Multimodal Gesture Recognition Using Densely Connected Convolution And Blstm
2018 24TH INTERNATIONAL CONFERENCE ON PATTERN RECOGNITION (ICPR)(2018)
摘要
In this paper, we present a multimodal method based on densely connected convolution and bidirectional long-short-term-memory (BLSTM) for gesture recognition. The proposed method learns spatial features of gestures through the densely connected convolutional network, and then learns long-term temporal features by BLSTM network. In addition, fusion methods are evaluated on our model, and we find that fusion of features with different information can significantly improve the recognition accuracy. This purely data driven approach achieves state-of-the-art recognition accuracy on the ChaLearn LAP 2014 dataset and the Sheffield Kinect gesture (SKIG) dataset. (98.80% on the ChaLearn LAP and 99.07% on SKIG)
更多查看译文
关键词
bidirectional long-short-term-memory,densely connected convolutional network,multimodal gesture recognition,Sheffield Kinect gesture dataset,fusion methods,BLSTM network,long-term temporal features
AI 理解论文
溯源树
样例
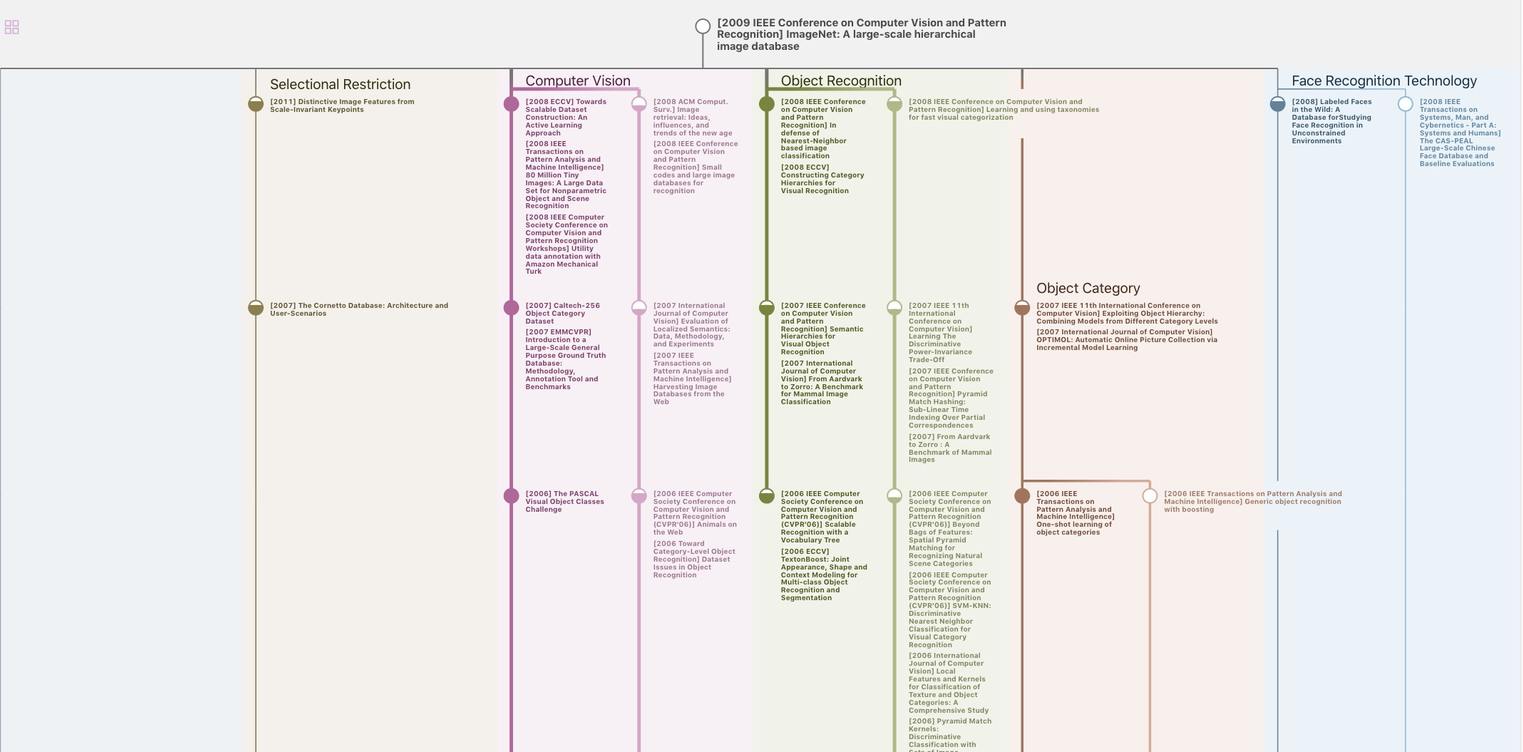
生成溯源树,研究论文发展脉络
Chat Paper
正在生成论文摘要