Bayesian Modeling and Inference for Nonignorably Missing Longitudinal Binary Response Data with Applications to HIV Prevention Trials.
STATISTICA SINICA(2018)
摘要
Missing data are frequently encountered in longitudinal clinical trials. To better monitor and understand the progress over time, one must handle the missing data appropriately and examine whether the missing data mechanism is ignorable or nonignorable. In this article, we develop a new probit model for longitudinal binary response data. It resolves a challenging issue for estimating the variance of the random effects, and substantially improves the convergence and mixing of the Gibbs sampling algorithm. We show that when improper uniform priors are specified for the regression coefficients of the joint multinomial model via a sequence of one-dimensional conditional distributions for the missing data indicators under nonignorable missingness, the joint posterior distribution is improper. A variation of Jeffreys prior is thus established as a remedy for the improper posterior distribution. In addition, an efficient Gibbs sampling algorithm is developed using a collapsing technique. Two model assessment criteria, the deviance information criterion (DIC) and the logarithm of the pseudomarginal likelihood (LPML), are used to guide the choices of prior specifications and to compare the models under different missing data mechanisms. We report on extensive simulations conducted to investigate the empirical performance of the proposed methods. The proposed methodology is further illustrated using data from an HIV prevention clinical trial.
更多查看译文
关键词
Collapsed Gibbs sampler,DIC,identifiability,Jeffreys prior,latent variable,LPML,probit model
AI 理解论文
溯源树
样例
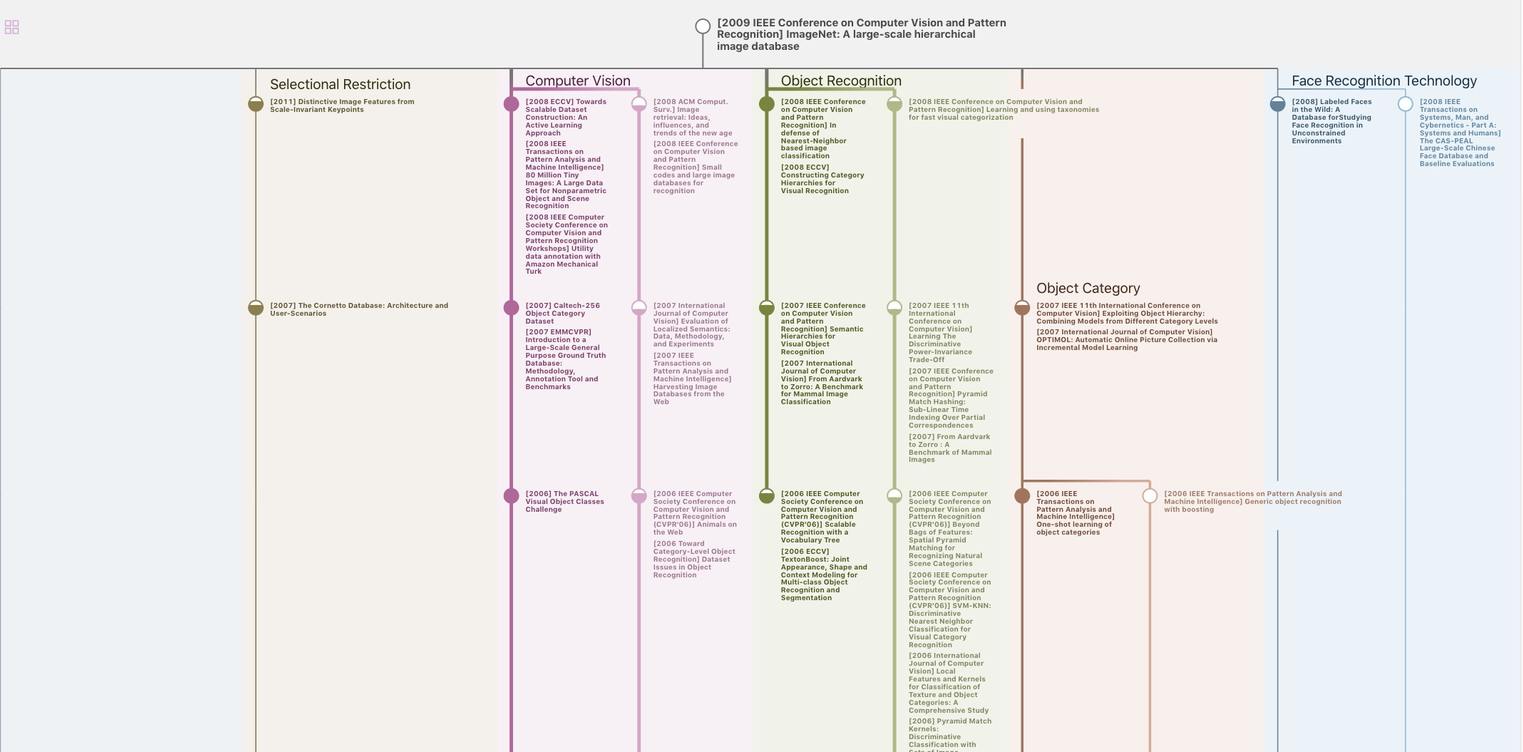
生成溯源树,研究论文发展脉络
Chat Paper
正在生成论文摘要