BSHIFT: A Low Cost Deep Neural Networks Accelerator
International Journal of Parallel Programming(2019)
摘要
Deep neural networks (DNNs) have become ubiquitous in artificial intelligence applications, including image processing, speech processing and natural language processing. However, the main characteristic of DNNs is that they are computationally and memory intensive, making them difficult to deploy on embedded systems with limited hardware resources and power budgets. To address this limitation, we introduce a new quantization method with mixed data structure and bit-shifting broadcast accelerator structure BSHIFT. These works together reduce the storage requirement of neural networks models from 32 to 5 bits without affecting their accuracy. We implement BSHIFT at TSMC 16 nm technology node, and the efficiency achieves 64 TOPS/s per watt in our experiments.
更多查看译文
关键词
Deep neural networks,Low power,Lossless,Accelerator
AI 理解论文
溯源树
样例
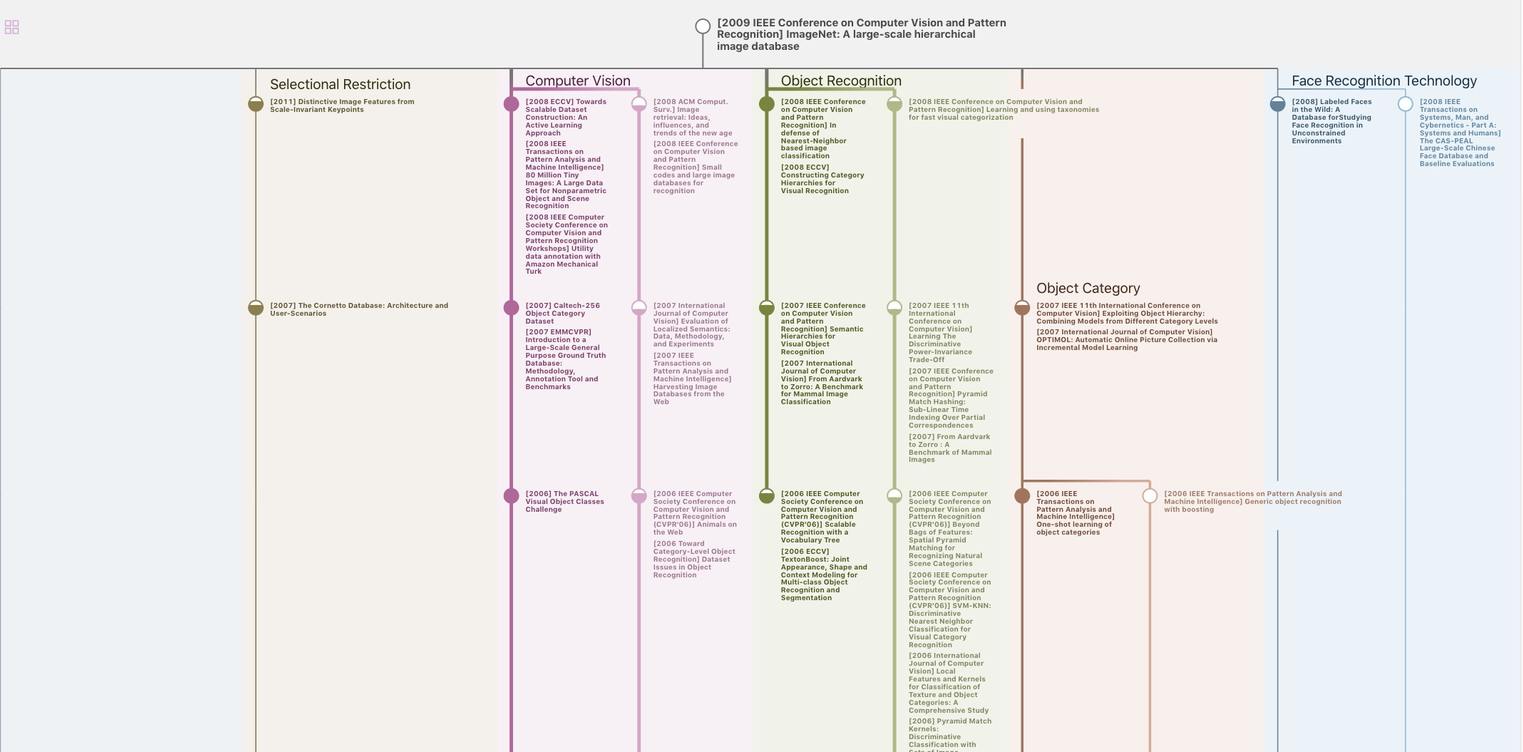
生成溯源树,研究论文发展脉络
Chat Paper
正在生成论文摘要