Benchmarking Keyword Spotting Efficiency on Neuromorphic Hardware
PROCEEDINGS OF THE 2019 7TH ANNUAL NEURO-INSPIRED COMPUTATIONAL ELEMENTS WORKSHOP (NICE 2019)(2018)
摘要
Using Intel's Loihi neuromorphic research chip and ABR's Nengo Deep Learning toolkit, we analyze the inference speed, dynamic power consumption, and energy cost per inference of a two-layer neural network keyword spotter trained to recognize a single phrase. We perform comparative analyses of this keyword spotter running on more conventional hardware devices including a CPU, a GPU, Nvidia's Jetson TX1, and the Movidius Neural Compute Stick. Our results indicate that for this inference application, Loihi outperforms all of these alternatives on an energy cost per inference basis while maintaining equivalent inference accuracy. Furthermore, an analysis of tradeoffs between network size, inference speed, and energy cost indicates that Loihi's comparative advantage over other low-power computing devices improves for larger networks.
更多查看译文
关键词
keyword spotting, speech recognition, machine learning, neuromorphic computing, spiking neural networks
AI 理解论文
溯源树
样例
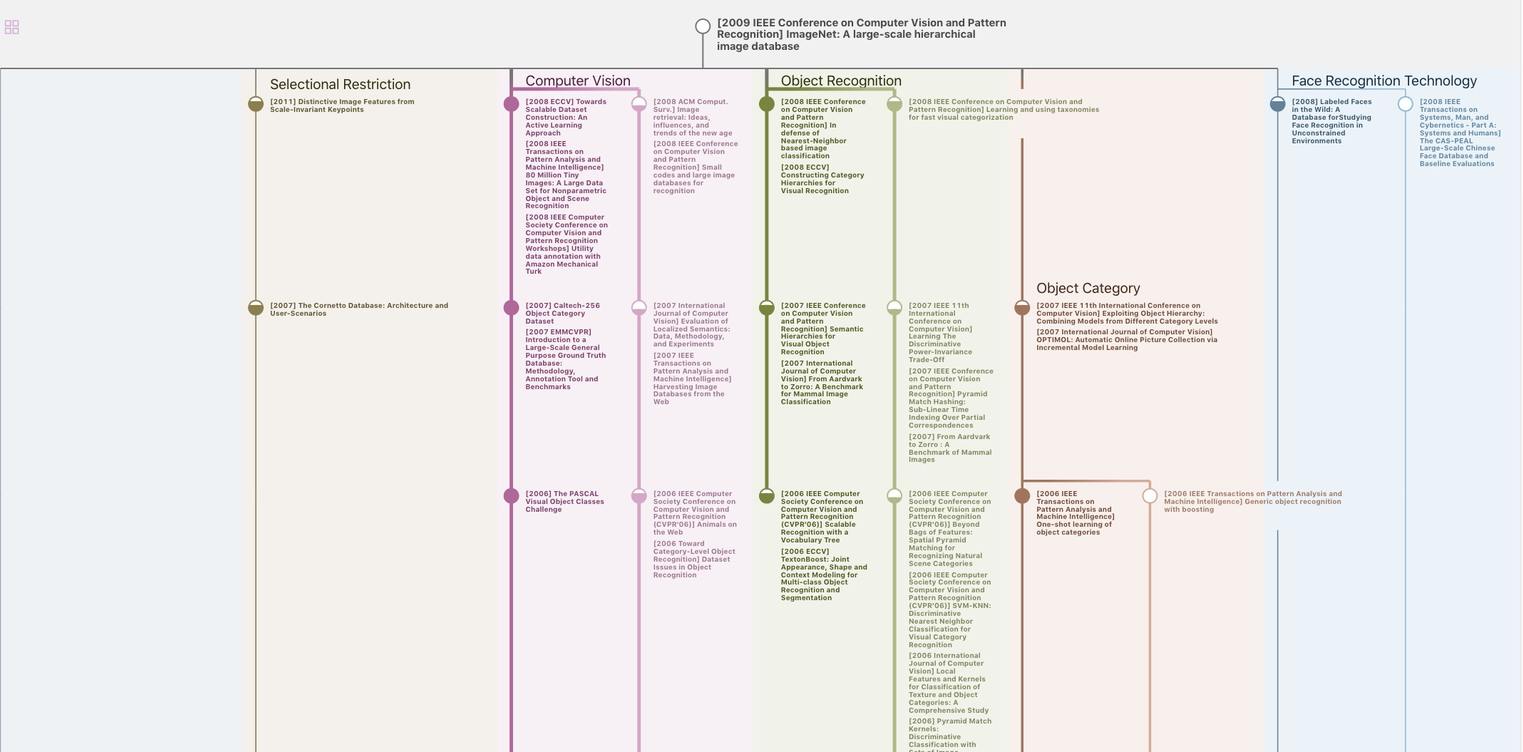
生成溯源树,研究论文发展脉络
Chat Paper
正在生成论文摘要