Hybrid Matrix Factorization Update for Progress Modeling in Intelligent Tutoring Systems.
Communications in Computer and Information Science(2017)
摘要
Intelligent Tutoring Systems often profit of intelligent components, which allow to personalize the proposed contents' characteristics and sequence. Adaptive sequencing, in particular, requires either a detrimental data collection for users or extensive domain information provided by experts of the educational area. In this paper we propose an efficient domain independent method to model student progress that can be later used to sequence tasks in large commercial systems. The developed method is based on the integration of domain independent Matrix Factorization Performance Prediction with Kalman Filters state modeling abilities. Our solution not only reduces the prediction error, but also possesses a more computationally efficient model update. Finally, we give hints about a potential interpretability of student's state computed by Matrix Factorization, that, because of its implicit modeling, did not allow human experts, to monitor user's knowledge acquisition.
更多查看译文
关键词
Progress modeling,Kalman filerts,Matrix factorization,Performance prediction,Sequencing
AI 理解论文
溯源树
样例
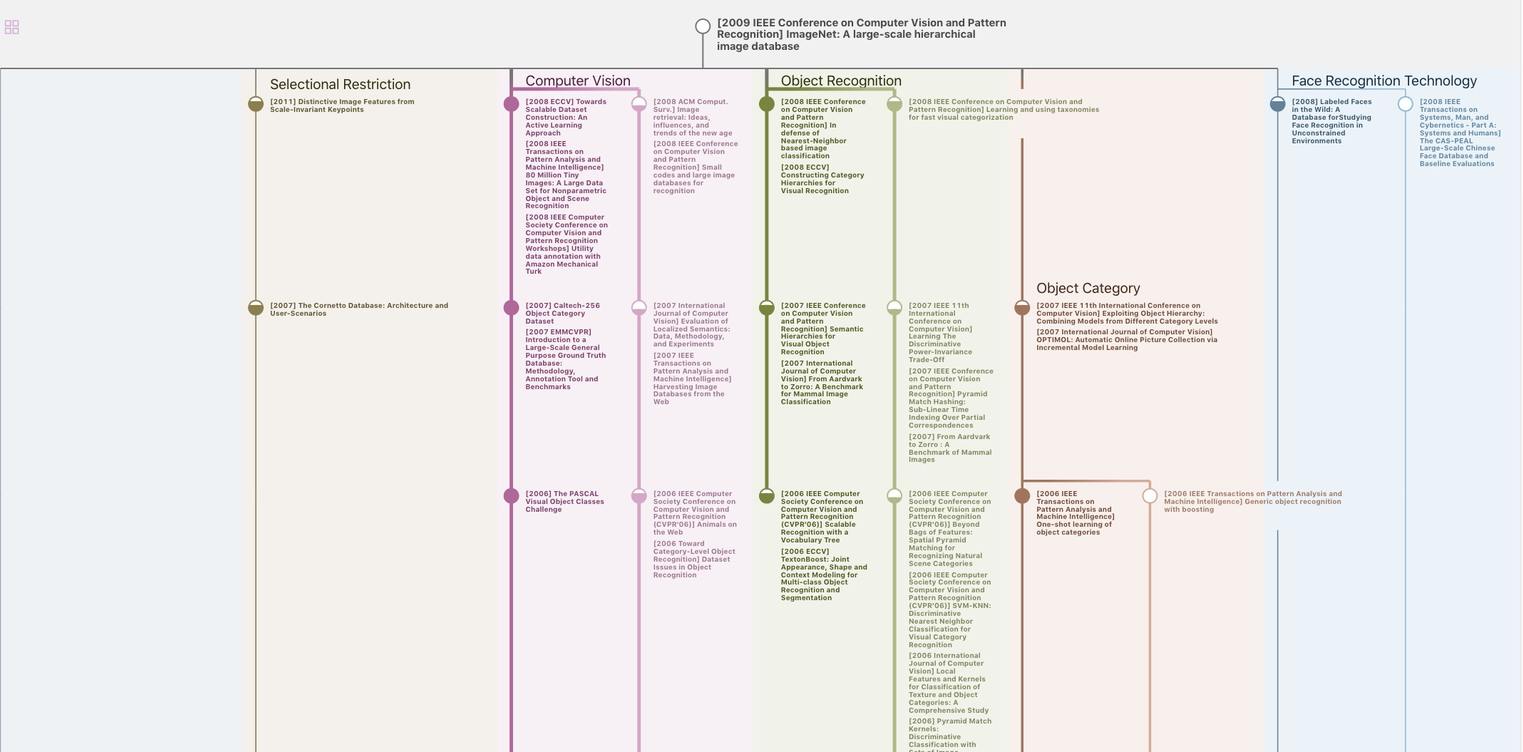
生成溯源树,研究论文发展脉络
Chat Paper
正在生成论文摘要