Marginal Deep Architectures
PROCEEDINGS 2017 4TH IAPR ASIAN CONFERENCE ON PATTERN RECOGNITION (ACPR)(2017)
摘要
Many deep architectures have been proposed in recent years. To obtain good results, most of the previous deep models need a large number of training data. In this paper, for small and middle scale applications, we propose a novel deep learning framework based on stacked feature learning models. Particularly, we stack marginal Fisher analysis (MFA) layer by layer for the initialization of the deep architecture and call it "Marginal Deep Architectures" (MDA). In the implementation of MDA, the weight matrices of MFA are first learned layer by layer, and then some deep learning techniques are employed to fine tune the deep architecture. To evaluate the effectiveness of MDA, we have compared it with related feature learning methods and deep learning models on six small and middle scale real-world applications. Extensive experiments demonstrate that MDA performs not only better than shallow feature learning models, but also deep learning models in these applications.
更多查看译文
关键词
Deep architectures,Feature learning,Marginal Fisher analysis
AI 理解论文
溯源树
样例
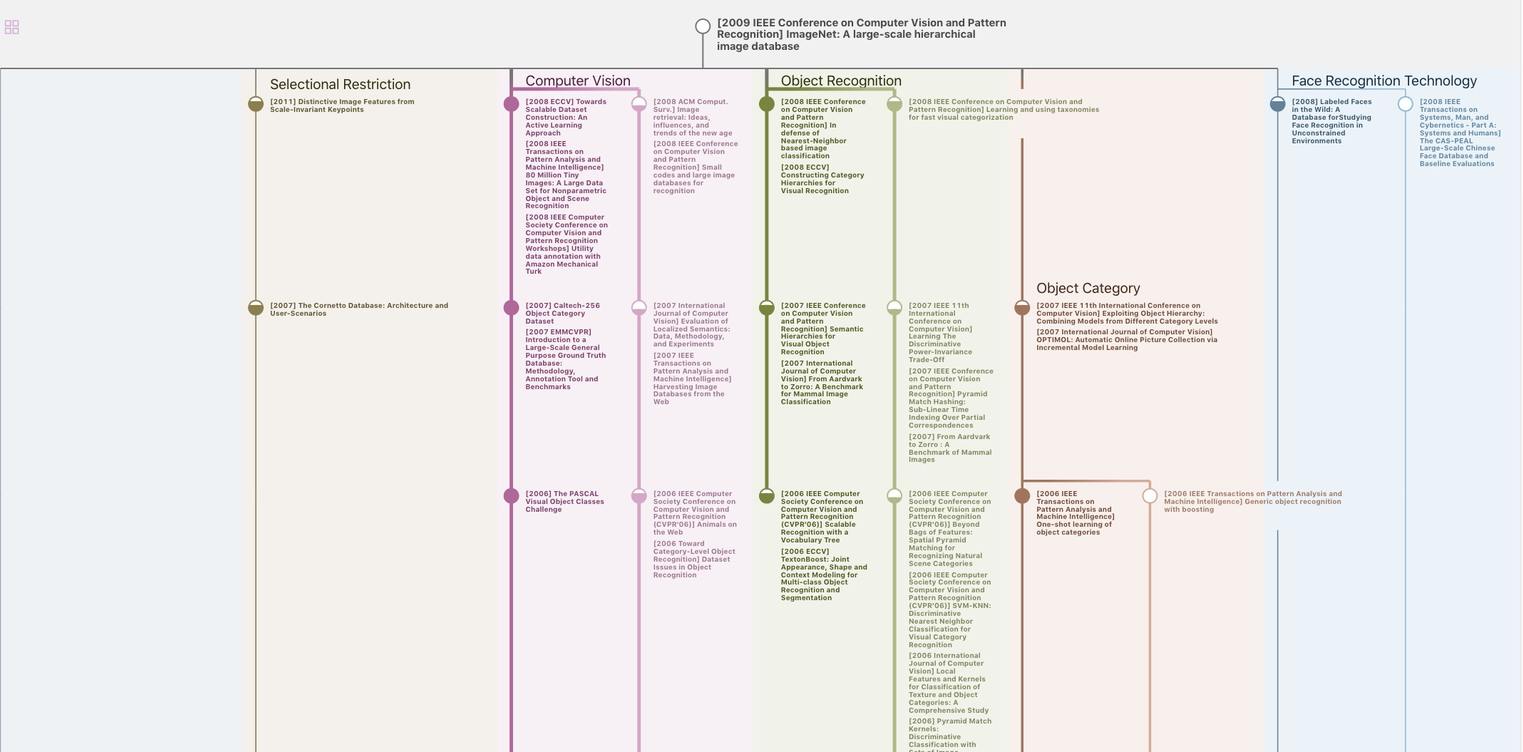
生成溯源树,研究论文发展脉络
Chat Paper
正在生成论文摘要