HC-HDSD: A method of hypergraph construction and high-density subgraph detection for inferring high-order epistatic interactions
Computational Biology and Chemistry(2019)
摘要
Detecting epistatic interactions, or nonlinear interactive effects of Single Nucleotide Polymorphisms (SNPs), has gained increasing attention in explaining the “missing heritability” of complex diseases. Though much work has been done in mapping SNPs underlying diseases, most of them constrain to 2-order epistatic interactions. In this paper, a method of hypergraph construction and high-density subgraph detection, named HC-HDSD, is proposed for detecting high-order epistatic interactions. The hypergraph is constructed by low-order epistatic interactions that identified using the normalized co-information measure and the exhaustive search. The hypergraph consists of two types of vertices: real ones representing main effects of SNPs and virtual ones denoting interactive effects of epistatic interactions. Then, both maximal clique centrality algorithm and near-clique mining algorithm are employed to detect high-density subgraphs from the constructed hypergraph. These high-density subgraphs are inferred as high-order epistatic interactions in the HC-HDSD. Experiments are performed on several simulation data sets, results of which show that HC-HDSD is promising in inferring high-order epistatic interactions while substantially reducing the computation cost. In addition, the application of HC-HDSD on a real Age-related Macular Degeneration (AMD) data set provides several new clues for the exploration of causative factors of AMD.
更多查看译文
关键词
Epistatic interactions,Single nucleotide polymorphisms (SNPs),Co-information,Hypergraph,High-density subgraph
AI 理解论文
溯源树
样例
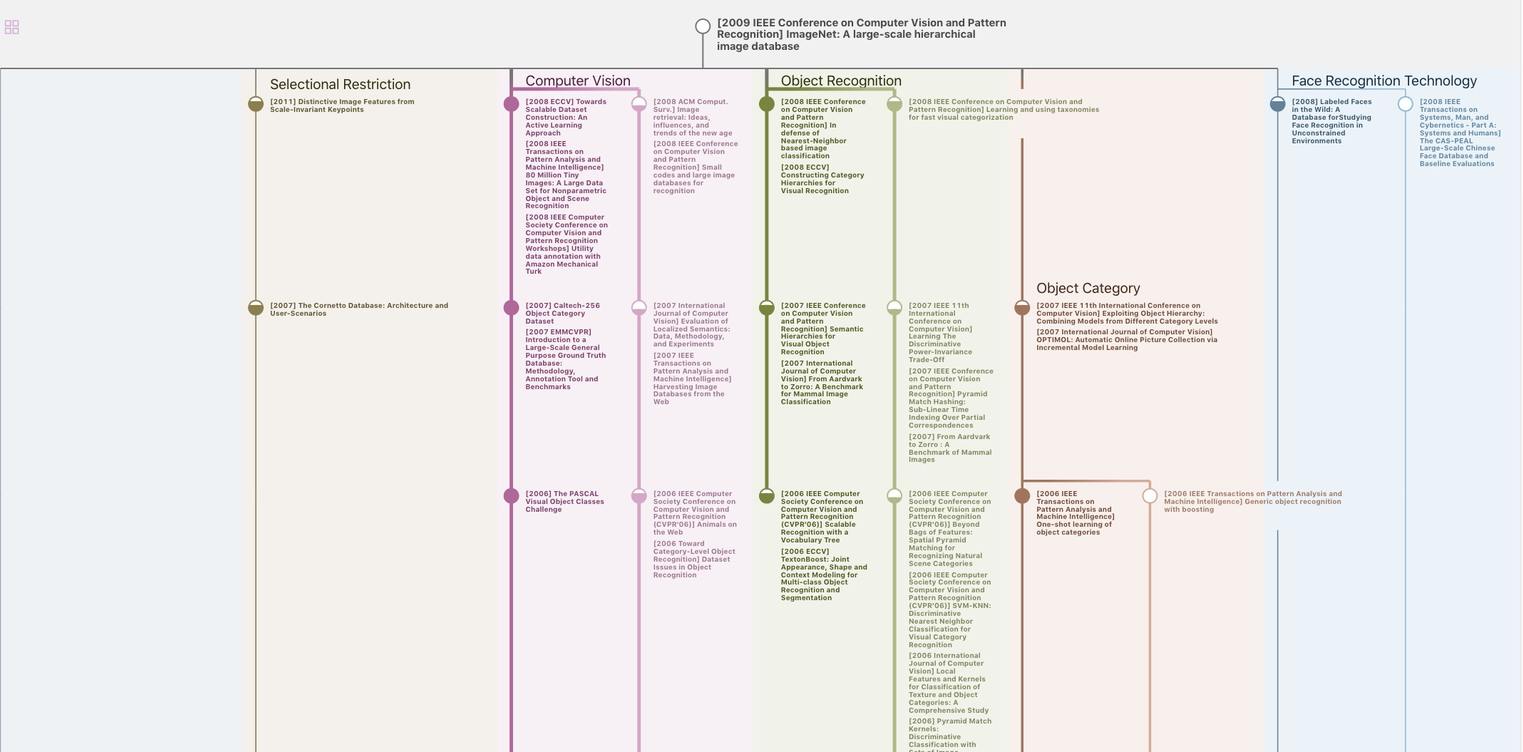
生成溯源树,研究论文发展脉络
Chat Paper
正在生成论文摘要