Multi-Scale Generative Adversarial Networks For Crowd Counting
2018 24TH INTERNATIONAL CONFERENCE ON PATTERN RECOGNITION (ICPR)(2018)
摘要
We investigate generative adversarial networks as an effective solution to the crowd counting problem. These networks not only learn the mapping from crowd image to corresponding density map, but also learn a loss function to train this mapping. There are many challenges to the task of crowd counting, such as severe occlusions in extremely dense crowd scenes, perspective distortion, and high visual similarity between pedestrians and background elements. To address these problems, we proposed multi-scale generative adversarial network to generate high-quality crowd density maps of arbitrary crowd density scenes. We utilized the adversarial loss from discriminator to improve the quality of the estimated density map, which is critical to accurately predict crowd counts. The proposed multi-scale generator can extract multiple hierarchy features from the crowd image. The results showed that the proposed method provided better performance compared to current state-of-the-art methods
更多查看译文
关键词
multiscale generative adversarial network,multiple hierarchy features,multiscale generator,adversarial loss,arbitrary crowd density scenes,high-quality crowd density maps,extremely dense crowd scenes,crowd image,crowd counting problem
AI 理解论文
溯源树
样例
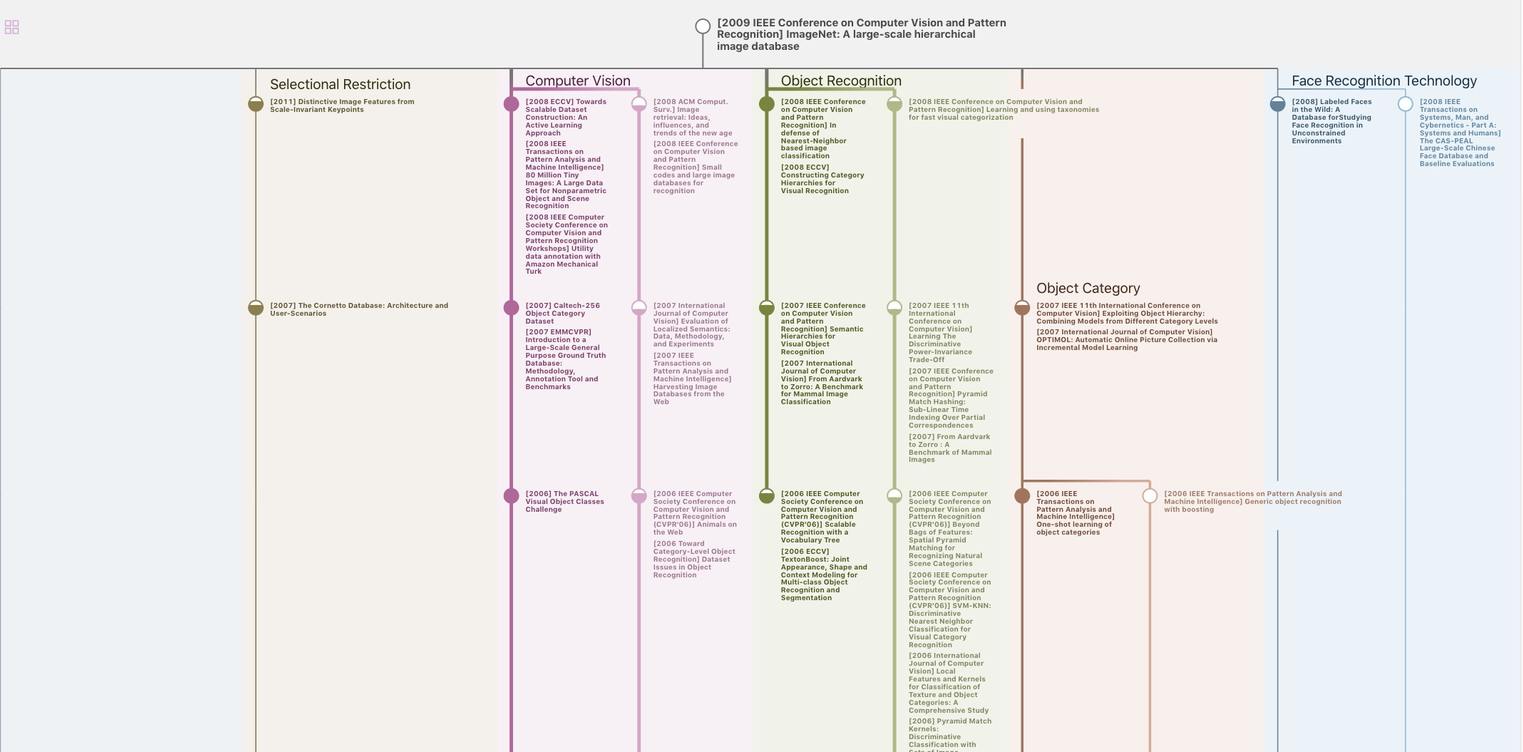
生成溯源树,研究论文发展脉络
Chat Paper
正在生成论文摘要