Pedestrian Detection with Multi-scale Context-Embedded Feature Learning
2017 4th IAPR Asian Conference on Pattern Recognition (ACPR)(2017)
摘要
Large variance in instance scales will results in undesirable large intra-category variance in features, which may severely hurt the performance of modern pedestrian detection (PD) methods. In this paper, we develop a new approach to alleviate scale variety and explore context information for pedestrian detection. Separate detectors are trained with a set of scale-variant templates for different scales pedestrian instances, and thus the features from various layers can be fused in a foveal style to capture both high-resolution detail and coarse low-resolution cues. In the meantime, local context is embedded in a scalevariant manner to enhance the ability for small-size instances detection further. Experiments show that the proposed work achieves state-of-the-art performance on SJTUSPID dataset [20], as well as competitive results on Caltech dataset [5].
更多查看译文
关键词
Pedestrian detection,Scale variety,Feature fusion,Contextual reasoning
AI 理解论文
溯源树
样例
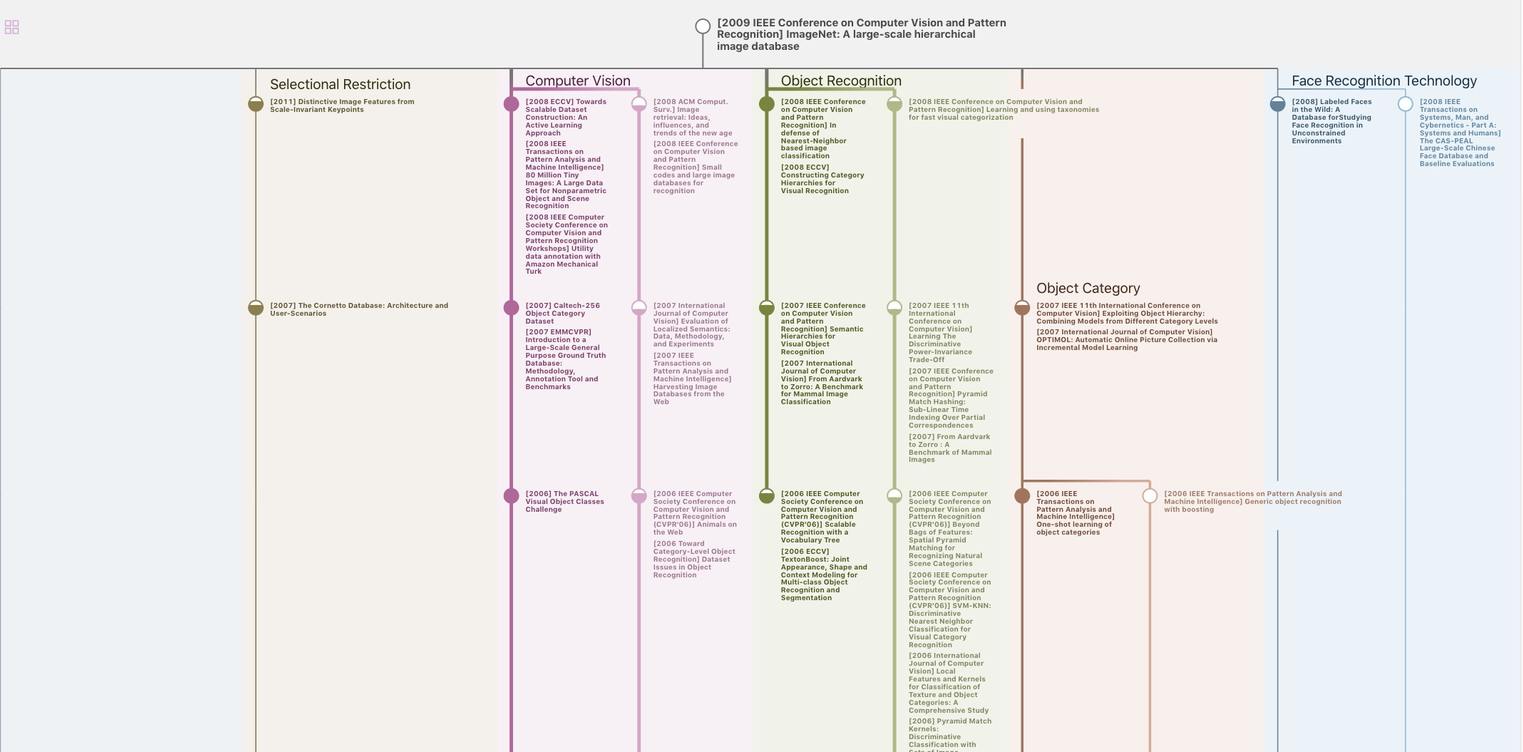
生成溯源树,研究论文发展脉络
Chat Paper
正在生成论文摘要