Hybrid Networks: Improving Deep Learning Networks Via Integrating Two Views Of Images
NEURAL INFORMATION PROCESSING (ICONIP 2018), PT I(2018)
摘要
The principal component analysis network (PCANet) is an unsupervised parsimonious deep network, utilizing principal components as filters in the layers. It creates an amalgamated view of the data by transforming it into column vectors which destroys its spatial structure while obtaining the principal components. In this research, we first propose a tensor-factorization based method referred as the Tensor Factorization Networks (TFNet). The TFNet retains the spatial structure of the data by preserving its individual modes. This presentation provides a minutiae view of the data while extracting matrix factors. However, the above methods are restricted to extract a single representation and thus incurs information loss. To alleviate this information loss with the above methods we propose Hybrid Network (HybridNet) to simultaneously learn filters from both the views of the data. Comprehensive results on multiple benchmark datasets validate the superiority of integrating both the views of the data in our proposed HybridNet.
更多查看译文
关键词
Tensor decomposition, Classification, Feature extraction
AI 理解论文
溯源树
样例
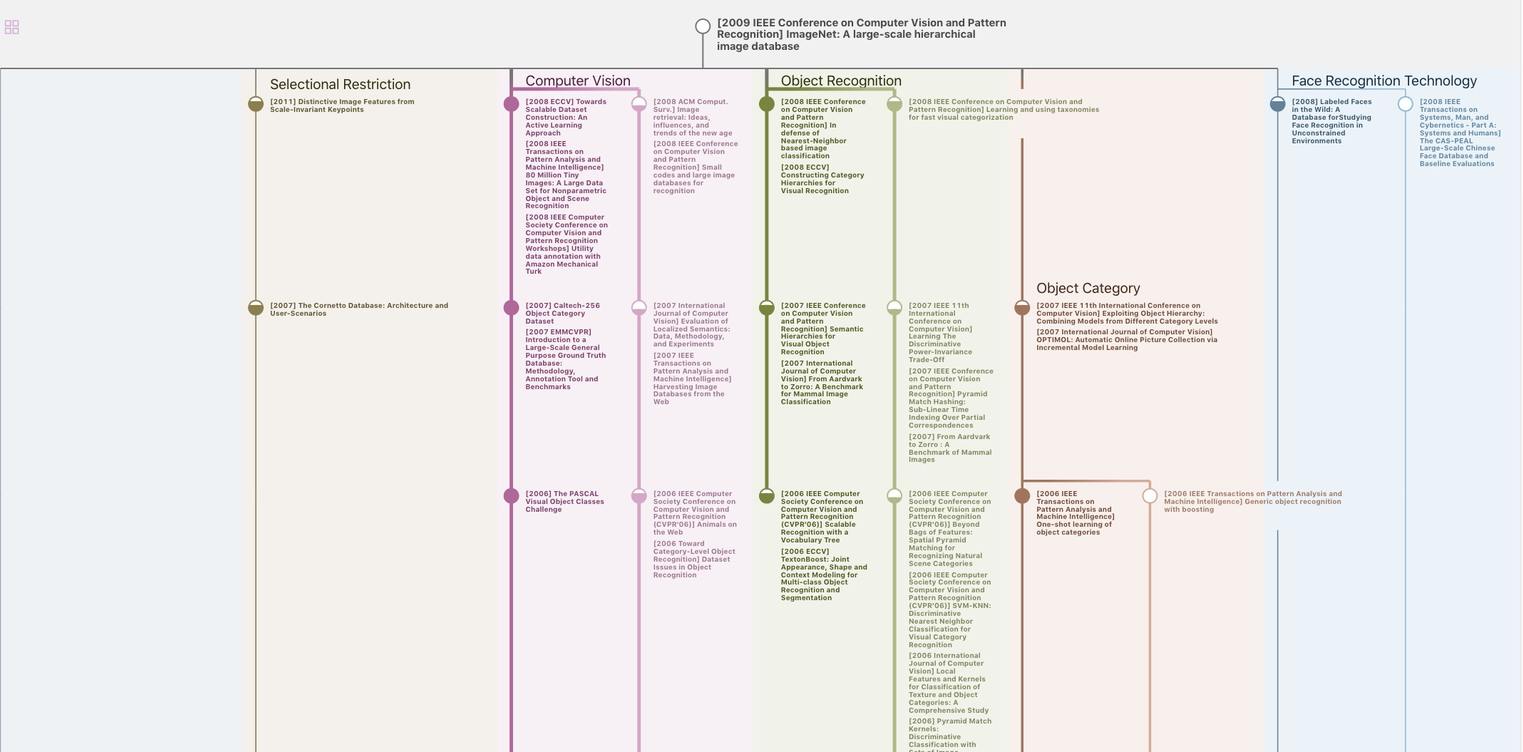
生成溯源树,研究论文发展脉络
Chat Paper
正在生成论文摘要