Weka Trainable Segmentation Plugin in ImageJ: A Semi-Automatic Tool Applied to Crystal Size Distributions of Microlites in Volcanic Rocks.
MICROSCOPY AND MICROANALYSIS(2018)
摘要
Crystals within volcanic rocks record geochemical and textural signatures during magmatic evolution before eruption. Clues to this magmatic history can be examined using crystal size distribution (CSD) studies. The analysis of CSDs is a standard petrological tool, but laborious due to manual hand-drawing of crystal margins. The trainable Weka segmentation (TWS) plugin in ImageJ is a promising alternative. It uses machine learning and image segmentation to classify an image. We recorded back-scattered electron (BSE) images of three volcanic samples with different crystallinity (35, 50 and >= 85 vol. %), using scanning electron microscopes (SEM) of variable image resolutions, which we then tested using TWS. Crystal measurements obtained from the automatically segmented images are compared with those of the manual segmentation. Samples up to 50 vol. % crystallinity are successfully segmented using TWS. Segmentation at significantly higher crystallinities fails, as crystal boundaries cannot be distinguished. Accuracy performance tests for the TWS classifiers yield high F-scores (>0.930), hence, TWS is a successful and fast computing tool for outlining crystals from BSE images of glassy rocks. Finally, reliable CSD's can be derived using a low-cost desktop SEM, paving the way for a wide range of research to take advantage of this new petrological method.
更多查看译文
关键词
crystal size distributions,microlites,electron microprobe,image segmentation,automation
AI 理解论文
溯源树
样例
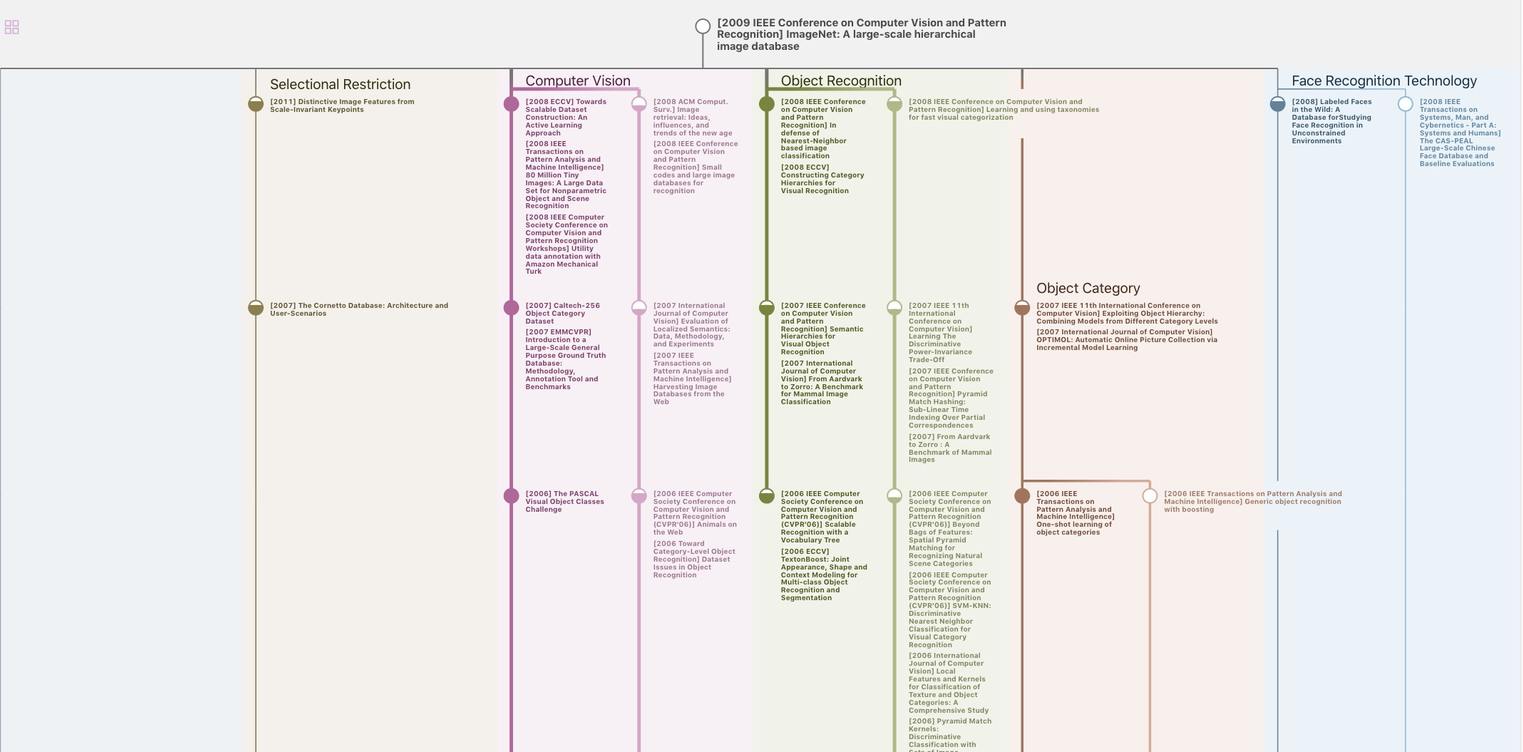
生成溯源树,研究论文发展脉络
Chat Paper
正在生成论文摘要