘要
In this paper, we develop a new deep neural network which can extract discriminative and generalizable global descriptors from the raw 3D point cloud. Specifically, two novel modules, Adaptive Local Feature Extraction and Graph-based Neighborhood Aggregation, are designed and integrated into our network. This contributes to extract the local features adequately, reveal the spatial distribution of the point cloud, and find out the local structure and neighborhood relations of each part in a large-scale point cloud with an end-to-end manner. Furthermore, we utilize the network output for point cloud based analysis and retrieval tasks to achieve large-scale place recognition and environmental analysis. We tested our approach on the Oxford RobotCar dataset. The results for place recognition increased the existing state-of-the-art result (PointNetVLAD) from 81.01% to 94.92%. Moreover, we present an application to analyze the large-scale environment by evaluating the uniqueness of each location in the map, which can be applied to localization and loop-closure tasks, which are crucial for robotics and self-driving applications.
更多查看译文
AI 理解论文
溯源树
样例
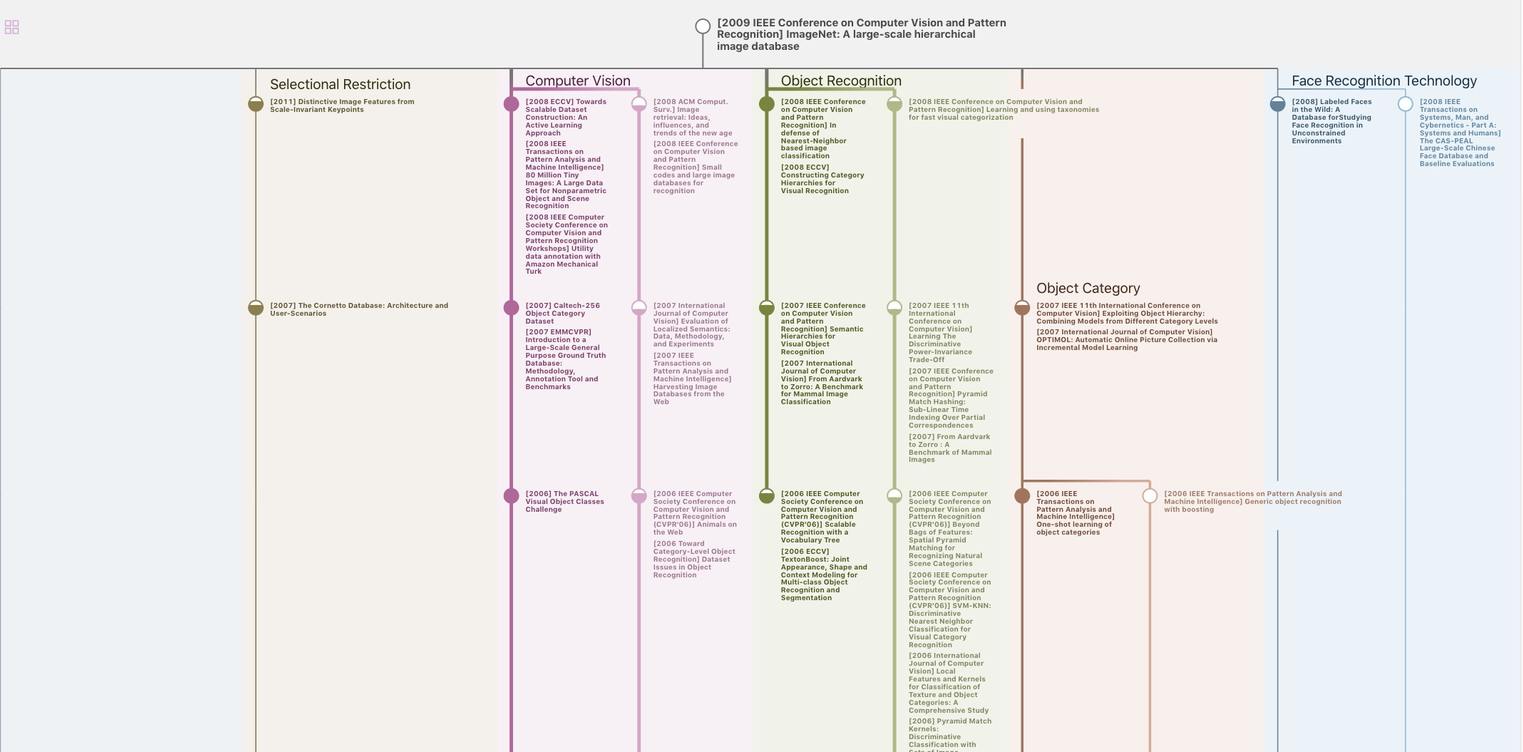
生成溯源树,研究论文发展脉络
Chat Paper
正在生成论文摘要