Fvr-Sgd: A New Flexible Variance-Reduction Method For Sgd On Large-Scale Datasets
NEURAL INFORMATION PROCESSING (ICONIP 2018), PT II(2018)
摘要
Stochastic gradient descent (SGD) is a popular optimization method widely-used in machine learning, while the variance of gradient estimation leads to slow convergence. To accelerate the speed, many variance reduction methods have been proposed. However, most of these methods require additional memory cost or computational burden on full gradient, which results in low efficiency or even unavailable while applied to real-world applications with large-scale datasets. To handle this issue, we propose a new flexible variance reduction method for SGD, named FVR-SGD, which can reduce memory overhead and speedup the convergence using flexible subset size without extra operation. The details of convergence property are presented, the convergence of variance reduction method using flexible subset size can be guaranteed. Several numerical experiments are conducted on a genre of real-world large-scale datasets. The experimental results demonstrated that FVR-SGD outperforms contemporary SVRG algorithm. Specifically, the proposed method can achieve up to 40% reduction in the training time to solve the optimization problem of logistic regression.
更多查看译文
关键词
Machine learning, Optimization, Stochastic gradient descent, Variance reduction, Distributed optimization
AI 理解论文
溯源树
样例
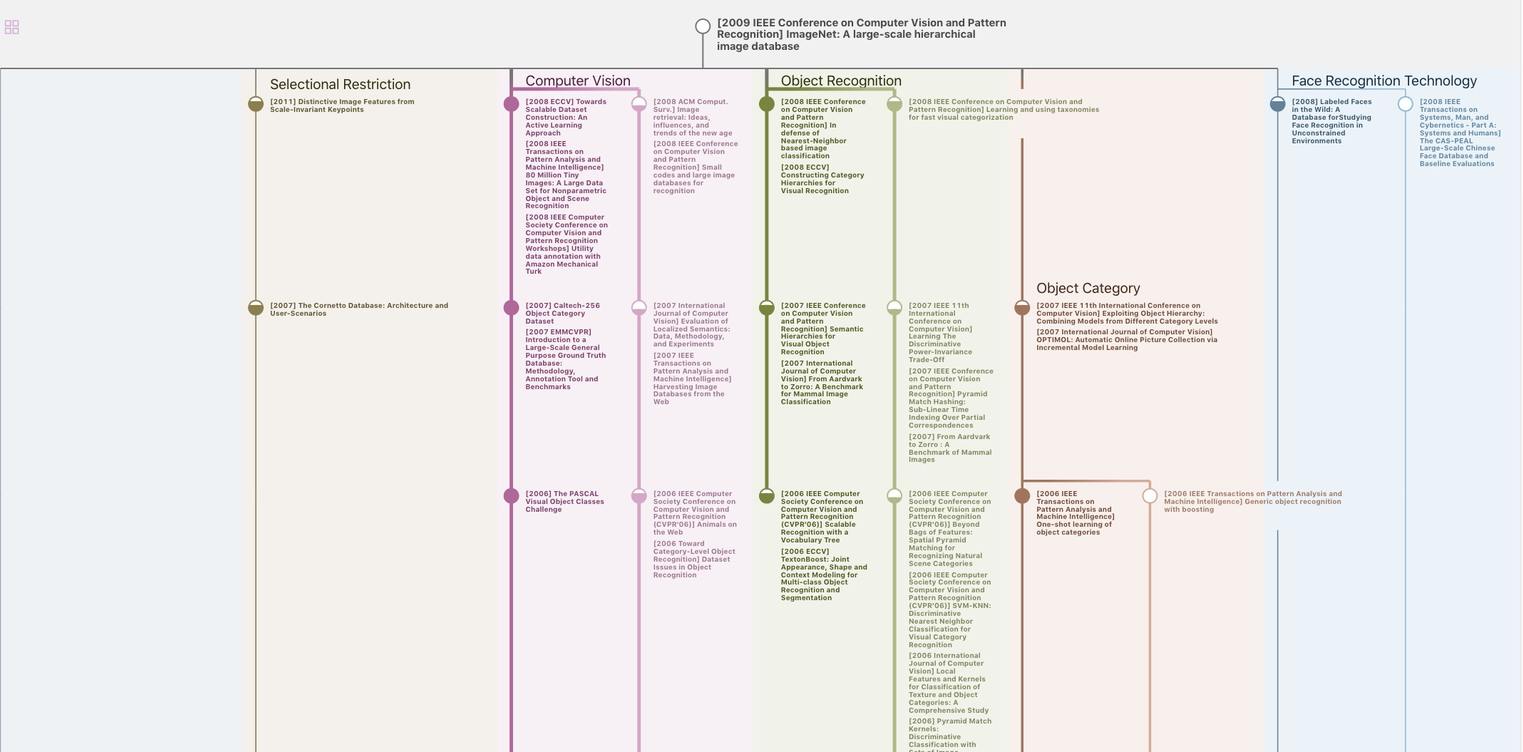
生成溯源树,研究论文发展脉络
Chat Paper
正在生成论文摘要