Person Re-identification Based on Feature Fusion and Triplet Loss Function
2018 24TH INTERNATIONAL CONFERENCE ON PATTERN RECOGNITION (ICPR)(2018)
摘要
The task of Person re-identification (re-ID) is to recognize an individual observed by non-overlapping cameras. Robust feature representation is a crucial problem in re-ID. With the rise of deep learning, most current approaches adopt convolutional neural networks (CNN) to extract features. However, the feature representation learned by CNN is often global and lacks detailed local information. To address this issue, this paper proposes a simple CNN architecture consisting of a re-ID subnetwork and an attribute sub-network. In re-ID sub-network, global feature and semantic feature are extracted and fused in a weighted manner, and triplet loss is adopted to further improve the discriminative ability of the learned fusion feature. On the other hand, attribute sub-network focuses on local aspects of a person and offers local structural information that is helpful for re-ID. The two sub-networks are combined on the loss level and their complementary aspects are leveraged to improve the re-ID accuracy. Comparative evaluations demonstrate that our method outperforms several state-of-the-art ones. On the challenging Market1501 and DukeMTMC datasets, 86.3% rank-1 accuracy and 69.4% mAP, and 72.1% rank-1 accuracy and 53.4% mAP are achieved respectively.
更多查看译文
关键词
robust feature representation,deep learning,convolutional neural networks,detailed local information,simple CNN architecture,re-ID subnetwork,attribute sub-network,global feature,semantic feature,triplet loss,learned fusion feature,local aspects,local structural information,loss level,re-ID accuracy,feature fusion,loss function,nonoverlapping cameras
AI 理解论文
溯源树
样例
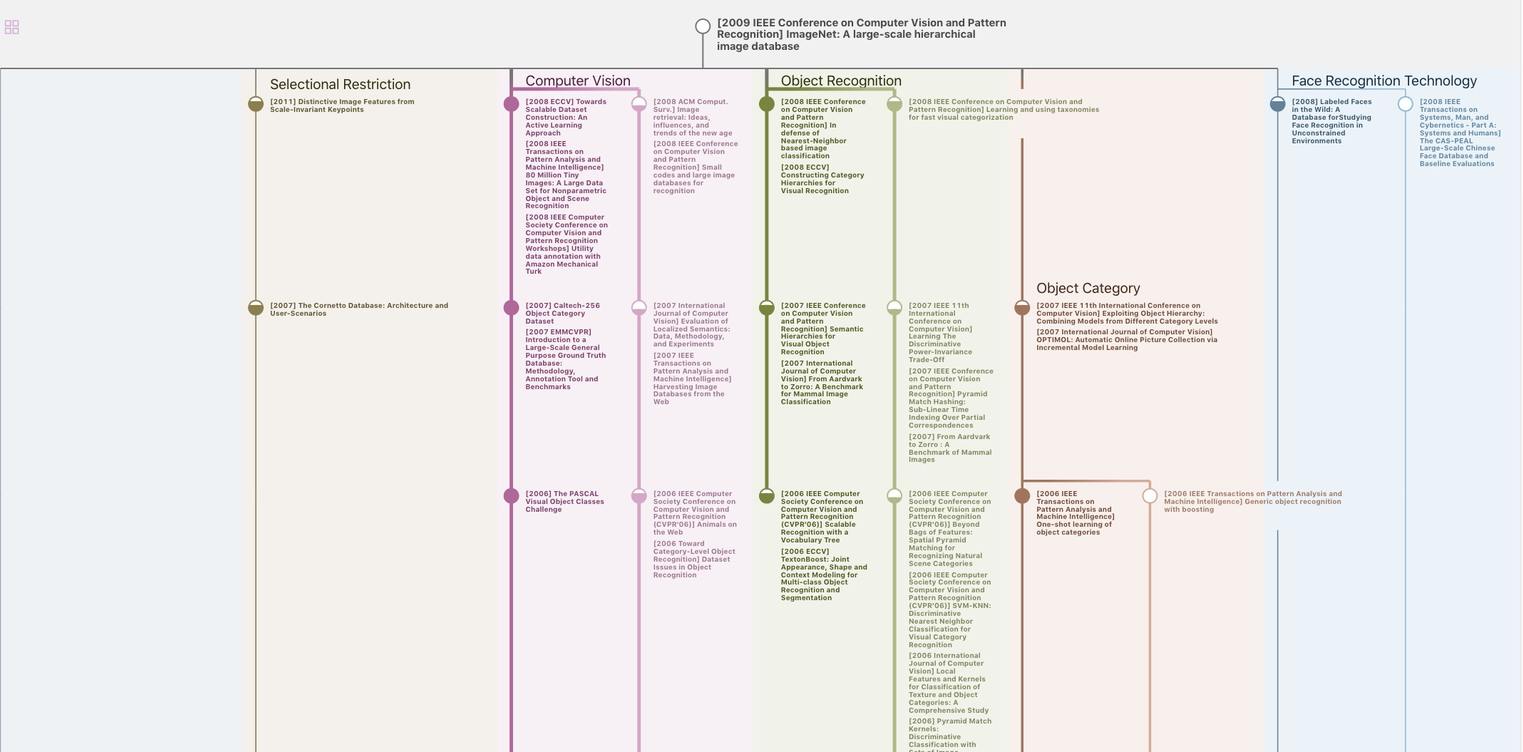
生成溯源树,研究论文发展脉络
Chat Paper
正在生成论文摘要