Learning to Drive from Simulation without Real World Labels
2019 International Conference on Robotics and Automation (ICRA)(2018)
摘要
Simulation can be a powerful tool for understanding machine learning systems and designing methods to solve real-world problems. Training and evaluating methods purely in simulation is often "doomed to succeed" at the desired task in a simulated environment, but the resulting models are incapable of operation in the real world. Here we present and evaluate a method for transferring a vision-based lane following driving policy from simulation to operation on a rural road without any real-world labels. Our approach leverages recent advances in image-to-image translation to achieve domain transfer while jointly learning a single-camera control policy from simulation control labels. We assess the driving performance of this method using both open-loop regression metrics, and closed-loop performance operating an autonomous vehicle on rural and urban roads.
更多查看译文
关键词
domain transfer,single-camera control policy,simulation control labels,driving performance,rural roads,urban roads,machine learning systems,simulated environment,vision-based lane,driving policy,rural road,image-to-image translation,autonomous vehicle,open-loop regression metric
AI 理解论文
溯源树
样例
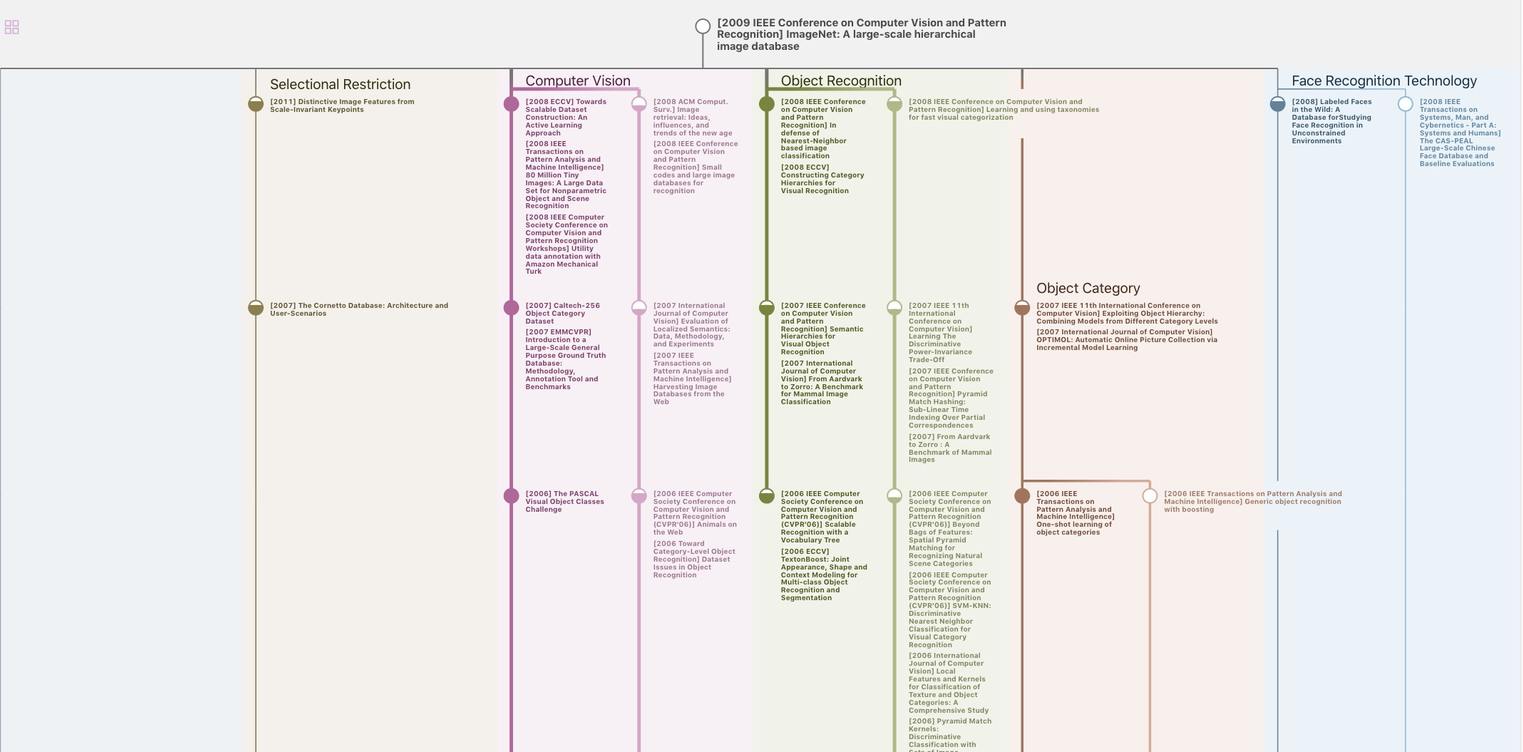
生成溯源树,研究论文发展脉络
Chat Paper
正在生成论文摘要