Moving Target Indication Using Deep Convolutional Neural Network.
IEEE ACCESS(2018)
摘要
Existing moving target indication (MTI) methods are limited either by the sufficient and homogeneous secondary data (SD) condition or by the high signal-to-clutter-ratio (SCR) requirement. In practice, such constraints are hard to be satisfied. In this paper, we propose a novel deep convolutional neural network (CNN)-based method for the MTI (CNN-MTI) to overcome the limitations of these methods. In the proposed method, the CNN architecture is deliberately designed, and the training data set is greatly augmented to ensure the CNN be deep and well-trained; in addition, the SD only act as the background interference during the augmentation of the training data set. These procedures ensure that the effective features of different classes of moving targets can be extracted, so that good MTI performance can be achieved without the requirement of the sufficient and homogeneous SD or the high SCR condition. Simulation results from the synthetic data and the experimental data demonstrate the validity and the robustness of the CNN-MTI with limited SD support in non-homogeneous and low SCR environment.
更多查看译文
关键词
Moving target indication (MTI),convolution neural network (CNN),space-time adaptive processing (STAP),feature extraction
AI 理解论文
溯源树
样例
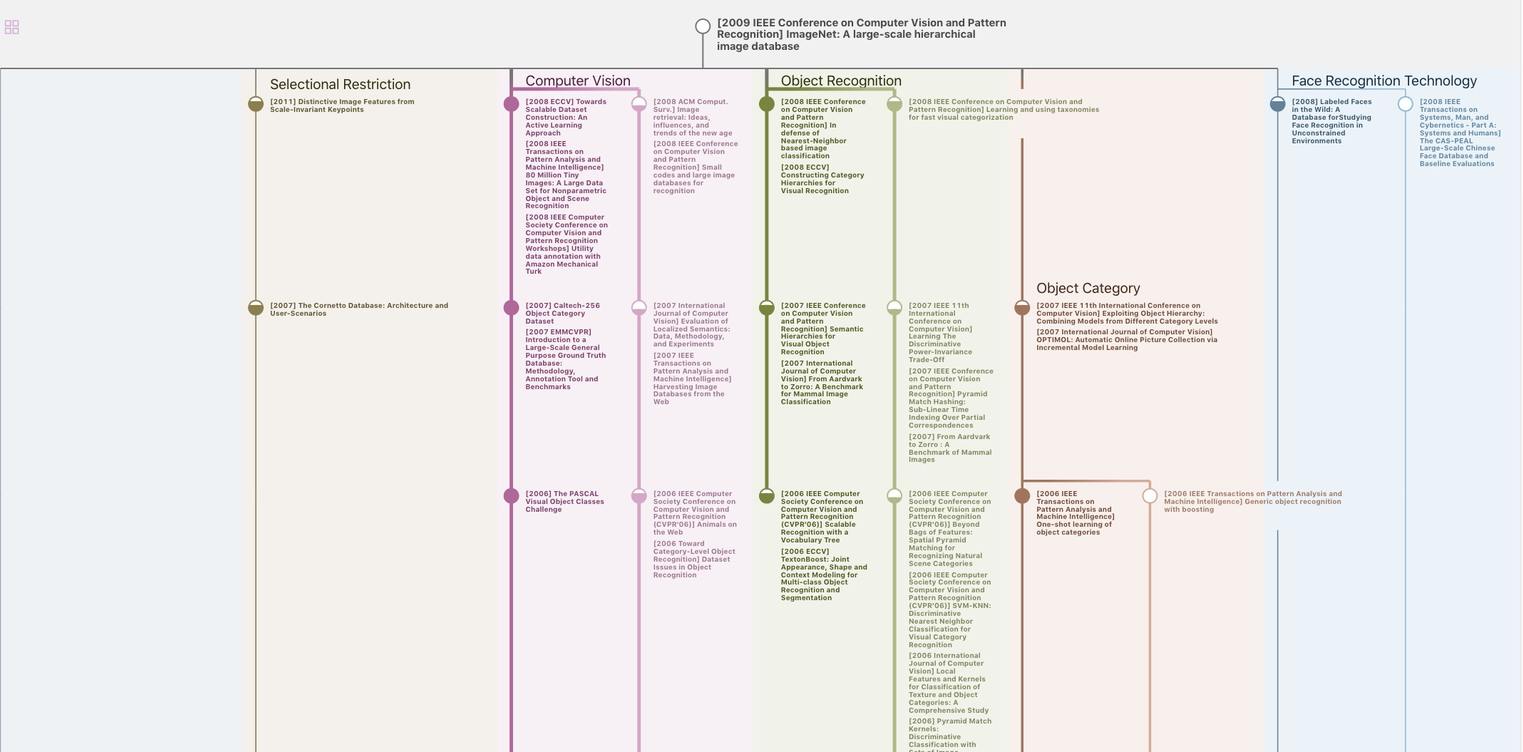
生成溯源树,研究论文发展脉络
Chat Paper
正在生成论文摘要