Nvm Weight Variation Impact On Analog Spiking Neural Network Chip
NEURAL INFORMATION PROCESSING (ICONIP 2018), PT VII(2018)
摘要
In extremely energy-efficient neuromorphic computing using analog non-volatile memory (NVM) devices, device variability arises due to process variation and electro/thermo-dynamics of NVM devices, such as phase change memory and resistive-RAM. Thus, for realizing NVM-based neuromorphic computing, it is important to quantitatively analyze the impact of synaptic device variability on neural network training accuracy and assess requirements for NVM devices. We investigated the analysis using simulations focusing on a spiking neural network (SNN)-based restricted Boltzmann machine (RBM). MNIST dataset simulation results revealed that more than 500 steps of conductance achieve comparable performance to the previous study of software-based simulation on SNN-based RBM. We also observed that at least a less than 10% of variation in conductance update for each synaptic device is required for achieving comparable performance to the result with no variation. These results provide baselines for designing and optimizing the characteristics of NVM devices.
更多查看译文
关键词
Spiking neural network, Neuromorphic computing, Restricted Boltzmann machine, Spike-timing-dependent plasticity, Phase change memory, Resistive-RAM
AI 理解论文
溯源树
样例
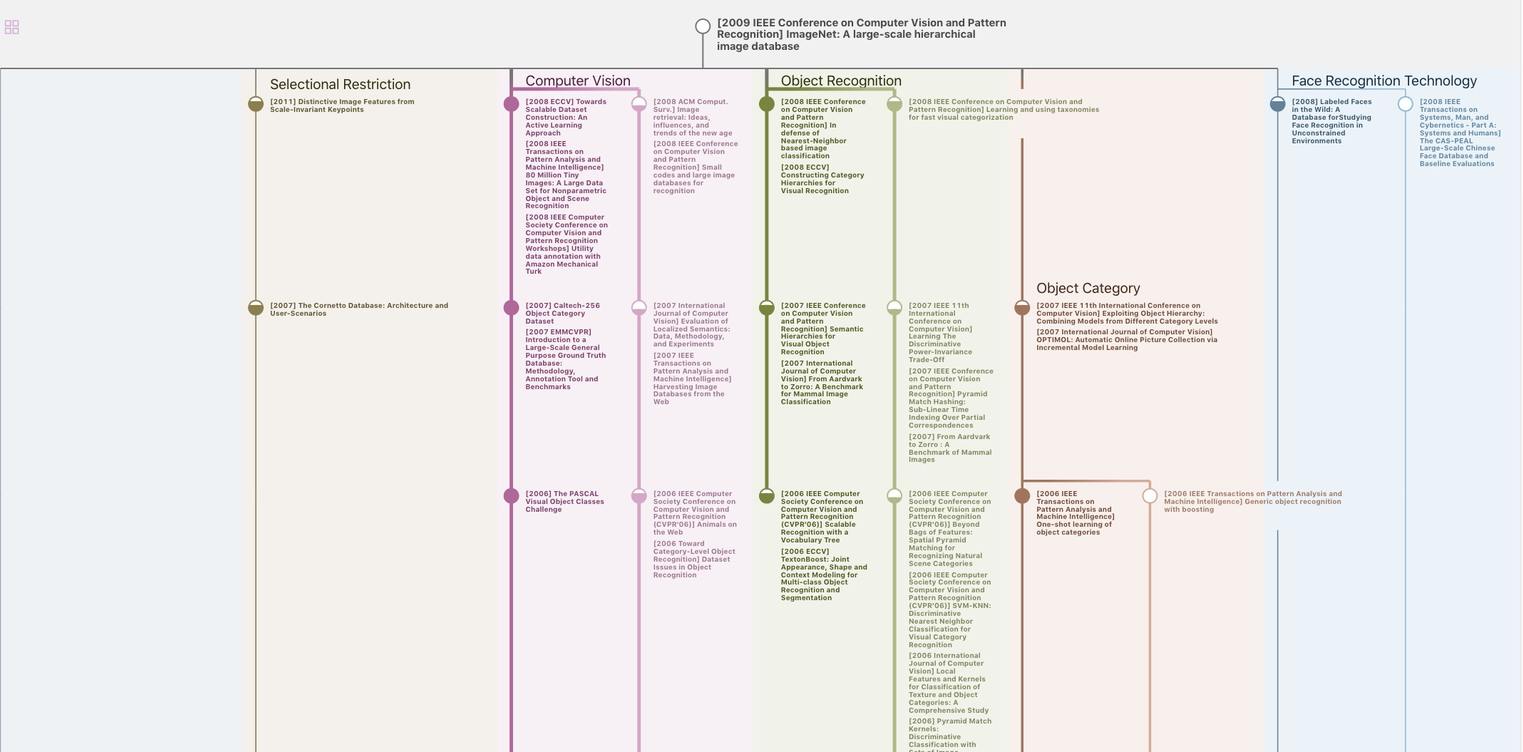
生成溯源树,研究论文发展脉络
Chat Paper
正在生成论文摘要