A Least Squares Approach To Region Selection
NEURAL INFORMATION PROCESSING (ICONIP 2018), PT V(2018)
摘要
Region selection is able to boost the recognition performance for images with background clutter by discovering the object regions. In this paper, we propose a region selection method under the least squares framework. With the assumption that an object is a combination of several over-segmented regions, we impose a selection variable on each region, and employ a linear model to perform classification. The model parameter and the selection parameter are alternatively updated to minimize a sum-of-squares error function. During the iteration, the selection parameter can automatically pick the discriminant regions accounting for the object category, then fine tunes the linear model with the objects, independently of the background. As a result, the learnt model is able to distinguish object regions and non-object regions, which actually generates irregular-shape object localization. Our method performs significantly better than the baselines on two datasets, and the performance can be further improved when combining deep CNN features. Moreover, the algorithm is easy to implement and computationally efficient because of the merits inherited from the least squares.
更多查看译文
关键词
Least squares, Region selection, Object localization
AI 理解论文
溯源树
样例
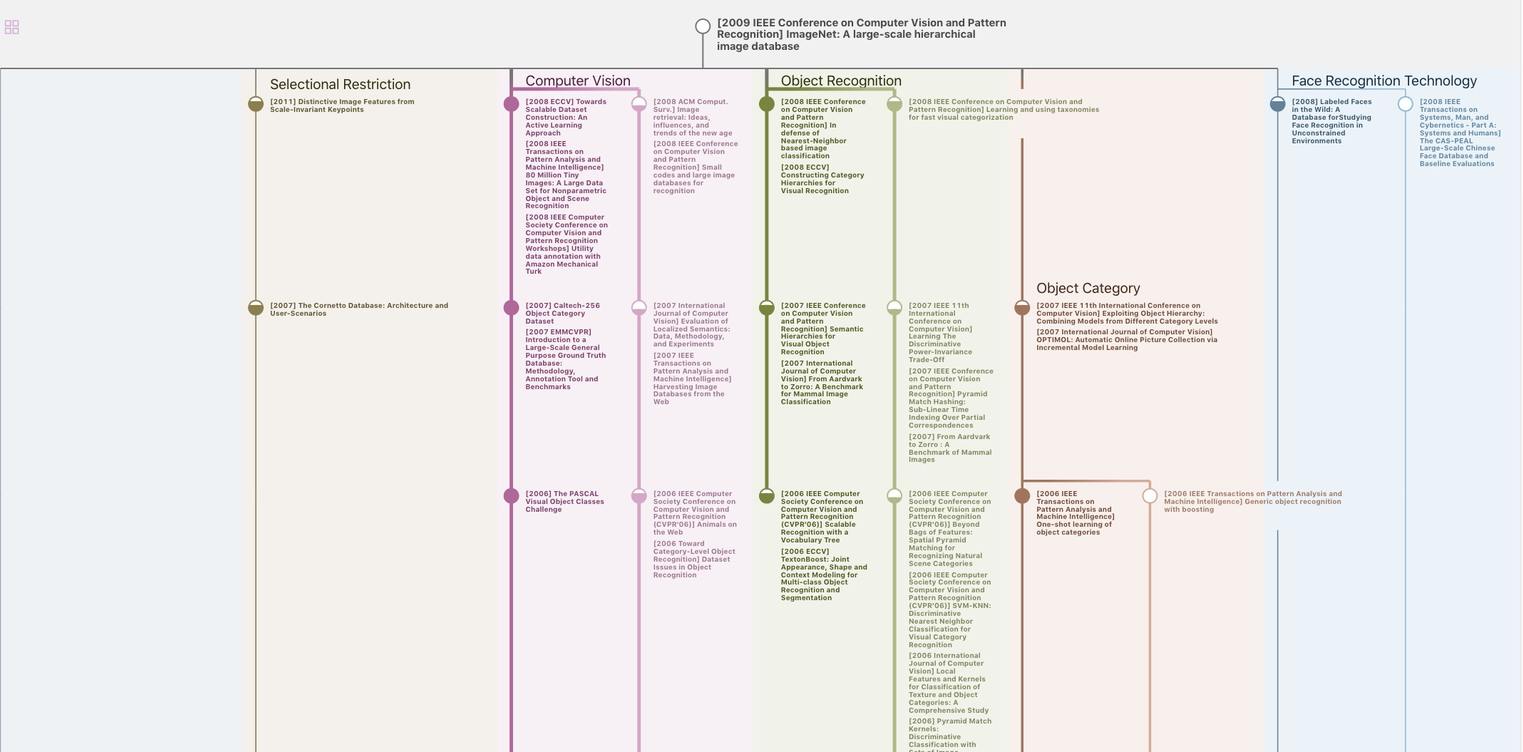
生成溯源树,研究论文发展脉络
Chat Paper
正在生成论文摘要