Accelerating Topic Detection on Web for a Large-Scale Data Set via Stochastic Poisson Deconvolution.
MMM(2019)
摘要
Organizing webpages into hot topics is one of the key steps to understand the trends from multi-modal web data. To handle this pressing problem, Poisson Deconvolution (PD), a state-of-the-art method, recently is proposed to rank the interestingness of web topics on a similarity graph. Nevertheless, in terms of scalability, PD optimized by expectation-maximization is not sufficiently efficient for a large-scale data set. In this paper, we develop a Stochastic Poisson Deconvolution (SPD) to deal with the large-scale web data sets. Experiments demonstrate the efficacy of the proposed approach in comparison with the state-of-the-art methods on two public data sets and one large-scale synthetic data set.
更多查看译文
关键词
Large-scale, Poisson Deconvolution, Unsupervised ranking, Web topic detection, Surrogate function
AI 理解论文
溯源树
样例
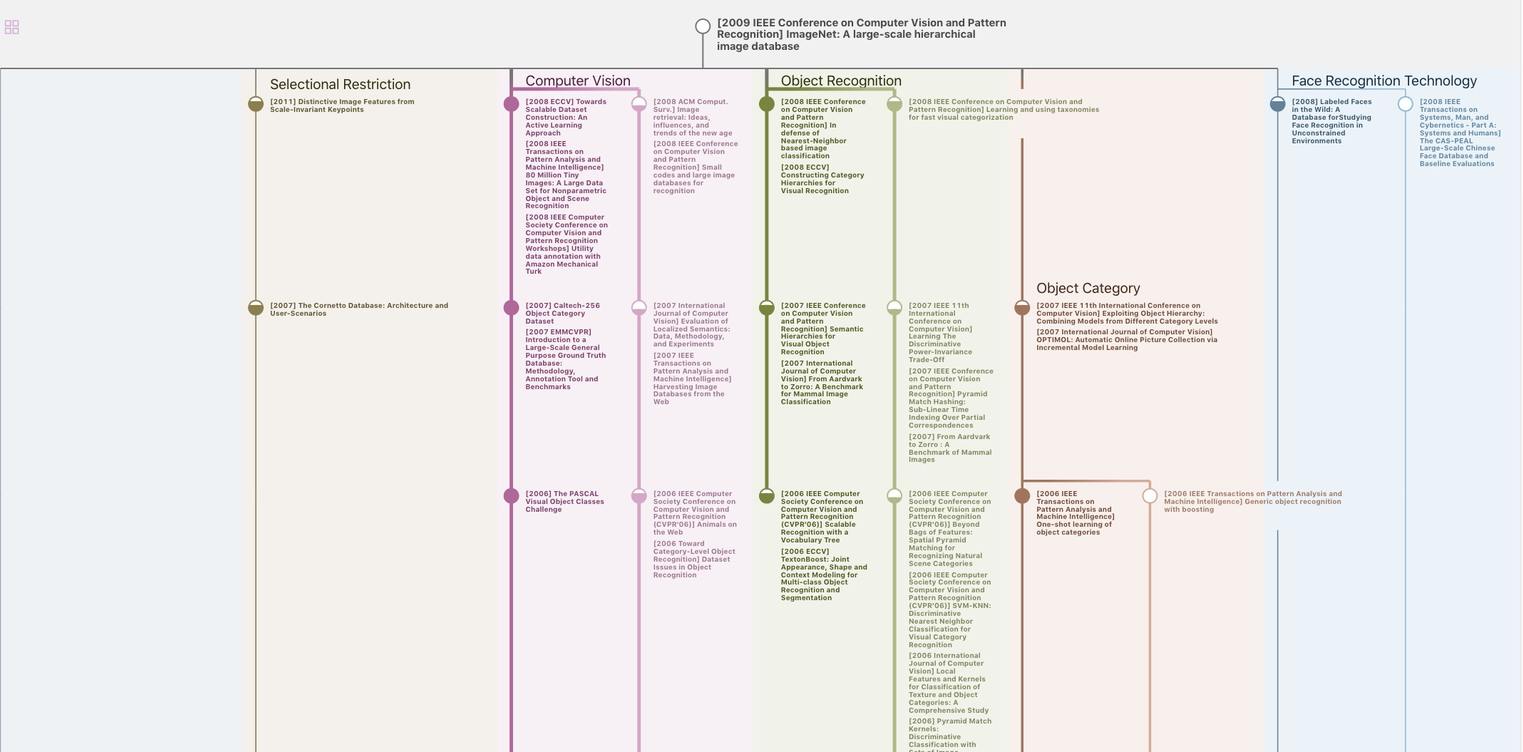
生成溯源树,研究论文发展脉络
Chat Paper
正在生成论文摘要